Causal inference based on non-experimental data in health inequality research
HANDBOOK OF HEALTH INEQUALITIES ACROSS THE LIFE COURSE(2023)
摘要
This chapter introduces different methods of causal analysis that are increasingly applied in health inequality research. The counterfactual model of causality and directed acyclic graphs (DAGs) are presented as the two important and complementary conceptual backbones of the various methods of causal inference. Definitions of a causal effect and potential biases are given and illustrated based on examples from research on health inequalities. Various cross-sectional methods are introduced that rely either on the assumption of selection on observables, such as regression (adjustment), matching and inverse probability weighting, or on the assumption of selection on unobservables, such as instrumental variable, and regression discontinuity design approaches. The before-after and the difference-in-differences estimator are presented as basic longitudinal estimators. The chapter ends with a brief guidance for causal inference for applied research.
更多查看译文
关键词
health inequality research,non-experimental
AI 理解论文
溯源树
样例
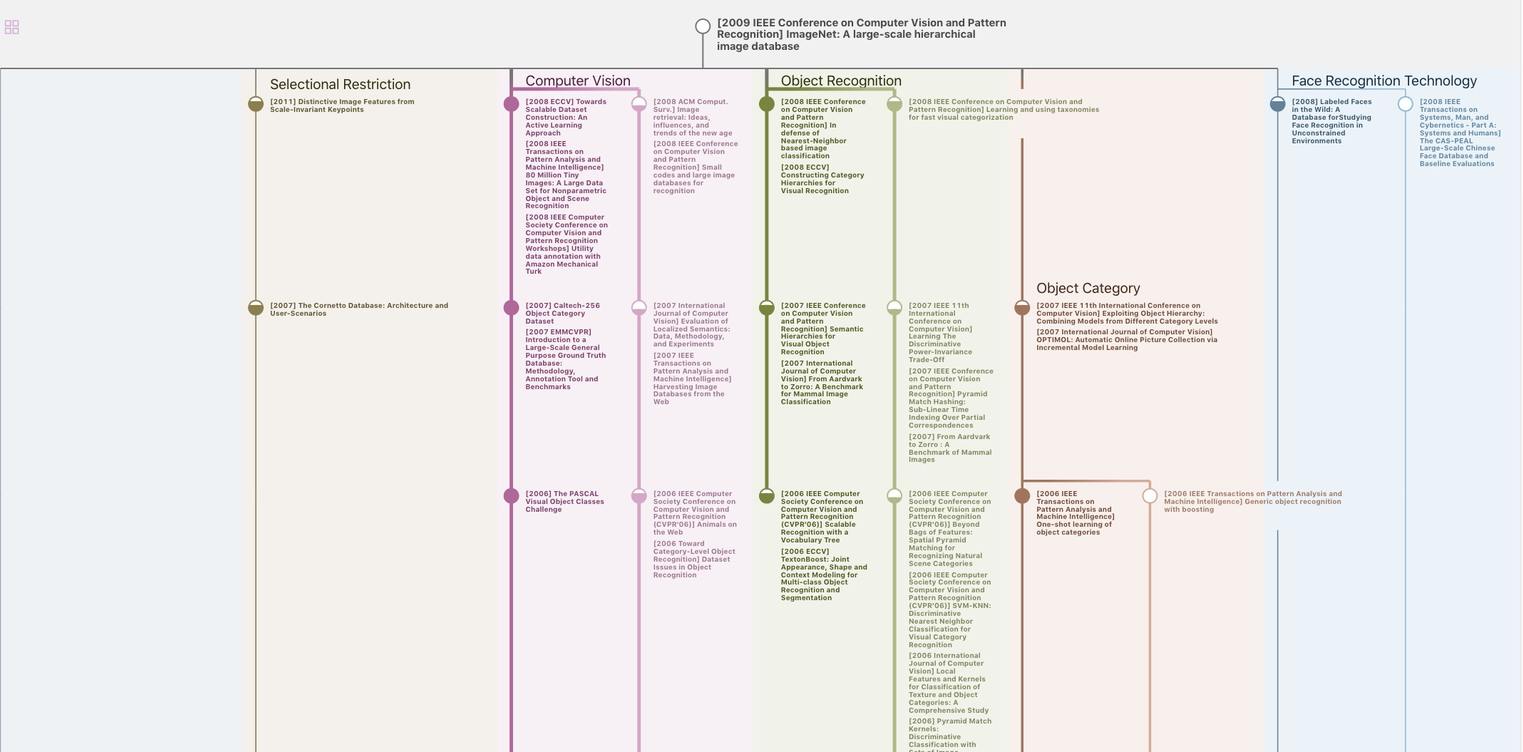
生成溯源树,研究论文发展脉络
Chat Paper
正在生成论文摘要