A Phase-Change Memristive Reinforcement Learning for Rapidly Outperforming Champion Street-Fighter Players
ADVANCED INTELLIGENT SYSTEMS(2023)
摘要
The interactions with humans, and simultaneously, making of real-time decisions in physical systems, are involved in many applications of artificial intelligence. An example of these conditions is maneuver sports. Movement-type simulations, viz., the esports game Street Fighter (SF), recapitulate the complex multicharacter interactions and, concurrently, generate the millisecond-level control challenges of human athletes. Herein, the physical and mental signatures of the SF agent (it is called SF R2) are controlled by utilizing a previously unreported model-free, natural, deep reinforcement learning algorithm "Decay-based Phase-change memristive character-type Proximal Policy Optimization" (DP-PPO) through an assemblage of hybrid case-type training processes; and an integrated training configuration for time-trial evaluations, as well as competitions with a world's best SF player, is developed. A short length of time utilized by the SF R2 to defeat the opponent and, simultaneously, maintaining a good health level is achieved, as well as excellent handling of imperfect information settings. Training studies reveal a moderate maneuver etiquette in the SF R2, along with rapid, effective head-to-head competitions with one of the world's best SF player. This paves the way for achieving a broadly applicable training scheme, capable of quickly controlling complicated-movement systems in fields where agents should observe unspecified human norms.
更多查看译文
关键词
agents,esports games,human players,phase-change memory materials,reinforcement learning
AI 理解论文
溯源树
样例
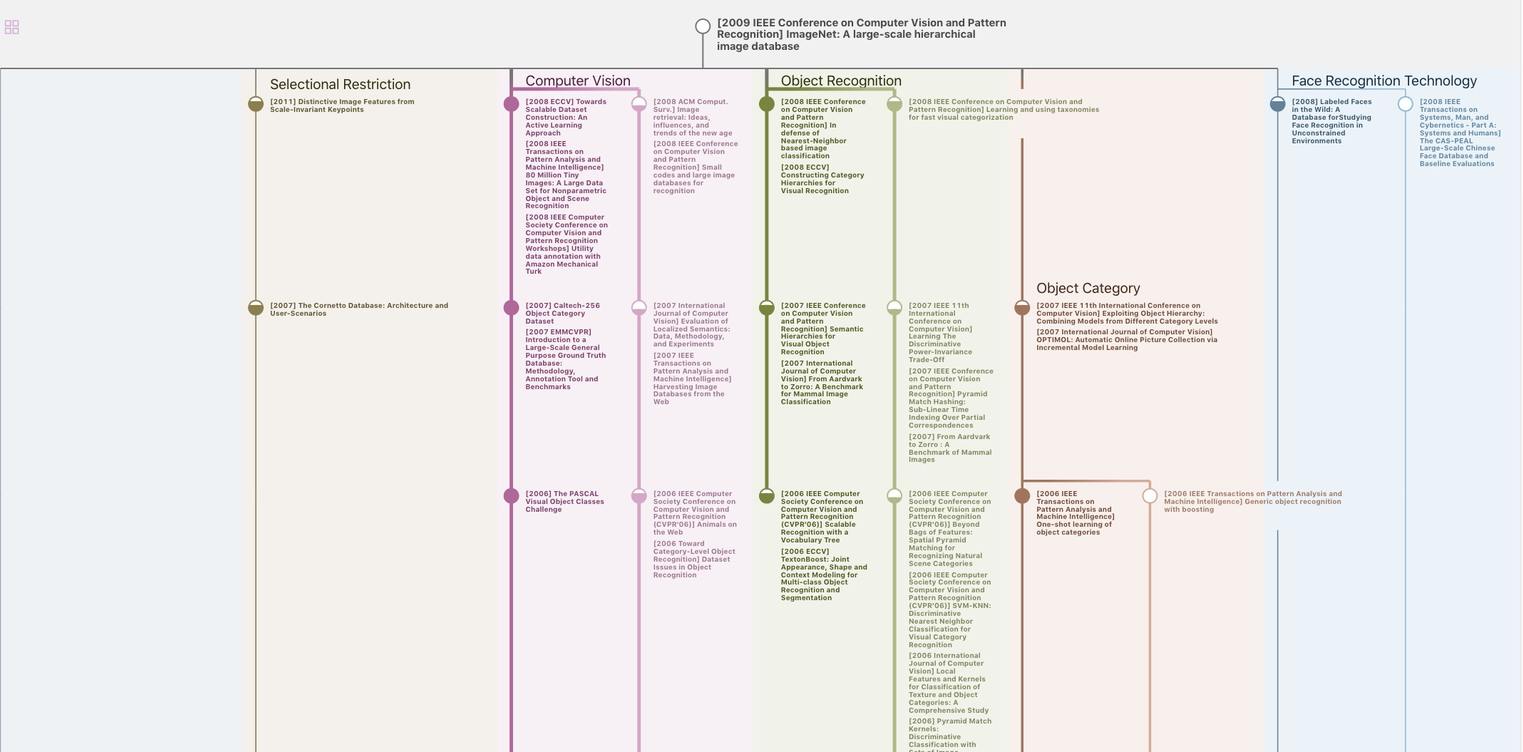
生成溯源树,研究论文发展脉络
Chat Paper
正在生成论文摘要