Feature-wise attention based boosting ensemble method for fraud detection
ENGINEERING APPLICATIONS OF ARTIFICIAL INTELLIGENCE(2023)
摘要
Transaction fraud detection is an essential topic in financial research, protecting customers and financial institutions from suffering significant financial losses. The existing ensemble learning-based fraud detection model improves the accuracy of distinguishing fraudulent transactions by integrating multiple base learners. However, their insufficient optimization of variance leads to the inadequate generalization of the model, which makes them unable to meet the requirements of detecting evolving fraudulent behavior. So, this paper proposes an attention-based boosting ensemble model (AM-Boost) for fraud detection that imparts diversity perturbation to the base learner from the feature dimension. Firstly, the feature-wise attention mechanism is proposed to help the model exploit the highly informative fraud features by learning distributed global feature space. And it is combined with boosting strategy to reduce the variance by lowering the correlation between base learners. Secondly, we propose a feature diversity regularization term in the integration stage to optimize the weighted majority voting strategy with a reasonable trade-off between bias and variance. Finally, we evaluate the proposed method on a public fraud detection dataset and a real-world transaction dataset. Extensive experiment results demonstrate that our method outperforms the baseline methods in both datasets.
更多查看译文
关键词
Fraud detection,Attention mechanism,Ensemble learning,Boosting
AI 理解论文
溯源树
样例
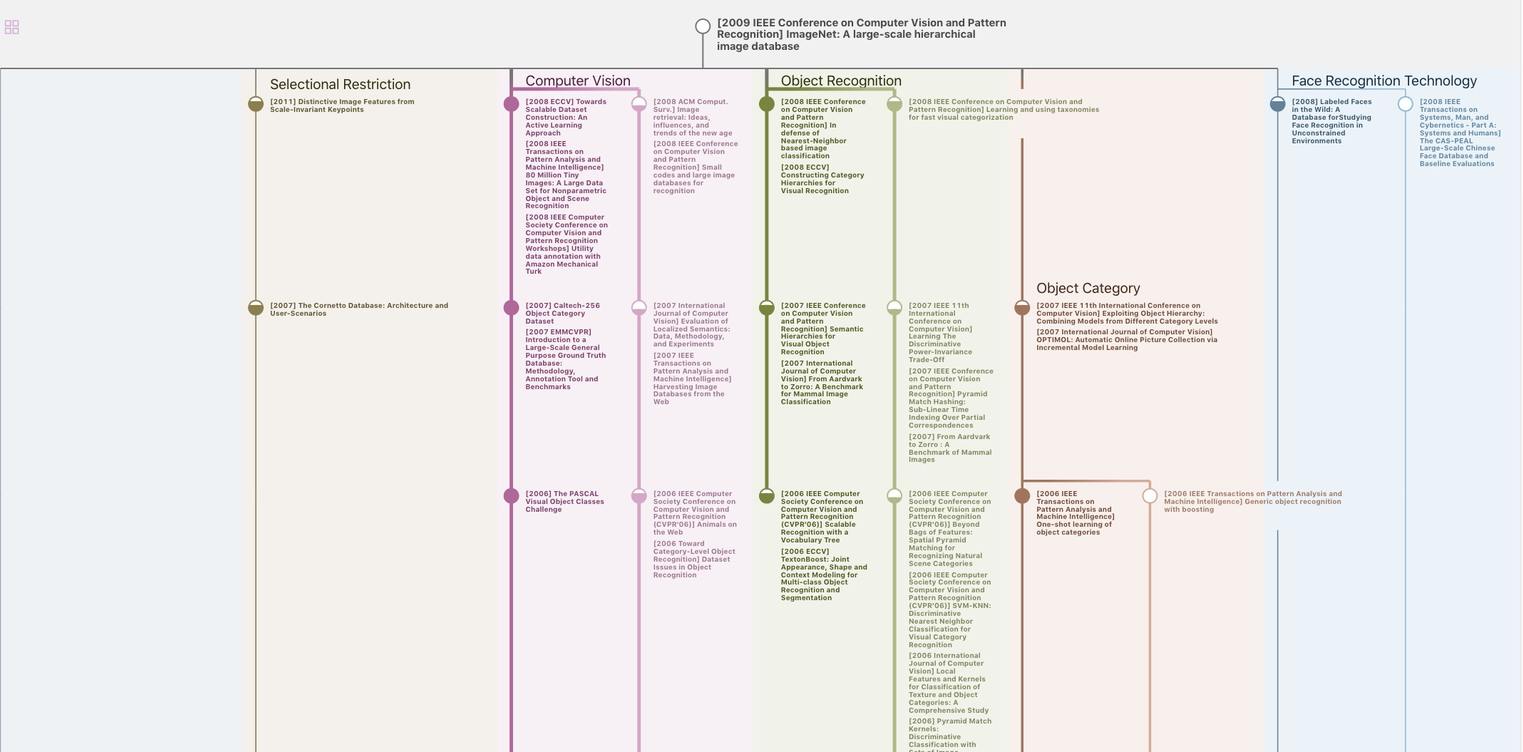
生成溯源树,研究论文发展脉络
Chat Paper
正在生成论文摘要