Ensemble machine learning framework for daylight modelling of various building layouts
BUILDING SIMULATION(2023)
摘要
The application of machine learning (ML) modelling in daylight prediction has been a promising approach for reliable and effective visual comfort assessment. Although many advancements have been made, no standardized ML modelling framework exists in daylight assessment. In this study, 625 different building layouts were generated to model useful daylight illuminance (UDI). Two state-of-the-art ML algorithms, eXtreme Gradient Boosting (XGBoost) and random forest (RF), were employed to analyze UDI in four categories: UDI-f (fell short), UDI-s (supplementary), UDI-a (autonomous), and UDI-e (exceeded). A feature (internal finish) was introduced to the framework to better reflect real-world representation. The results show that XGBoost models predict UDI with a maximum accuracy of R2 = 0.992. Compared to RF, the XGBoost ML models can significantly reduce prediction errors. Future research directions have been specified to advance the proposed framework by introducing new features and exploring new ML architectures to standardize ML applications in daylight prediction.
更多查看译文
关键词
artificial intelligence,indoor environment,machine learning,parametric building layout,sunlight,visual comfort
AI 理解论文
溯源树
样例
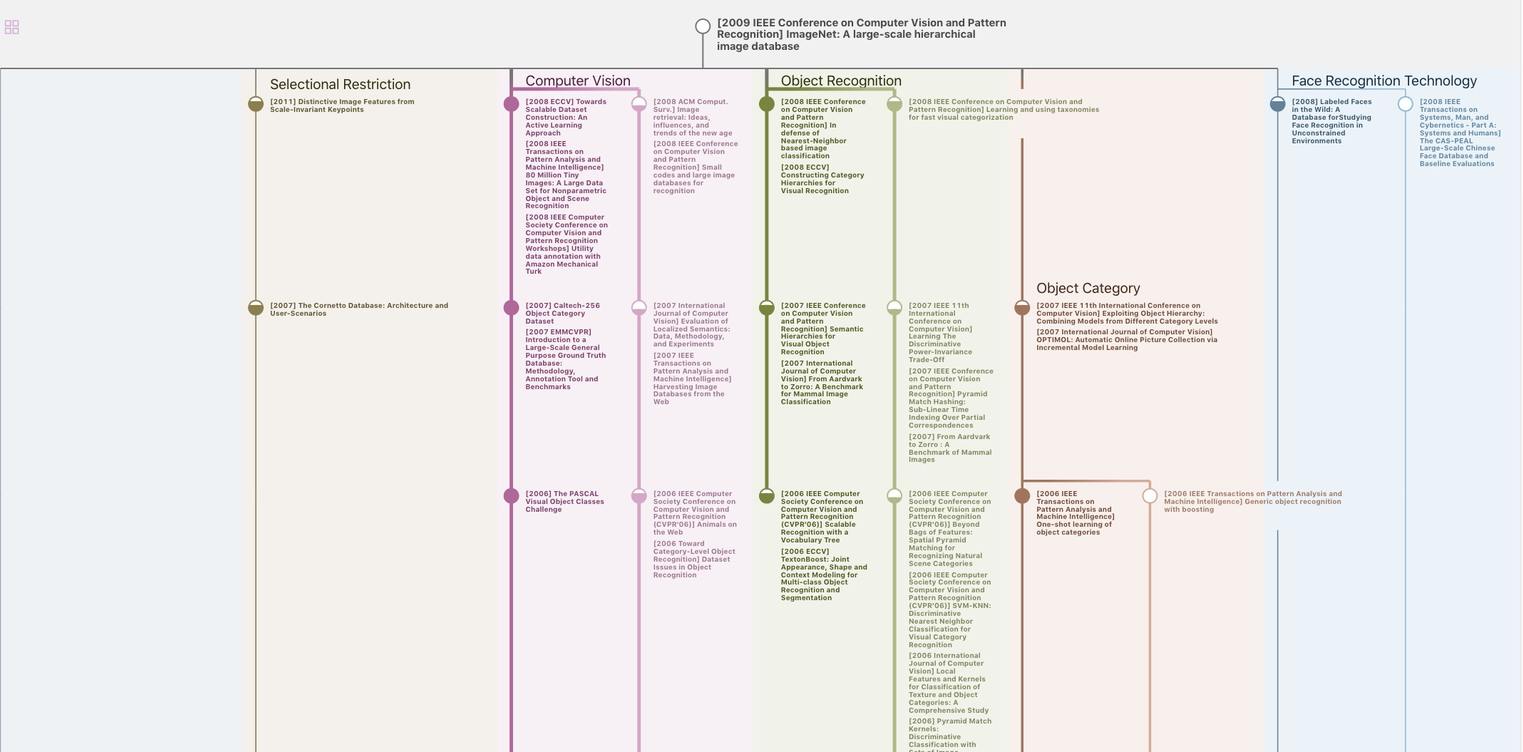
生成溯源树,研究论文发展脉络
Chat Paper
正在生成论文摘要