Episodes and Topics in Multivariate Temporal Data
COMPUTER GRAPHICS FORUM(2023)
摘要
The term 'episode' refers to a time interval in the development of a dynamic process or behaviour of an entity. Episode-based data consist of a set of episodes that are described using time series of multiple attribute values. Our research problem involves analysing episode-based data in order to understand the distribution of multi-attribute dynamic characteristics across a set of episodes. To solve this problem, we applied an existing theoretical model and developed a general approach that involves incrementally increasing data abstraction. We instantiated this general approach in an analysis procedure in which the value variation of each attribute within an episode is represented by a combination of symbols treated as a 'word'. The variation of multiple attributes is thus represented by a combination of 'words' treated as a 'text'. In this way, the the set of episodes is transformed to a collection of text documents. Topic modelling techniques applied to this collection find groups of related (i.e. repeatedly co-occurring) 'words', which are called 'topics'. Given that the 'words' encode variation patterns of individual attributes, the 'topics' represent patterns of joint variation of multiple attributes. In the following steps, analysts interpret the topics and examine their distribution across all episodes using interactive visualizations. We test the effectiveness of the procedure by applying it to two types of episode-based data with distinct properties and introduce a range of generic and data type-specific visualization techniques that can support the interpretation and exploration of topic distribution.
更多查看译文
关键词
multivariate temporal data,topics
AI 理解论文
溯源树
样例
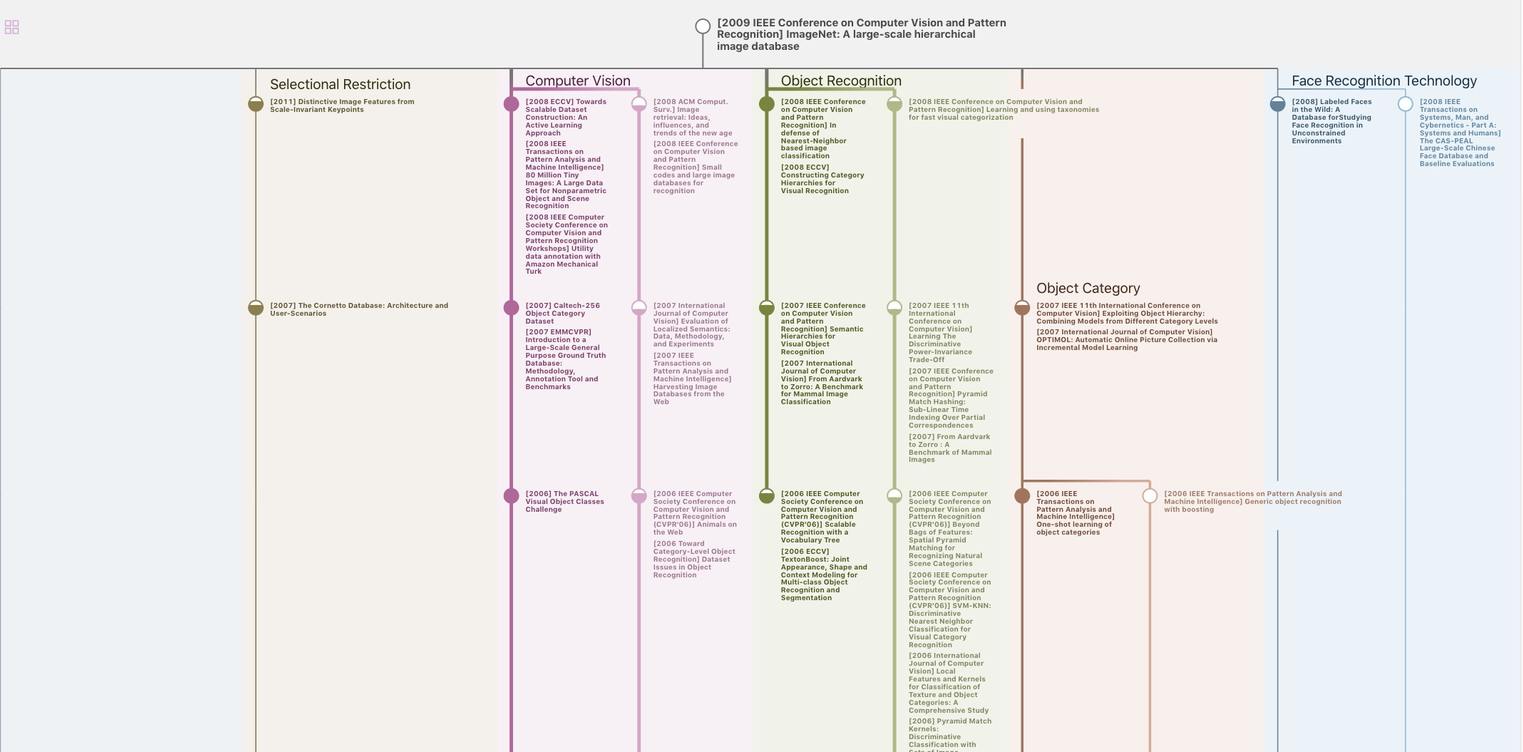
生成溯源树,研究论文发展脉络
Chat Paper
正在生成论文摘要