Image semantic segmentation algorithm based on a multi-expert system
JOURNAL OF ELECTRONIC IMAGING(2023)
摘要
Deeplab series semantic segmentation algorithms extract target semantic features using deep layers of a convolutional neural network, resulting in target features lacking detailed information, such as edges and shapes extracted by shallow layers. Deeplabv3plus uses atrous convolution to obtain feature maps, which lose some image information. All of the above have an impact on segmentation performance improvement. In response to these issues, which reduce segmentation performance, we propose an image semantic segmentation algorithm based on a multi-expert system that builds multiple expert models based on the Deeplabv3plus network architecture. For the target image, each expert model makes independent judgments, and the segmentation results are obtained through the ensemble learning of these expert models. Expert model 1 employs the proposed attention-based atrous spatial pyramid pooling (C-ASPP) module to capture richer global semantic information via a parallel attention mechanism and ASSP module. Expert model 2 designs a feature fusion-based decoder that uses a feature fusion approach to obtain detailed information. Expert model 3 introduces a loss function in the Deeplabv3plus network for supervised detailed information loss. The final segmentation results are generated by adjudicating the results derived by the different expert models, which improves the segmentation performance by compensating for the loss of detailed information and enhancing the semantic features. Evaluated on the commonly used semantic segmentation datasets PASCAL VOC 2012 and CamVid, the algorithm's mIoU reached 82.42% and 69.18%, respectively, which were 2.46% and 1.82% higher than Deeplabv3plus, proving the better segmentation performance of the algorithm. (C) 2023 SPIE and IS&T
更多查看译文
关键词
semantic segmentation,Deeplab,multi-expert system,ensemble learning
AI 理解论文
溯源树
样例
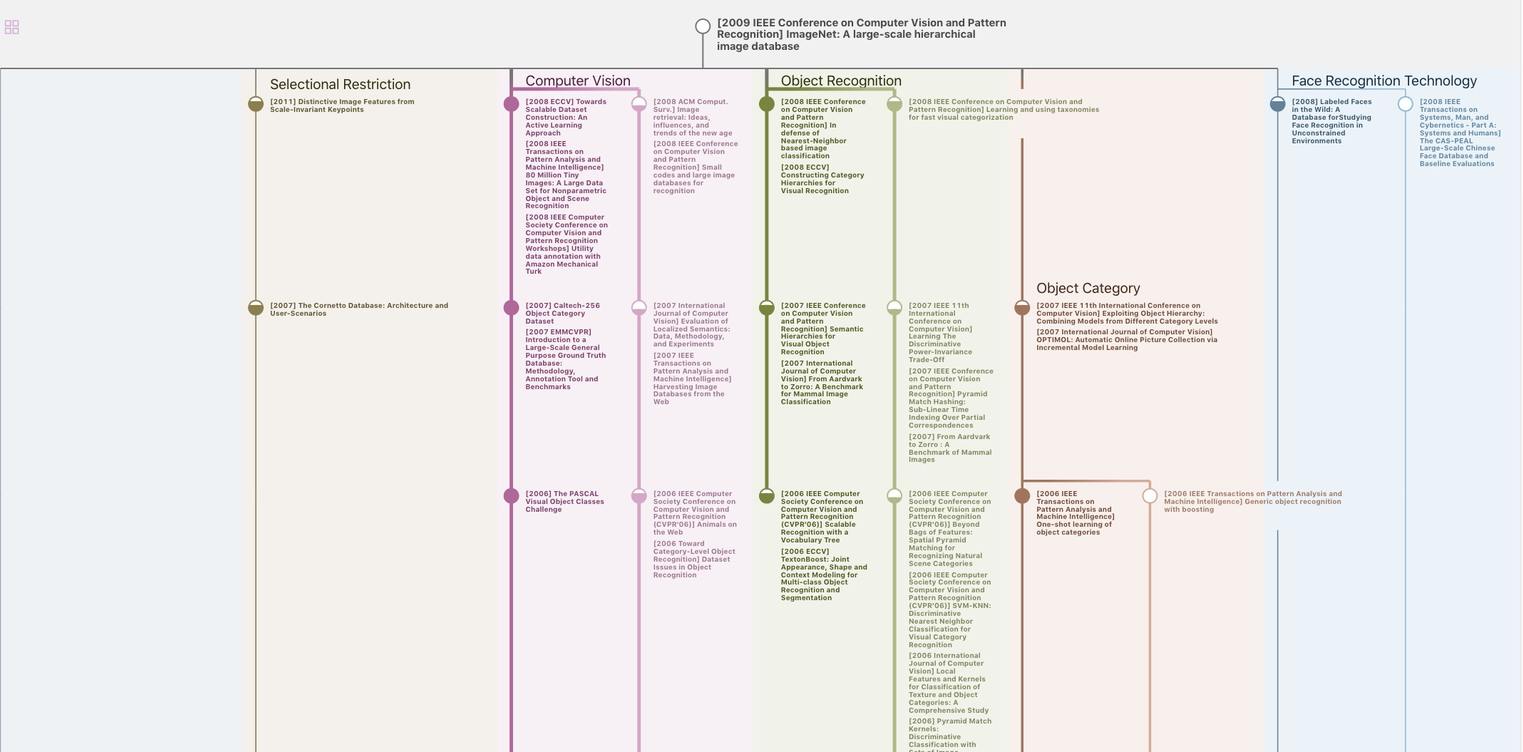
生成溯源树,研究论文发展脉络
Chat Paper
正在生成论文摘要