Deep learning to estimate RECIST in patients with cancer treated in real-world settings
JOURNAL OF CLINICAL ONCOLOGY(2023)
摘要
1564 Background: Creating large oncology clinical-genomic datasets is laborious and time-consuming. Deep learning approaches that extract RECIST outcomes at scale from observational electronic health records (EHR) data could tremendously facilitate precision oncology research. Methods: This retrospective study included patients with solid tumors treated on therapeutic clinical trials from 2004-2022 at Dana-Farber Cancer Institute with radiology reports in EHR and RECIST labels available from the tumor imaging metrics core. Each RECIST label was generated using corresponding radiology reports at a given time point. Patients were sampled into training, validation, and held-out test sets. A deep learning model (RECIST model) was trained to predict two outcomes: overall response and progressive disease at each time point, using reports from that time point and prior time points for each patient. This trained RECIST deep learning model was deployed on real-world radiology reports, and results were compared with true labels abstracted by trained human curators using the PRISSMM framework. Results: This study included 5153 patients with a total of 99,318 radiological reports who had RECIST annotations (median age at protocol enrollment [IQR] 60 years [52-57]; females 61% [3133]; and white 90% [4653]). The most common cancer types included breast (n=1006; 20%) lung (n=573; 11%), and ovarian cancer (n=539; 10%). The training subset included 4121 (79.9%) patients, the validation subset 518 (10.1%), and the held-out test set 514 (9.9%) patients. In the test set, the results showed an AUC of 0.86, and 0.87 with the best F1 scores of 0.72 and 0.63 for predicting overall response, and progressive disease, respectively. The real-world data set included 4482 patients. RECIST model evaluation on real-world radiology reports showed good performance for ascertaining PRISSMM annotations of progression from those reports (AUC 0.83, best F1 0.68), but poor performance for ascertaining PRISSMM annotations of response (AUC 0.63, best F1 0.28). Evaluation metrics are outlined in the Table. Conclusions: This study demonstrated the feasibility of using deep learning approaches to predict RECIST outcomes from radiology reports for patients with solid cancer tumors. The results show that the deep learning model accurately predicted RECIST labels on a held-out test set and real-world radiology reports for the outcome of progressive disease. These findings could accelerate precision oncology research providing a scalable way of ascertaining cancer outcomes from observational EHR data.[Table: see text]
更多查看译文
关键词
deep learning,cancer,recist,patients,real-world
AI 理解论文
溯源树
样例
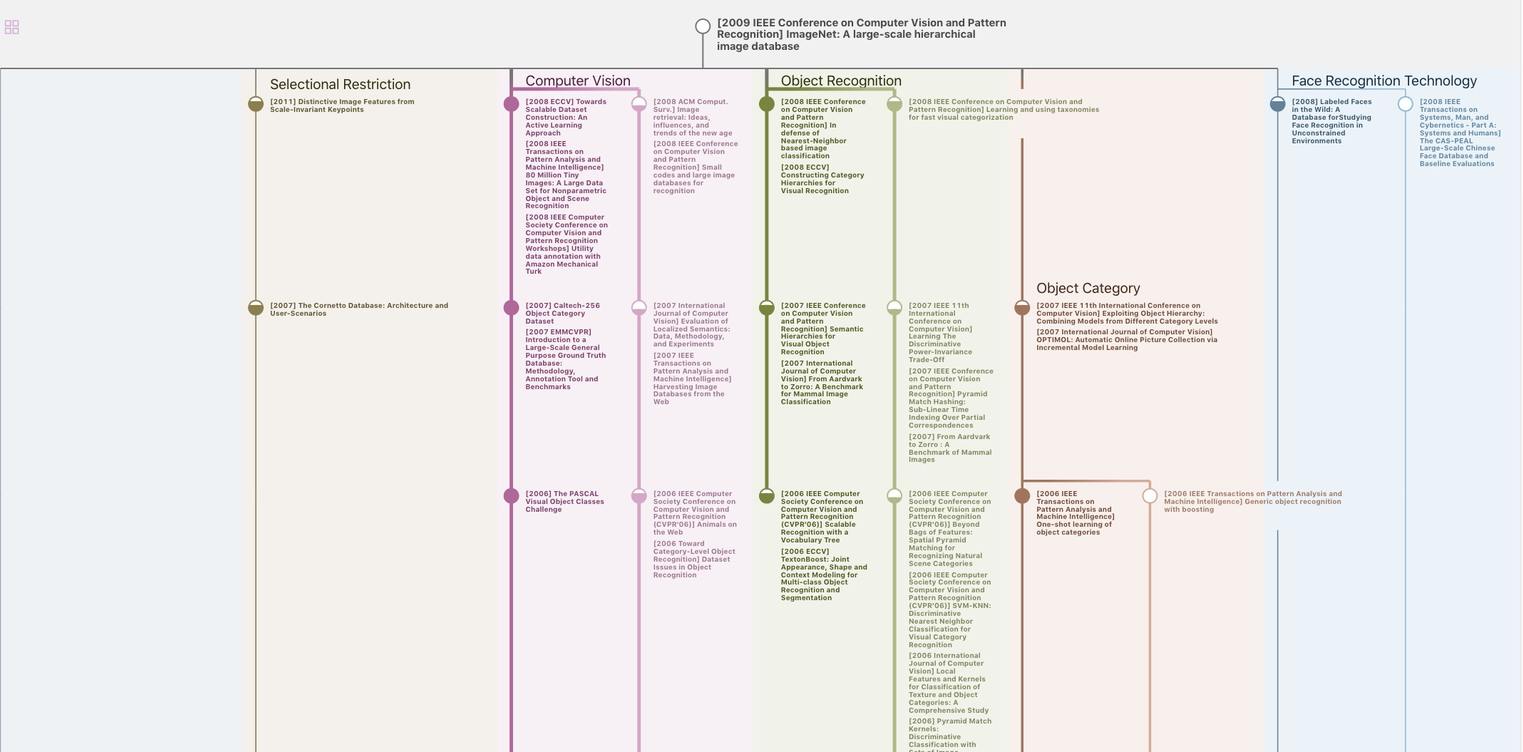
生成溯源树,研究论文发展脉络
Chat Paper
正在生成论文摘要