Counterfactual reasoning in space and time: Integrating graphical causal models in computational movement analysis
TRANSACTIONS IN GIS(2023)
摘要
Movement analysis is distinguished by an emphasis on understanding via observation and association. However, an important component of movement from the human and computer modeling perspective is the processes that bring about movement behavior in the first place. This article contextualizes the graphical causal modeling framework (for association, intervention, and counterfactual causal analysis) in GIScience, and more specifically within movement analysis studies. This is done by modeling the movement behavior of football players, applied to spatiotemporal data generated by an agent-based simulation. The movement dataset is thoroughly analyzed to infer the statistical associations among its variables, to estimate the effect of an intervention on some of those variables, and to answer a few counterfactual questions from the observations. We conclude that causal graphs (i.e., directed acyclic graphs), if implemented correctly, can assist analysts in infering causal relations from movement data. This research suggests the integration of causal graphs and agent-based paradigms as one solution for computational movement analysis.
更多查看译文
关键词
counterfactual reasoning,graphical causal models,movement,computational,space
AI 理解论文
溯源树
样例
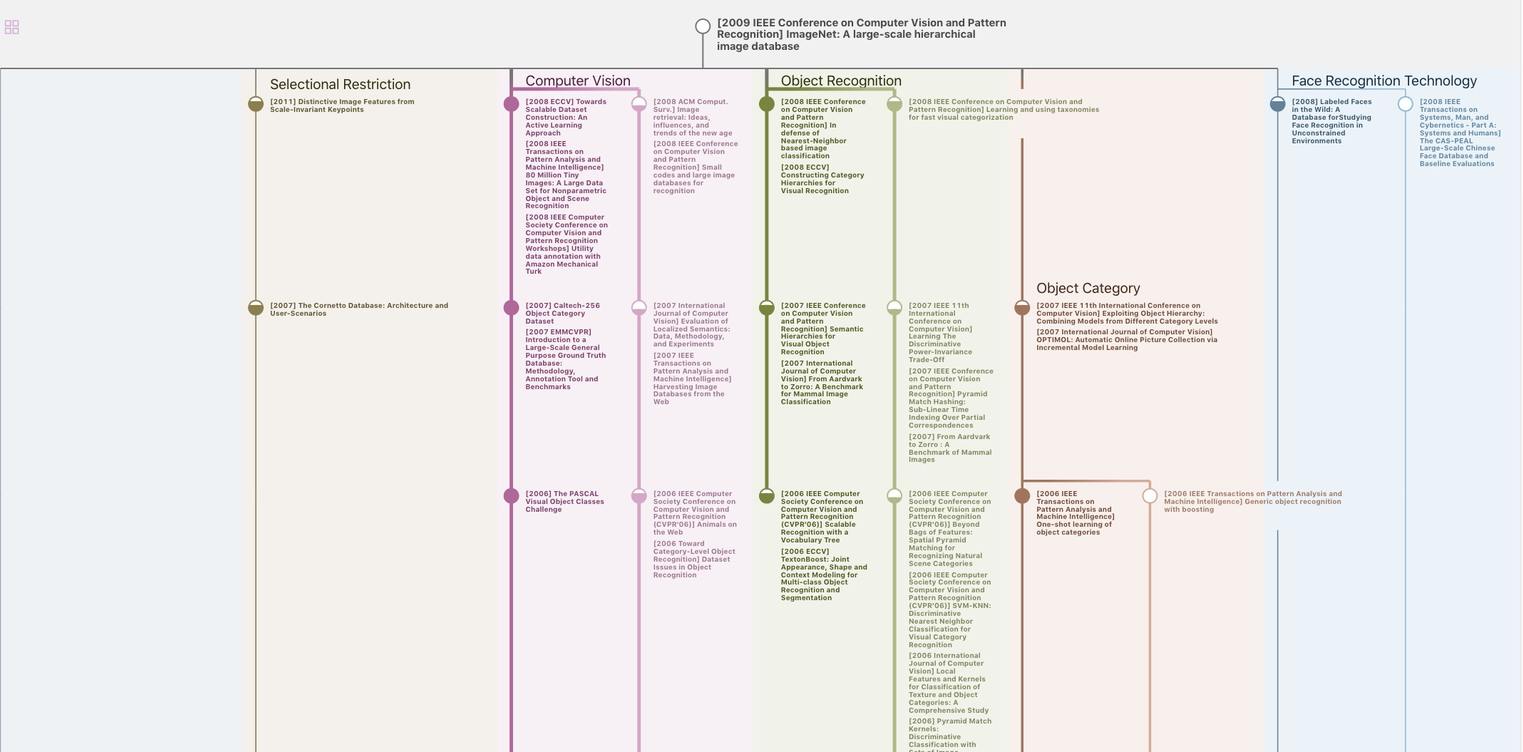
生成溯源树,研究论文发展脉络
Chat Paper
正在生成论文摘要