API-MalDetect: Automated malware detection framework for windows based on API calls and deep learning techniques
JOURNAL OF NETWORK AND COMPUTER APPLICATIONS(2023)
摘要
This paper presents API-MalDetect, a new deep learning-based automated framework for detecting malware attacks in Windows systems. The framework uses an NLP-based encoder for API calls and a hybrid automatic feature extractor based on convolutional neural networks (CNNs) and bidirectional gated recurrent units (BiGRU) to extract features from raw and long sequences of API calls. The proposed framework is designed to detect unseen malware attacks and prevent performance degradation over time or across different rates of exposure to malware by reducing temporal bias and spatial bias during training and testing. Experimental results show that API-MalDetect outperforms existing state-of-the-art malware detection techniques in terms of accuracy, precision, recall, F1-score, and AUC-ROC on different benchmark datasets of API call sequences. These results demonstrate that the ability to automatically identify unique and highly relevant patterns from raw and long sequences of API calls is effective in distinguishing malware attacks from benign activities in Windows systems using the proposed API-MalDetect framework. API-MalDetect is also able to show cybersecurity experts which API calls were most important in malware identification. Furthermore, we make our dataset available to the research community.
更多查看译文
关键词
00-01,99-00
AI 理解论文
溯源树
样例
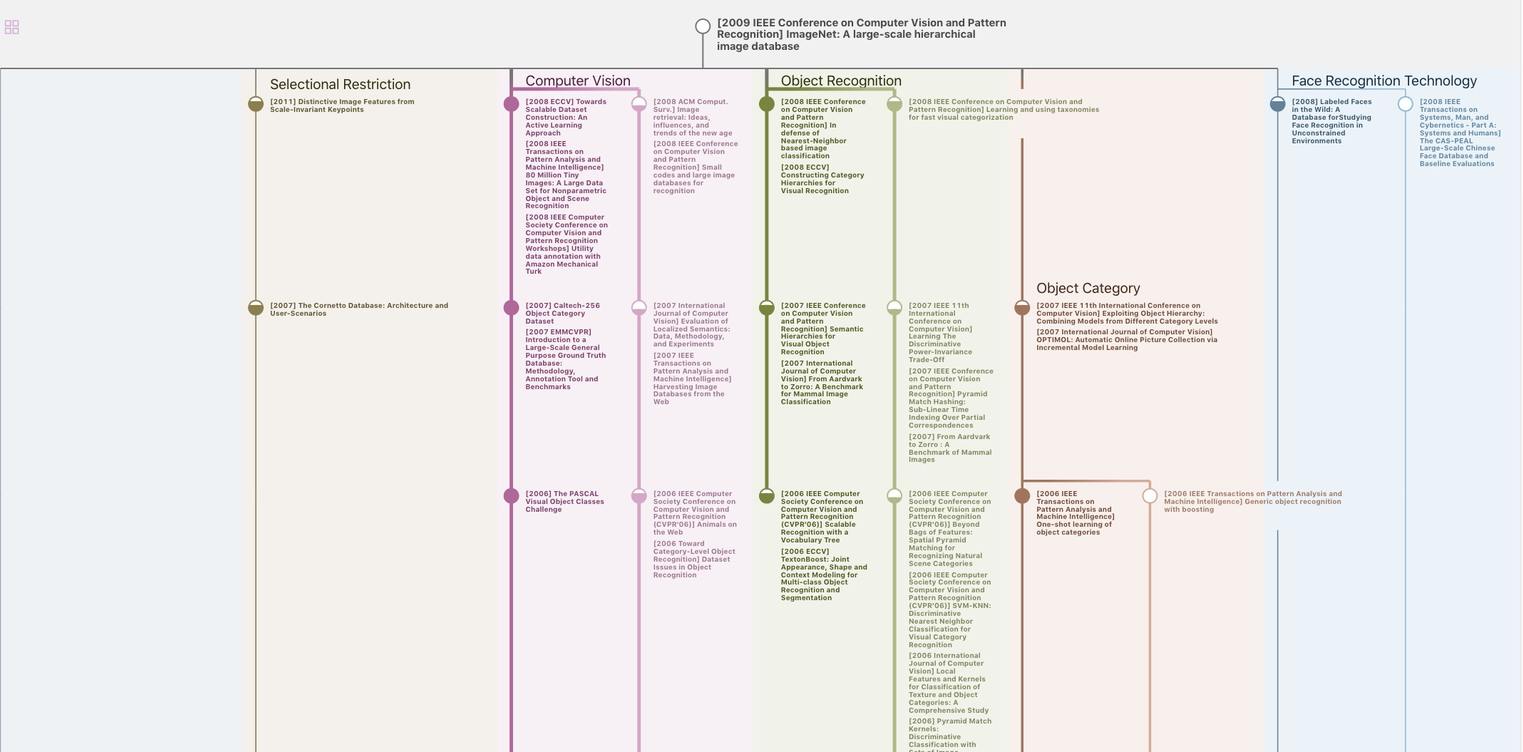
生成溯源树,研究论文发展脉络
Chat Paper
正在生成论文摘要