Prediction and identification of nonlinear dynamical systems using machine learning approaches
JOURNAL OF INDUSTRIAL INFORMATION INTEGRATION(2023)
摘要
Nonlinear dynamical systems are widely implemented in many areas. The prediction and identification of these dynamical systems purely based on observational data are of great significance for practical applications. In the work, we develop a machine learning based approach called Runge-Kutta guided next-generation reservoir computing (RKNG-RC). The proposed scheme can process data information generated by the most complicated nonlinear dynamical systems such as chaotic Lorenz63 system even with noise, and experimental systems such as chaotic Chua's electronic circuit, showing an outstanding ability for prediction tasks. More importantly, the RKNG-RC is found to have distinctive interpretability that from the trained weights the ordinary differential equation governing the observable data can be deduced, which is beyond the processing capacities of traditional approaches. The work provides an efficient platform for processing information generated by various dynamical systems.
更多查看译文
关键词
Prediction,Chaotic dynamical systems,Identification,Reservoir computing,Runge–Kutta
AI 理解论文
溯源树
样例
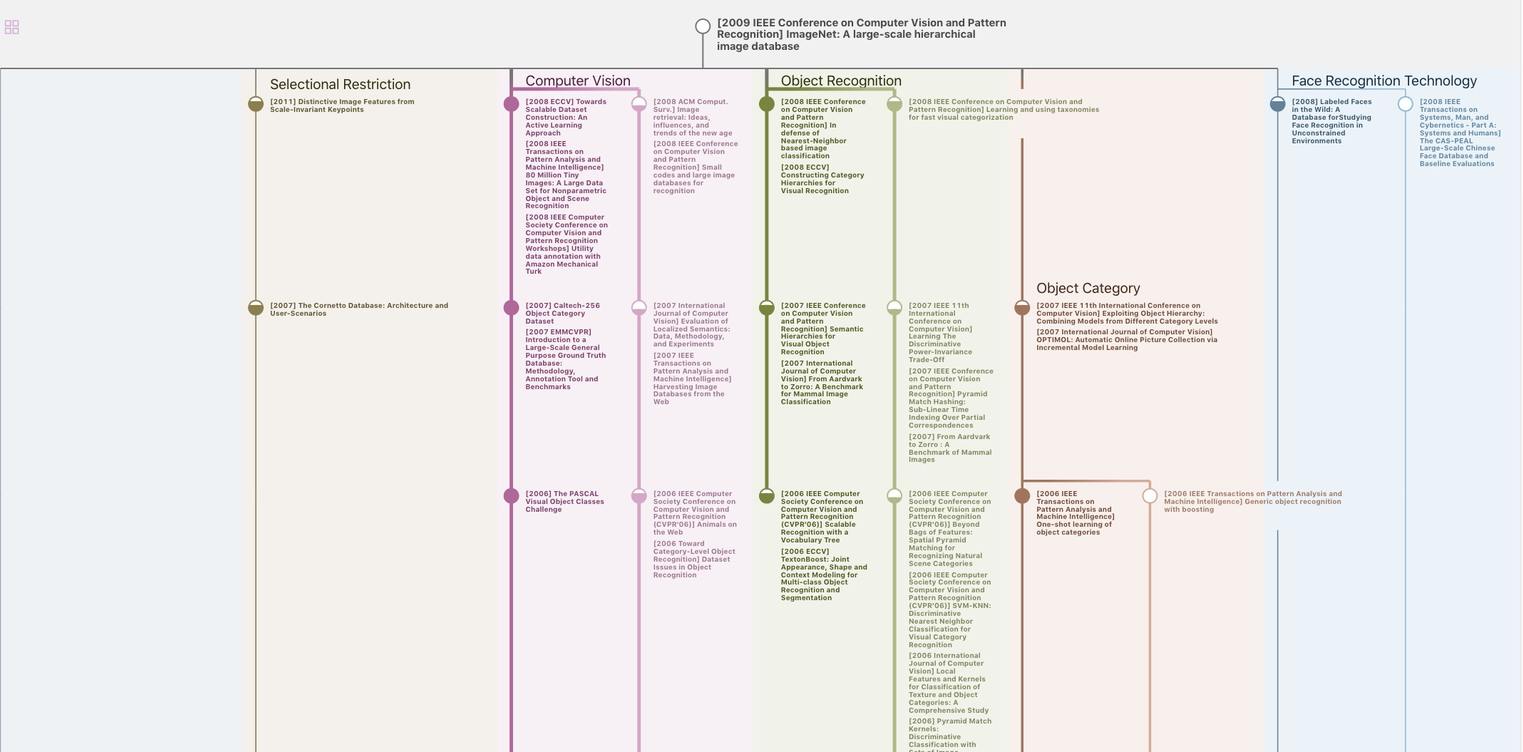
生成溯源树,研究论文发展脉络
Chat Paper
正在生成论文摘要