Real-time liver motion estimation via deep learning-based angle-agnostic X-ray imaging
MEDICAL PHYSICS(2023)
摘要
BackgroundReal-time liver imaging is challenged by the short imaging time (within hundreds of milliseconds) to meet the temporal constraint posted by rapid patient breathing, resulting in extreme under-sampling for desired 3D imaging. Deep learning (DL)-based real-time imaging/motion estimation techniques are emerging as promising solutions, which can use a single X-ray projection to estimate 3D moving liver volumes by solved deformable motion. However, such techniques were mostly developed for a specific, fixed X-ray projection angle, thereby impractical to verify and guide arc-based radiotherapy with continuous gantry rotation.PurposeTo enable deformable motion estimation and 3D liver imaging from individual X-ray projections acquired at arbitrary X-ray scan angles, and to further improve the accuracy of single X-ray-driven motion estimation.MethodsWe developed a DL-based method, X360, to estimate the deformable motion of the liver boundary using an X-ray projection acquired at an arbitrary gantry angle (angle-agnostic). X360 incorporated patient-specific prior information from planning 4D-CTs to address the under-sampling issue, and adopted a deformation-driven approach to deform a prior liver surface mesh to new meshes that reflect real-time motion. The liver mesh motion is solved via motion-related image features encoded in the arbitrary-angle X-ray projection, and through a sequential combination of rigid and deformable registration modules. To achieve the angle agnosticism, a geometry-informed X-ray feature pooling layer was developed to allow X360 to extract angle-dependent image features for motion estimation. As a liver boundary motion solver, X360 was also combined with priorly-developed, DL-based optical surface imaging and biomechanical modeling techniques for intra-liver motion estimation and tumor localization.ResultsWith geometry-aware feature pooling, X360 can solve the liver boundary motion from an arbitrary-angle X-ray projection. Evaluated on a set of 10 liver patient cases, the mean (& PLUSMN; s.d.) 95-percentile Hausdorff distance between the solved liver boundary and the "ground-truth" decreased from 10.9 (& PLUSMN;4.5) mm (before motion estimation) to 5.5 (& PLUSMN;1.9) mm (X360). When X360 was further integrated with surface imaging and biomechanical modeling for liver tumor localization, the mean (& PLUSMN; s.d.) center-of-mass localization error of the liver tumors decreased from 9.4 (& PLUSMN; 5.1) mm to 2.2 (& PLUSMN; 1.7) mm.ConclusionX360 can achieve fast and robust liver boundary motion estimation from arbitrary-angle X-ray projections for real-time imaging guidance. Serving as a surface motion solver, X360 can be integrated into a combined framework to achieve accurate, real-time, and marker-less liver tumor localization.
更多查看译文
关键词
graph neural network,liver,real-time imaging,X-ray
AI 理解论文
溯源树
样例
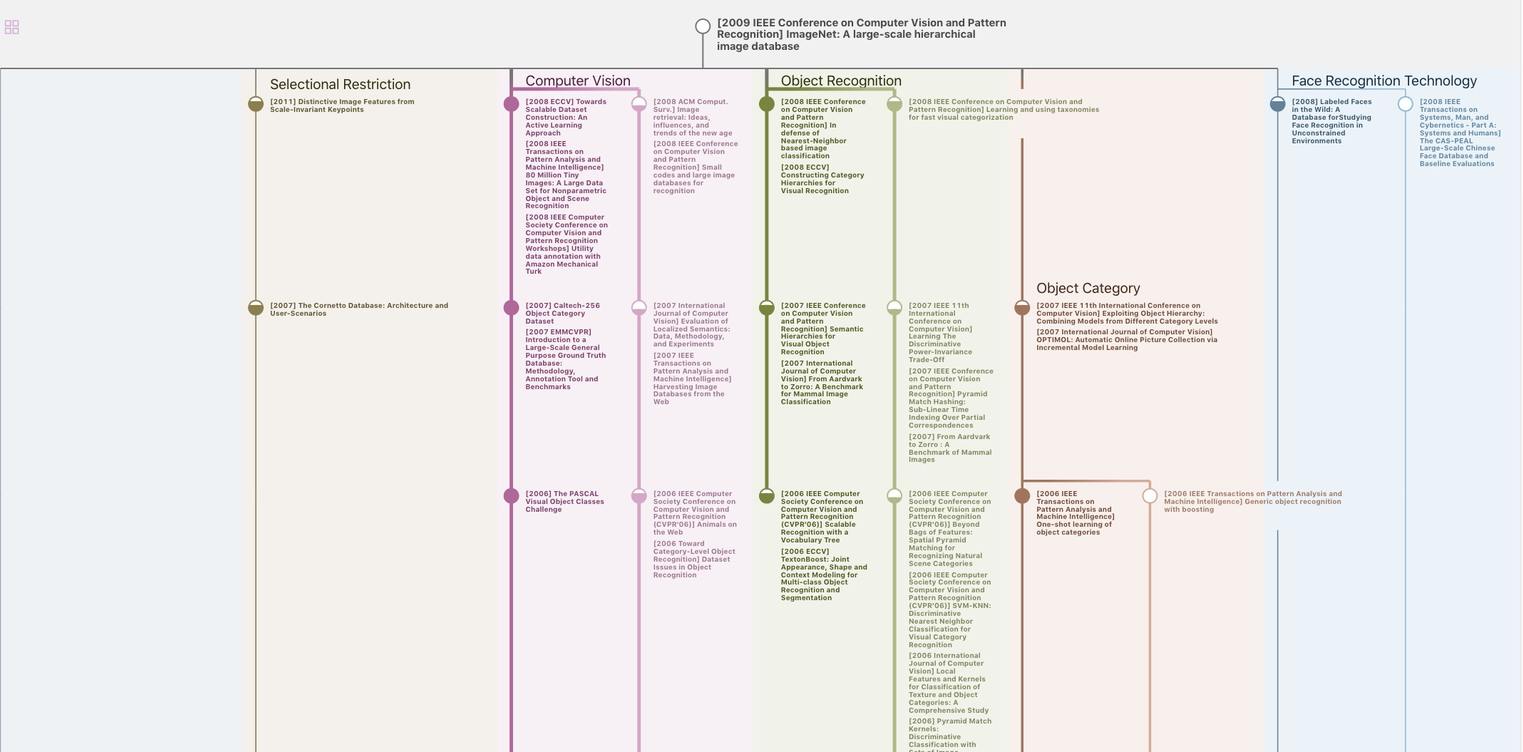
生成溯源树,研究论文发展脉络
Chat Paper
正在生成论文摘要