Holdout predictive checks for Bayesian model criticism
JOURNAL OF THE ROYAL STATISTICAL SOCIETY SERIES B-STATISTICAL METHODOLOGY(2024)
摘要
Bayesian modelling helps applied researchers to articulate assumptions about their data and develop models tailored for specific applications. Thanks to good methods for approximate posterior inference, researchers can now easily build, use, and revise complicated Bayesian models for large and rich data. These capabilities, however, bring into focus the problem of model criticism. Researchers need tools to diagnose the fitness of their models, to understand where they fall short, and to guide their revision. In this paper, we develop a new method for Bayesian model criticism, the holdout predictive check (HPC). Holdout predictive check are built on posterior predictive check (PPC), a seminal method that checks a model by assessing the posterior predictive distribution on the observed data. However, PPC use the data twice-both to calculate the posterior predictive and to evaluate it-which can lead to uncalibrated p-values. Holdout predictive check, in contrast, compare the posterior predictive distribution to a draw from the population distribution, a heldout dataset. This method blends Bayesian modelling with frequentist assessment. Unlike the PPC, we prove that the HPC is properly calibrated. Empirically, we study HPC on classical regression, a hierarchical model of text data, and factor analysis.
更多查看译文
关键词
Bayesian,model criticism,predictive checks
AI 理解论文
溯源树
样例
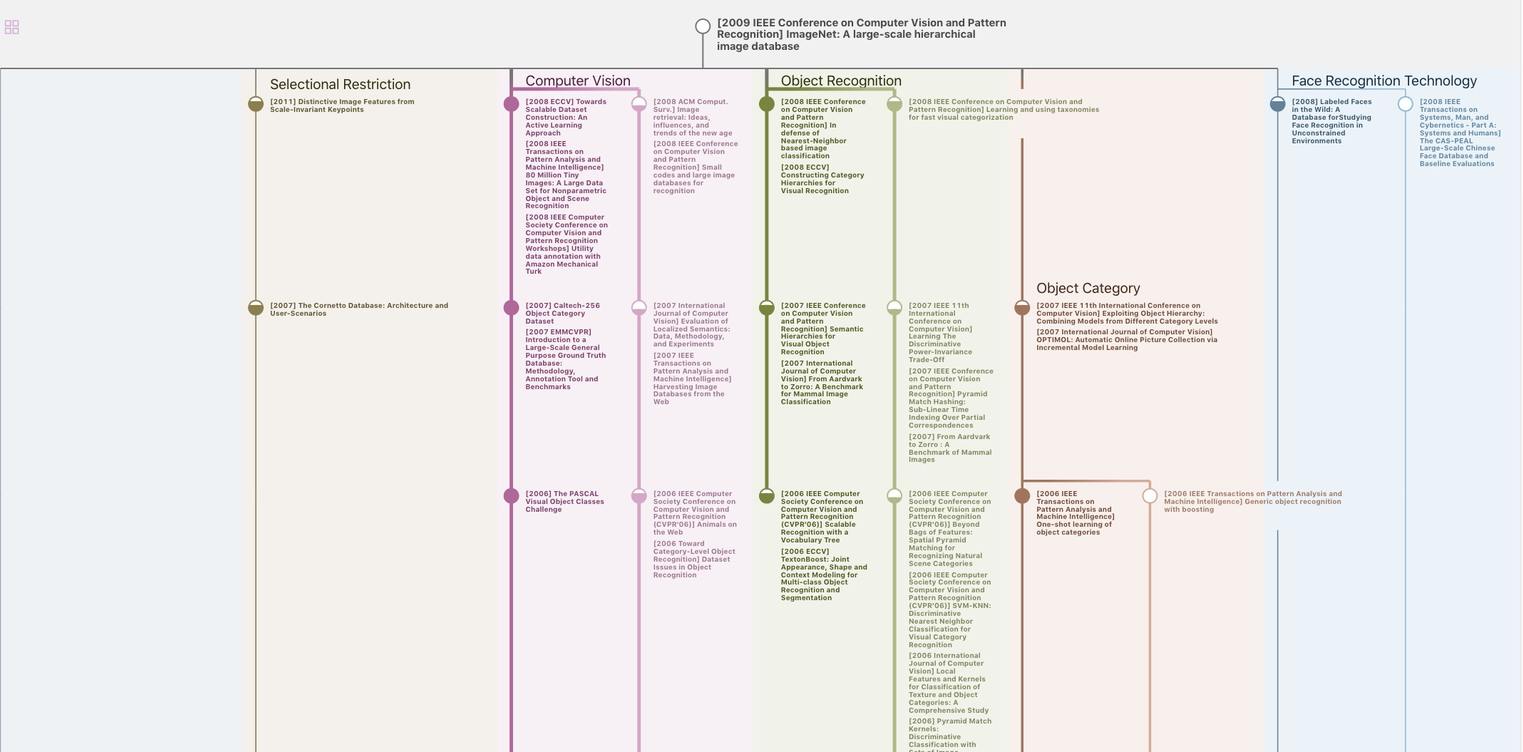
生成溯源树,研究论文发展脉络
Chat Paper
正在生成论文摘要