Exactly Uncorrelated Sparse Principal Component Analysis
JOURNAL OF COMPUTATIONAL AND GRAPHICAL STATISTICS(2024)
摘要
Sparse principal component analysis (PCA) aims to find principal components as linear combinations of a subset of the original input variables without sacrificing the fidelity of the classical PCA. Most existing sparse PCA methods produce correlated sparse principal components. We argue that many applications of PCA prefer uncorrelated principal components. However, handling sparsity and uncorrelatedness properties in a sparse PCA method is nontrivial. This article proposes an exactly uncorrelated sparse PCA method named EUSPCA, whose formulation is motivated by original views and motivations of PCA as advocated by Pearson and Hotelling. EUSPCA is a non-smooth constrained non-convex manifold optimization problem. We solve it by combining augmented Lagrangian and non-monotone proximal gradient methods. We observe that EUSPCA produces uncorrelated components and maintains a similar or better level of fidelity based on adjusted total variance through simulated and real data examples. In contrast, existing sparse PCA methods produce significantly correlated components. Supplemental materials for this article are available online.
更多查看译文
关键词
Augmented Lagrangian method,Manifold optimization,Non-monotone proximal gradient method,Principal component analysis,Sparse principal component,Uncorrelated component
AI 理解论文
溯源树
样例
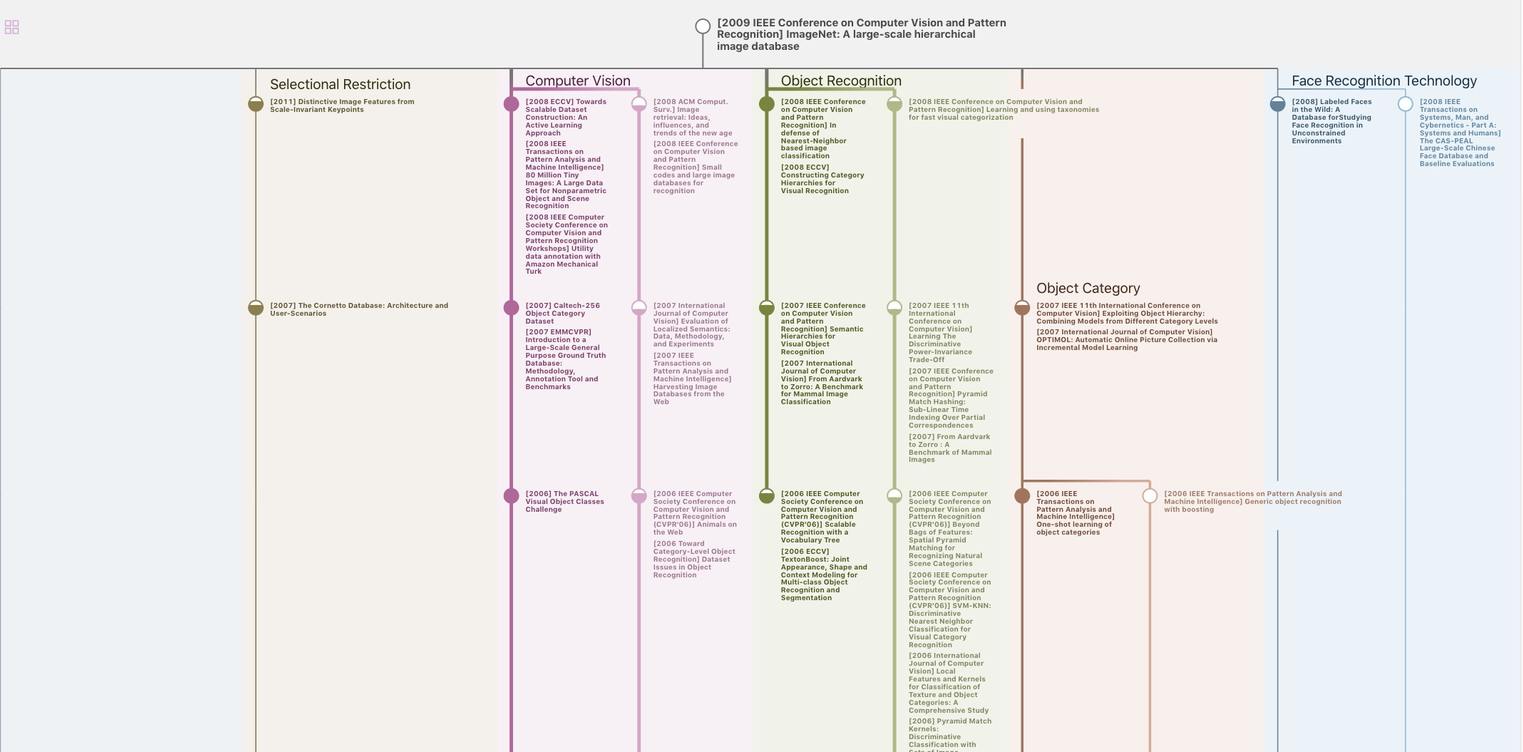
生成溯源树,研究论文发展脉络
Chat Paper
正在生成论文摘要