KE-X: Towards subgraph explanations of knowledge graph embedding based on knowledge information gain
KNOWLEDGE-BASED SYSTEMS(2023)
摘要
Over the past years, knowledge graph embedding approaches have proven effective for knowledge graph completion tasks. However, most existing models are either built on a certain embedding space or black-box neural networks, making it hard to access explanations for prediction results and resulting in limited explainability. In this paper, we propose to leverage information entropy to quantify the importance of explanation candidates, then build a framework KE-X, for explaining results from knowledge graph embedding approaches by generating explainable subgraphs. Specifically, by performing a modified message passing mechanism on a partially masked knowledge subgraph and maximizing knowledge information gain, KE-X can extract the most valuable subgraph explanation for a link prediction query. To evaluate KE-X, we conduct experiments on three real-world knowledge graphs with two representative KGE models, TransE and DistMult. Both quantitative and case study results show that our framework can extract high-quality explanations.
更多查看译文
关键词
Knowledge graph,Knowledge graph embedding,Explainability
AI 理解论文
溯源树
样例
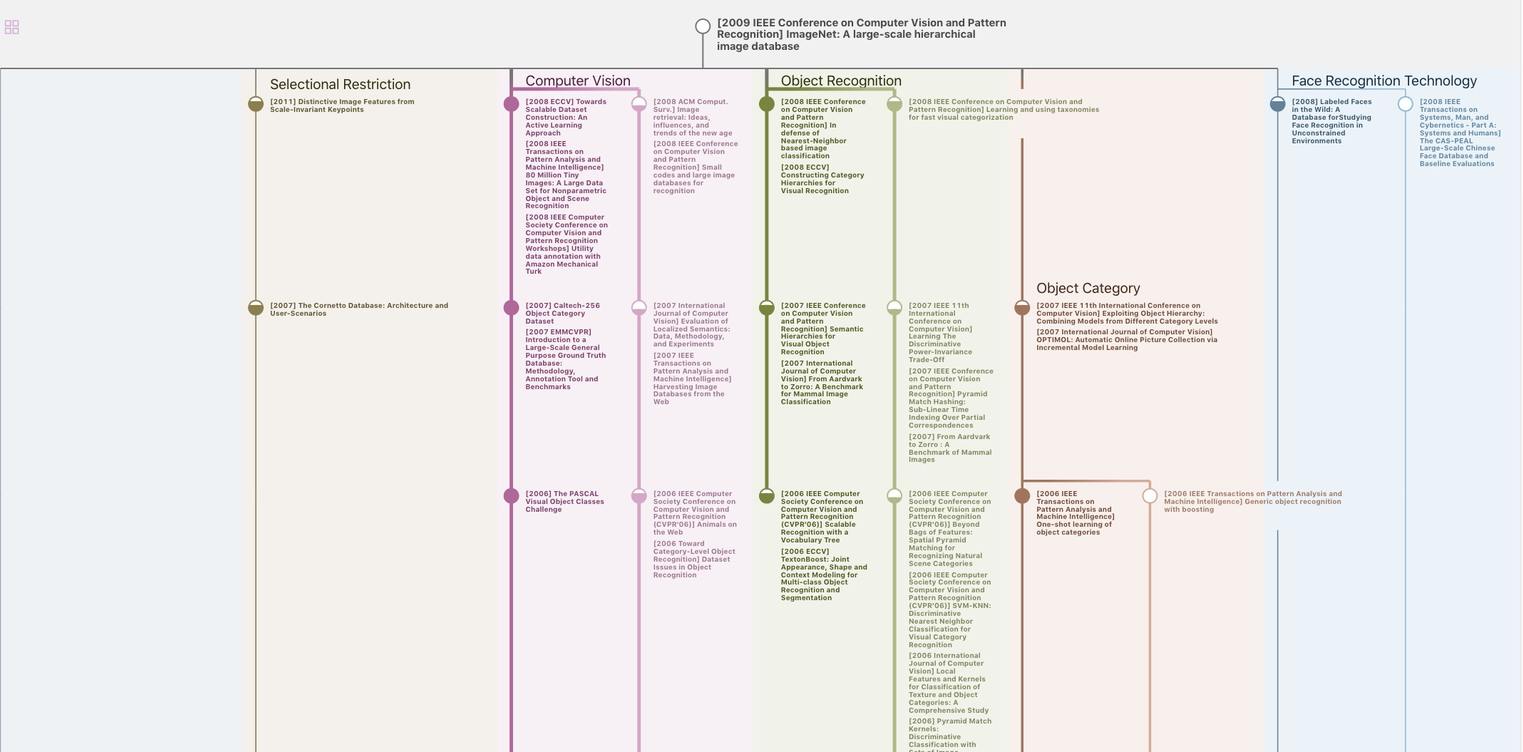
生成溯源树,研究论文发展脉络
Chat Paper
正在生成论文摘要