Stochastic distributionally robust unit commitment with deep scenario clustering
ELECTRIC POWER SYSTEMS RESEARCH(2023)
摘要
The increasing penetration of intermittent renewable generation in power systems allows for more available historical data at hand. However, it is challenging for traditional distributionally robust optimization to capture the difference and heterogeneity in historical samples which makes the solution remain highly conservative. To comprehensively characterize the underlying factors of uncertainty, we proposed a data-driven stochastic distributionally robust optimization model for unit commitment via group-wise ambiguity set constructed by deep representation clustering method. The expectation of the worst-case distribution under a group of scenarios is calculated in the model, with assuming that the scenarios belong to different groups which are ambiguity. A tractable approximation of the model is derived to avoid computational burden and we analyze the optimality conditions of the approximate formulation. Simulations on a modified IEEE-118 illustrate that the proposed approach can make a trade-off between stochastic optimization and distributionally robust optimization and has benefits in reducing the operation costs with the capability to hedge against the perturbation of unrelated samples.
更多查看译文
关键词
Unit commitment,Stochastic distributionally robust optimization,Deep representation learning clustering,Group-wise ambiguity sets
AI 理解论文
溯源树
样例
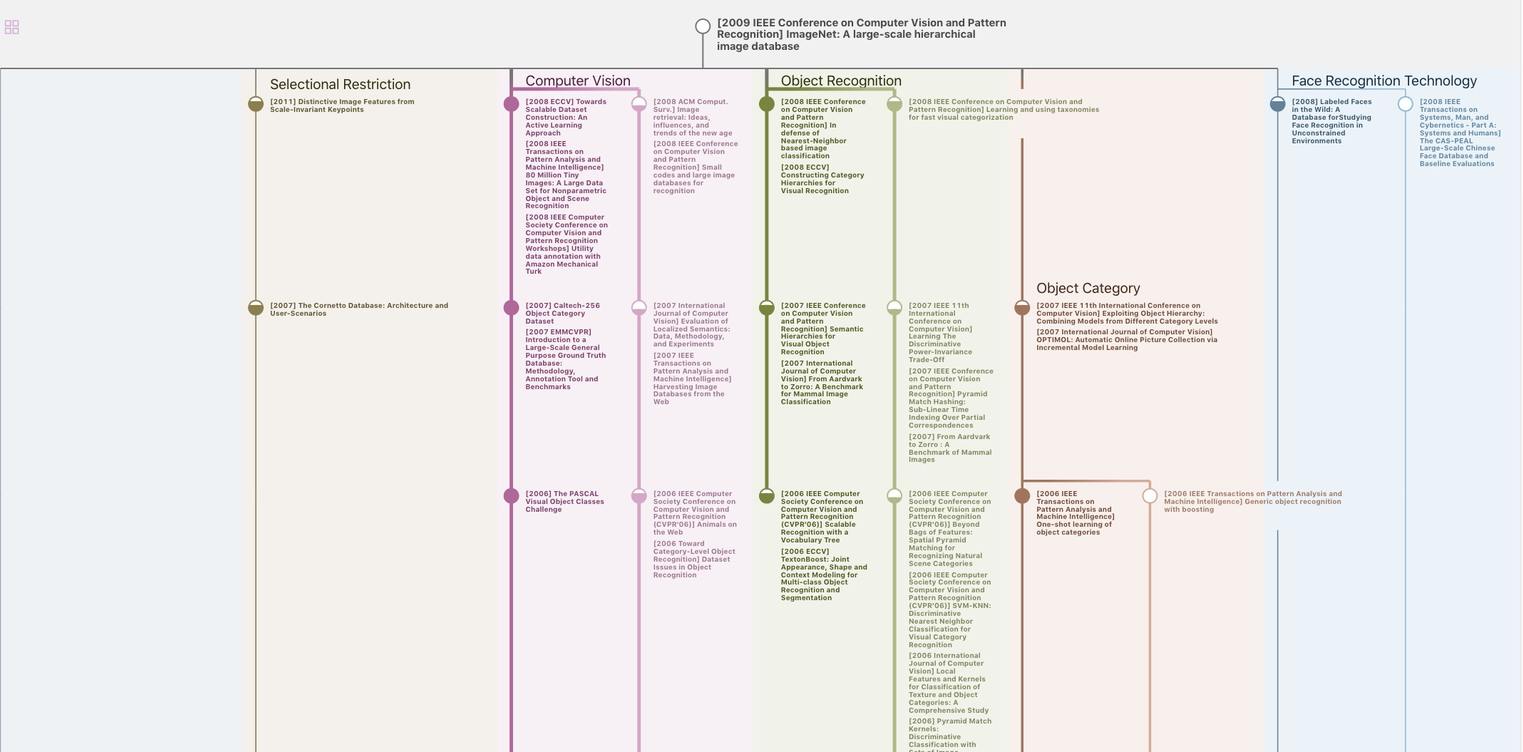
生成溯源树,研究论文发展脉络
Chat Paper
正在生成论文摘要