Performance Evaluation of Machine Learning and Deep Learning-Based Models for Predicting Remaining Capacity of Lithium-Ion Batteries
APPLIED SCIENCES-BASEL(2023)
摘要
Lithium-ion batteries are widely used in electric vehicles, smartphones, and energy storage devices due to their high power and light weight. The goal of this study is to predict the remaining capacity of a lithium-ion battery and evaluate its performance through three machine learning models: linear regression, decision tree, and random forest, and two deep learning models: neural network and ensemble model. Mean squared error (MSE), mean absolute error (MAE), coefficient of determination (R-squared), and root mean squared error (RMSE) were used to measure prediction accuracy. For the evaluation of the artificial intelligence model, the dataset was downloaded and integrated with measurement data of the CS2 lithium-ion battery provided by the University of Maryland College of Engineering. As a result of the study, the RMSE of the linear regression model was 0.045, the decision tree model was 0.038, the random forest model was 0.034, the neural network model was 0.032, and the ensemble model was 0.030. According to the measured values, the ensemble model showed the best predictive performance, followed by the neural network model. Decision tree and random forest models also showed very good performance, and the linear regression model showed relatively poor predictive performance compared to the other models.
更多查看译文
关键词
lithium-ion battery,ensemble model,neural network model,random forest model,decision tree model,linear regression model,remaining capacity
AI 理解论文
溯源树
样例
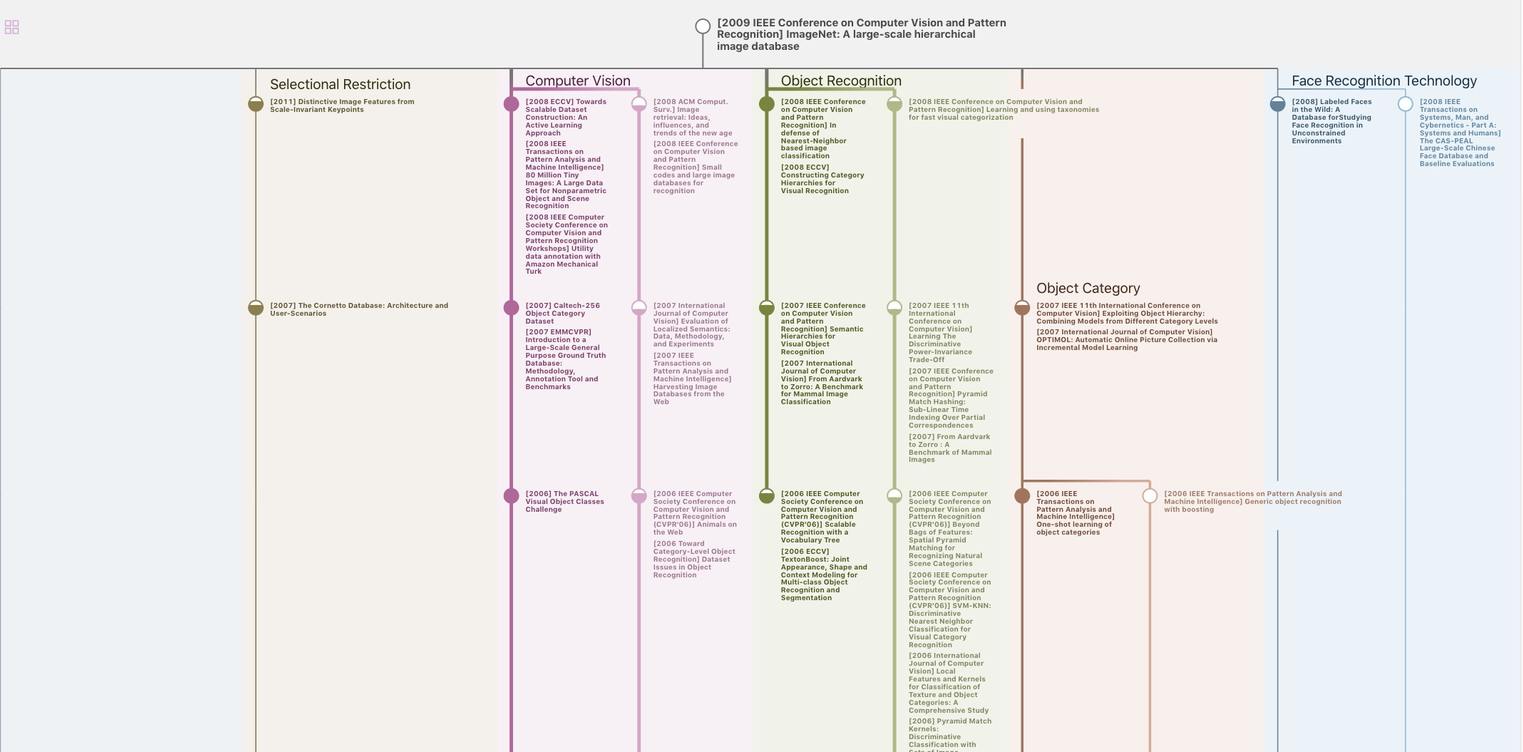
生成溯源树,研究论文发展脉络
Chat Paper
正在生成论文摘要