Development of a novel blood-based RNA gene expression platform for early-stage lung cancer diagnosis.
JOURNAL OF CLINICAL ONCOLOGY(2023)
摘要
8557 Background: Blood-based methods using circulating tumor DNA (ctDNA) and cell-free DNA (cfDNA) are under development for early and less invasive detection of lung cancer, although detection of the earliest stage cancers (stages 0-II) using these modalities is suboptimal. We hypothesized that a machine learning approach using RNA gene expression may offer important information on the biology of the patient, allowing for gene expression profiles to be used as a surrogate measurement of cancer disease phenotype and as a promising direction for early detection of lung cancer. In a previous study, 23 miRNA biomarkers were successfully discovered and validated for the non-invasive diagnostic classification of lung adenocarcinoma, achieving 97.7% sensitivity, 98.7% specificity in blood obtained from 383 clinical subjects. The aim of this study was to train a machine learning algorithm, from the 23 miRNA features, to test the signature for early lung cancer detection. Methods: A large and diverse clinical cohort was obtained from the NIH Gene Expression Omnibus database, GEO Accession Number GSE137140 ( n=3,744), comprised of miRNA extracted from serum samples consisting of subjects with pre-operative lung cancer ( n=1,566) and non-cancer controls ( n=2,178). Our analytic plan leveraged machine learning methods derived from XGBoost classification, a popular supervised-learning algorithm that uses sequentially built shallow decision trees to provide accurate results and avoidance of overfitting. The algorithm was trained using XGBoost 1.4.1.1 R library programmed with R v3.6.3. Results: The lung cancer cohort was heavily weighted towards early-stage lung cancer (87.7% stage I/II), including representation across prevalent histologic types (adenocarcinoma 77.8%, non-adenocarcinoma 22.2%) and those who self-reported as never smokers (37.9%). The 23-miRNA signature achieved 98% sensitivity, 89% specificity in the held-out test set (Table). When incorporating age and gender, the 23-miRNA signature achieved 95.5% sensitivity, 90.3% specificity. Conclusions: A machine learning approach using RNA gene expression in patient serum achieved high sensitivity and specificity in a large, predominantly early-stage, lung cancer cohort. A multi-analyte, multimodal approach that leverages machine learning algorithms with RNA gene expression profiles and available demographics and clinical risk-factors, represents the possibility to accurately detect lung cancer in the earliest stages. This approach has successfully been translated from microarray to PCR instrumentation, with further validation of this machine learning method and approach currently underway. [Table: see text]
更多查看译文
关键词
rna gene expression platform,lung cancer diagnosis,gene expression,blood-based,early-stage
AI 理解论文
溯源树
样例
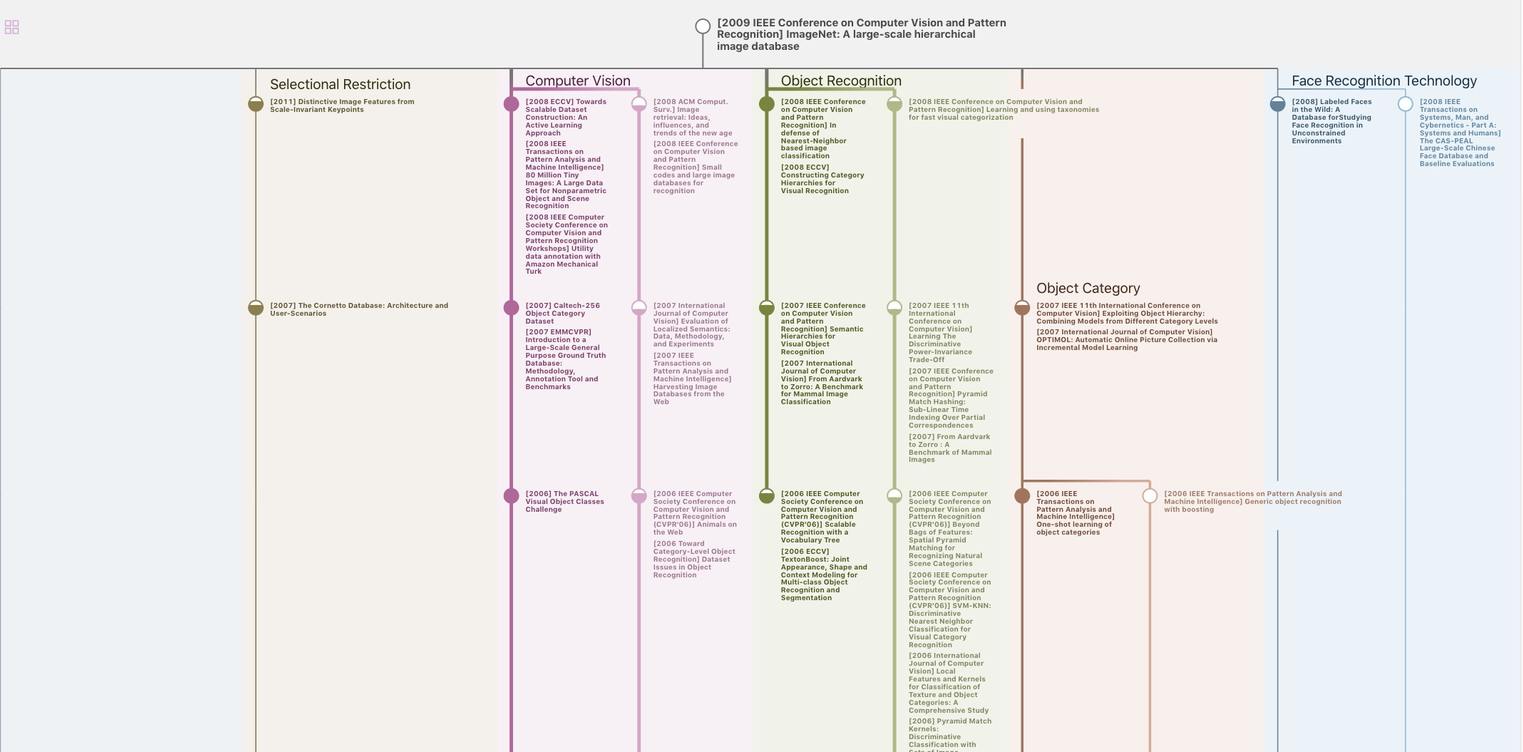
生成溯源树,研究论文发展脉络
Chat Paper
正在生成论文摘要