Transfer multiscale adaptive convolutional neural network for few-shot and cross-domain bearing fault diagnosis
MEASUREMENT SCIENCE AND TECHNOLOGY(2023)
摘要
Bearing intelligent fault diagnosis has been researched comprehensively in recent years. However, the scarcity of labeled training samples and various working conditions seriously hinder the widespread application of deep learning based fault diagnosis methods. To address this problem, we propose a transfer multiscale adaptive convolutional neural network (TMACNN), which significantly enhances the performance of deep learning based methods on few-shot and cross-domain bearing fault diagnosis in terms of network architecture and transfer strategy. On the one hand, we design a novel multiscale adaptive convolutional neural network (MACNN) framework that effectively improves the feature extraction and generalization abilities for bearing fault diagnosis by introducing mega-scale convolutions and continuous stacked multiscale convolutions. On the other hand, we propose an innovative transfer strategy for the MACNN that freezes the six stacked multiscale convolutional feature extraction units and fine-tunes the mega-scale convolution unit and the classifier, which are more suitable for few-shot transfer learning. In experiments on the CWRU dataset and Paderborn dataset, our proposed TMACNN outperforms various advanced baseline models for few-shot and cross-domain bearing fault diagnosis.
更多查看译文
关键词
multiscale convolutional neural network, deep learning, transfer learning, intelligent fault diagnosis
AI 理解论文
溯源树
样例
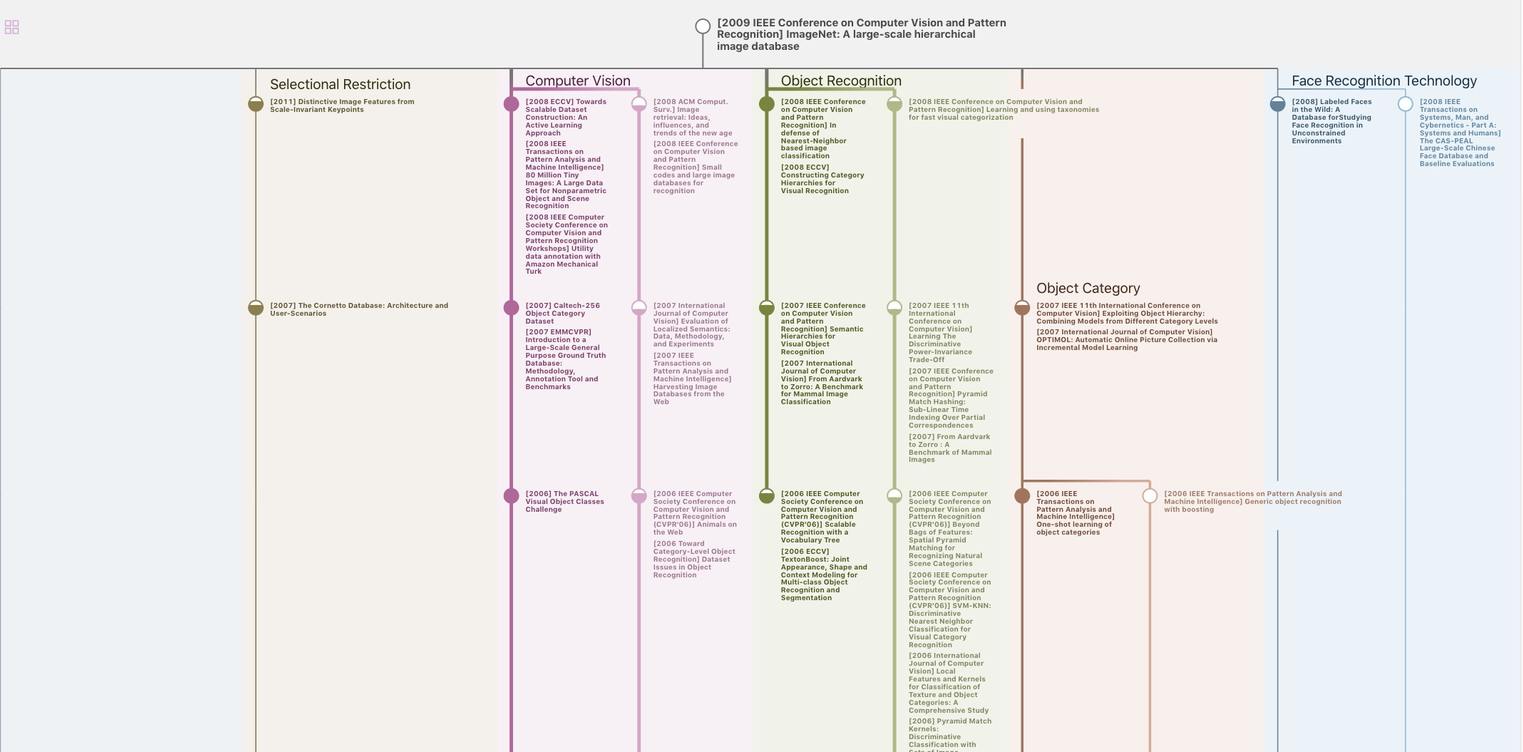
生成溯源树,研究论文发展脉络
Chat Paper
正在生成论文摘要