Designing a Bayesian Regularization Approach to Solve the Fractional Layla and Majnun System
MATHEMATICS(2023)
摘要
The present work provides the numerical solutions of the mathematical model based on the fractional-order Layla and Majnun model (MFLMM). A soft computing stochastic-based Bayesian regularization neural network approach (BRNNA) is provided to investigate the numerical accomplishments of the MFLMM. The nonlinear system is classified into two dynamics, whereas the correctness of the BRNNA is observed through the comparison of results. Furthermore, the reducible performance of the absolute error improves the exactitude of the computational BRNNA. Twenty neurons have been chosen, along with the data statics of training 74% and 13%, for both authorization and testing. The consistency of the designed BRNNA is demonstrated using the correlation/regression, error histograms, and transition of state values in order to solve the MFLMM.
更多查看译文
关键词
Layla and Majnun, fractional, neural networks, Bayesian regularization approach, numerical solutions
AI 理解论文
溯源树
样例
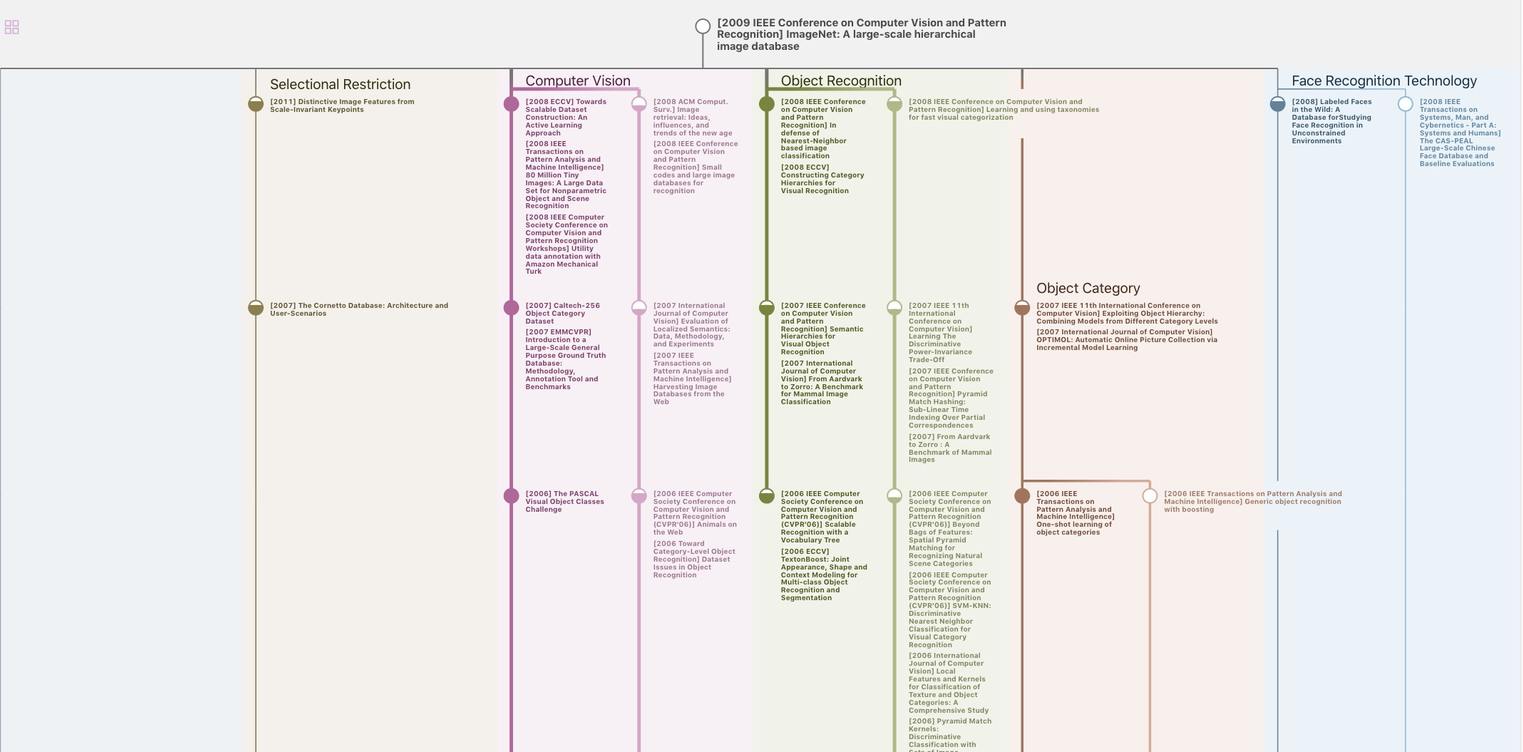
生成溯源树,研究论文发展脉络
Chat Paper
正在生成论文摘要