Datasets of Simulated Exhaled Aerosol Images from Normal and Diseased Lungs with Multi-Level Similarities for Neural Network Training/Testing and Continuous Learning
DATA(2023)
摘要
Although exhaled aerosols and their patterns may seem chaotic in appearance, they inherently contain information related to the underlying respiratory physiology and anatomy. This study presented a multi-level database of simulated exhaled aerosol images from both normal and diseased lungs. An anatomically accurate mouth-lung geometry extending to G9 was modified to model two stages of obstructions in small airways and physiology-based simulations were utilized to capture the fluid-particle dynamics and exhaled aerosol images from varying breath tests. The dataset was designed to test two performance metrics of convolutional neural network (CNN) models when used for transfer learning: interpolation and extrapolation. To this aim, three testing datasets with decreasing image similarities were developed (i.e., level 1, inbox, and outbox). Four network models (AlexNet, ResNet-50, MobileNet, and EfficientNet) were tested and the performances of all models decreased for the outbox test images, which were outside the design space. The effect of continuous learning was also assessed for each model by adding new images into the training dataset and the newly trained network was tested at multiple levels. Among the four network models, ResNet-50 excelled in performance in both multi-level testing and continuous learning, the latter of which enhanced the accuracy of the most challenging classification task (i.e., 3-class with outbox test images) from 60.65% to 98.92%. The datasets can serve as a benchmark training/testing database for validating existent CNN models or quantifying the performance metrics of new CNN models.Dataset: The dataset is submitted and will be published as a supplement to this paper in the journal Data, this field will be filled by the editors of the journal. CFD Lung Diagnosis|Kaggle.Dataset License: license under (CC0, CC-BY, CC-BY-SA, CC-BYaeor-NC, etc.)
更多查看译文
关键词
simulated exhaled aerosol images,lungs,neural network training/testing,continuous learning,neural network,multi-level
AI 理解论文
溯源树
样例
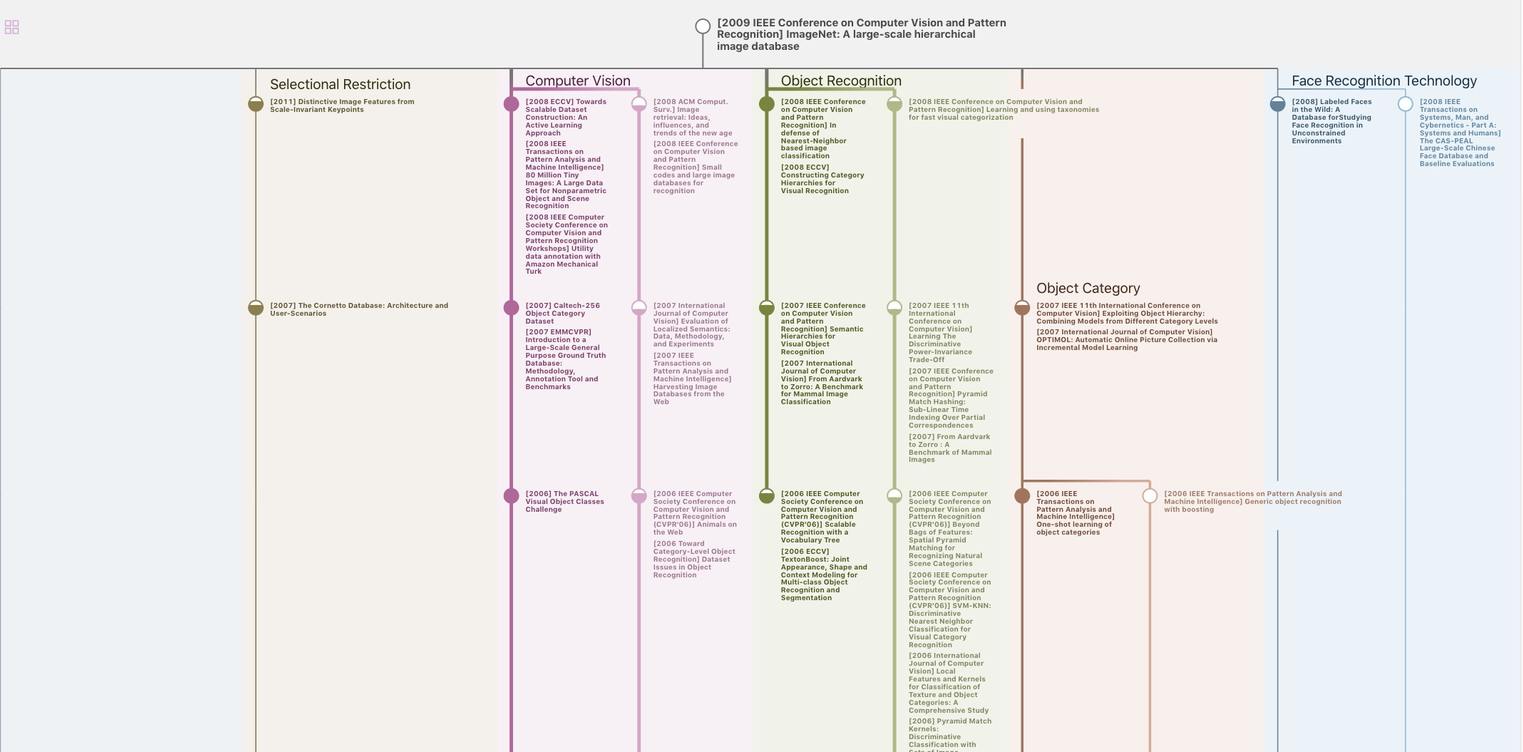
生成溯源树,研究论文发展脉络
Chat Paper
正在生成论文摘要