Dealer markets: A reinforcement learning mean field game approach
NORTH AMERICAN JOURNAL OF ECONOMICS AND FINANCE(2023)
摘要
We study the problem of finding an equilibrium strategy in an Over The Counter (OTC) market populated by multiple strategic dealers quoting the bid–ask prices. The need for an equilibrium strategy comes from the assumption that each dealer adapts their behavior by learning the optimal quoting strategy. Hence, we model the market as a game between many agents competing for resources. Based on this framework, we propose an efficient numerical procedure using Reinforcement Learning and Mean Field Games which learns an approximate equilibrium. Through an experimental campaign, we validate the proposed method in a realistic market-making scenario against strategic dealers trained to exploit our weaknesses and evaluate their performance against non-strategic agents.
更多查看译文
关键词
Dealer markets,Market making,Mean field games,Game theory,Reinforcement learning
AI 理解论文
溯源树
样例
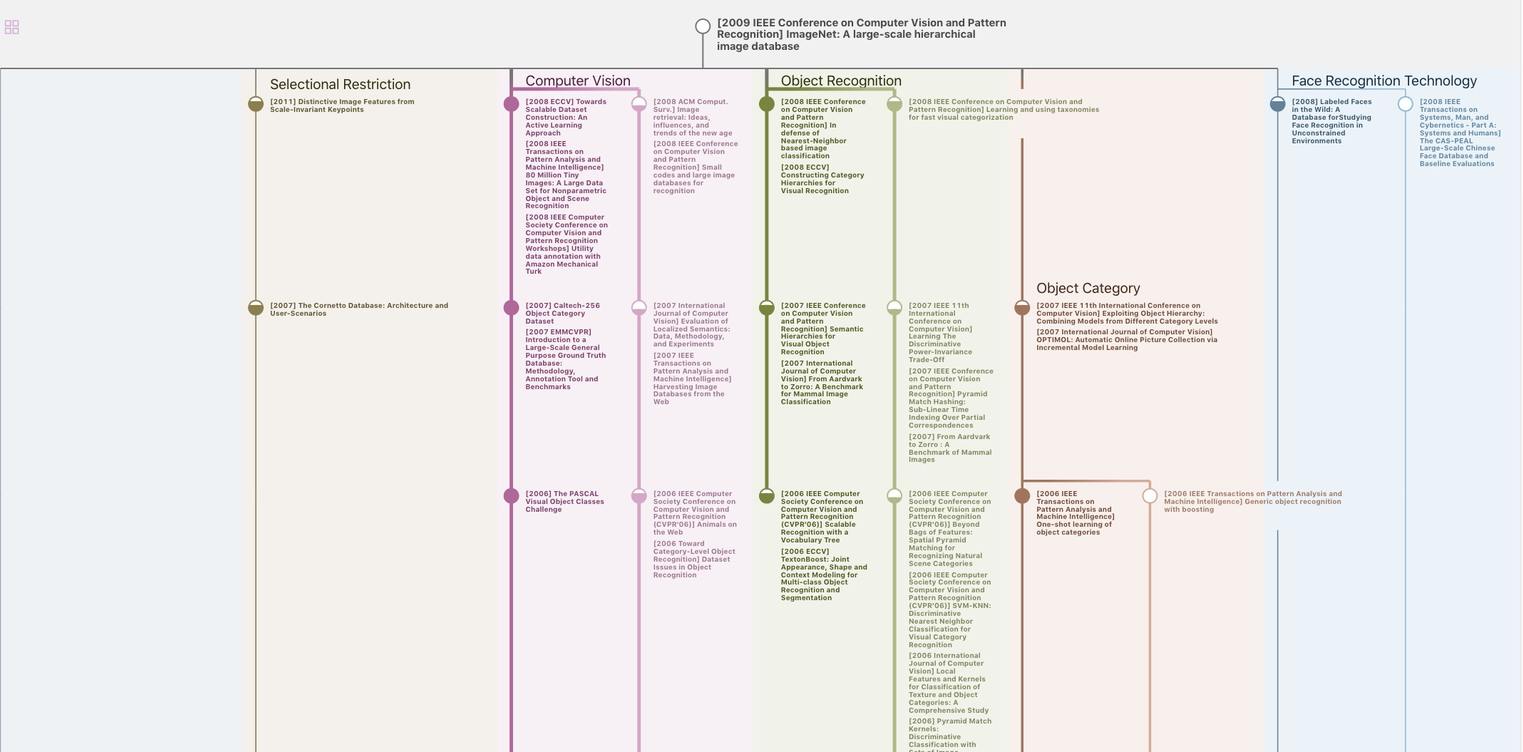
生成溯源树,研究论文发展脉络
Chat Paper
正在生成论文摘要