A distributional framework for evaluation, comparison and uncertainty quantification in soft clustering
INTERNATIONAL JOURNAL OF APPROXIMATE REASONING(2023)
摘要
In this article, we propose and study a general framework for comparing and evaluating soft clusterings, viewed as a form of uncertainty quantification for clustering tasks, with the aim of studying the uncertainty that arises from such a comparison. Our proposal is based on the interpretation of soft clustering as describing uncertain information, represented in the form of a mass function, about an unknown, target hard clustering. We present a general construction to this purpose, called distributional measures, whereby any evaluation measure can be naturally extended to soft clustering. We study the theoretical properties of the proposed approach, both in terms of computational complexity and metric properties. Furthermore, we study its relationship with other existing proposals providing, in particular, necessary and sufficient conditions for the equivalence between distributional measures and the recently proposed framework of transport-theoretic measures. We also propose sampling-based approximation algorithms with convergence guarantees, making it possible to apply the proposed method to real-world datasets. Finally, we apply the distributional measures in a computational experiment in order to illustrate their usefulness.
更多查看译文
关键词
Clustering analysis,Soft clustering,Evaluation,Validation,Comparison
AI 理解论文
溯源树
样例
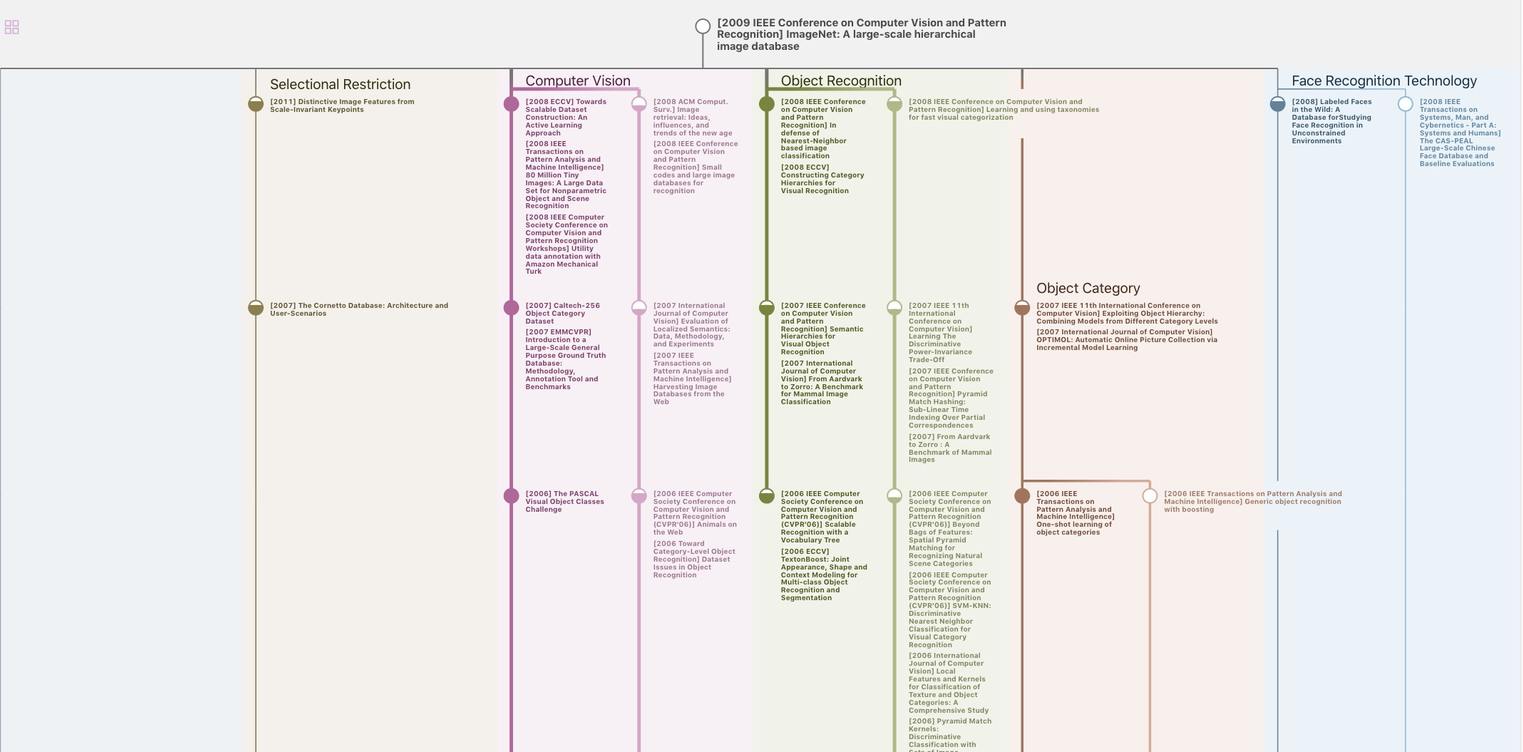
生成溯源树,研究论文发展脉络
Chat Paper
正在生成论文摘要