FedH2L: A Federated Learning Approach with Model and Statistical Heterogeneity
2023 IEEE INTERNATIONAL CONFERENCE ON JOINT CLOUD COMPUTING, JCC(2023)
摘要
Federated learning (FL) enables distributed participants to collectively learn a strong global model without sacrificing their individual data privacy. Mainstream FL approaches require each participant to share a common network architecture and further assume that data are sampled IID across participants. However, in real-world deployments, participants may require heterogeneous network architectures; and the data distribution is almost non-uniform. To address these issues we introduce FedH2L, which is agnostic to the model architecture and robust to different data distributions across participants. In contrast to approaches sharing parameters or gradients, FedH2L relies on mutual distillation, exchanging only posteriors on a shared seed set between participants in a decentralized manner. This makes it extremely bandwidth efficient, model agnostic, and crucially produces models capable of performing well on the whole data distribution when learning from heterogeneous silos.
更多查看译文
关键词
Federated Learning,Model heterogeneity,Statistical heterogeneity,Domain shift,Mutual learning
AI 理解论文
溯源树
样例
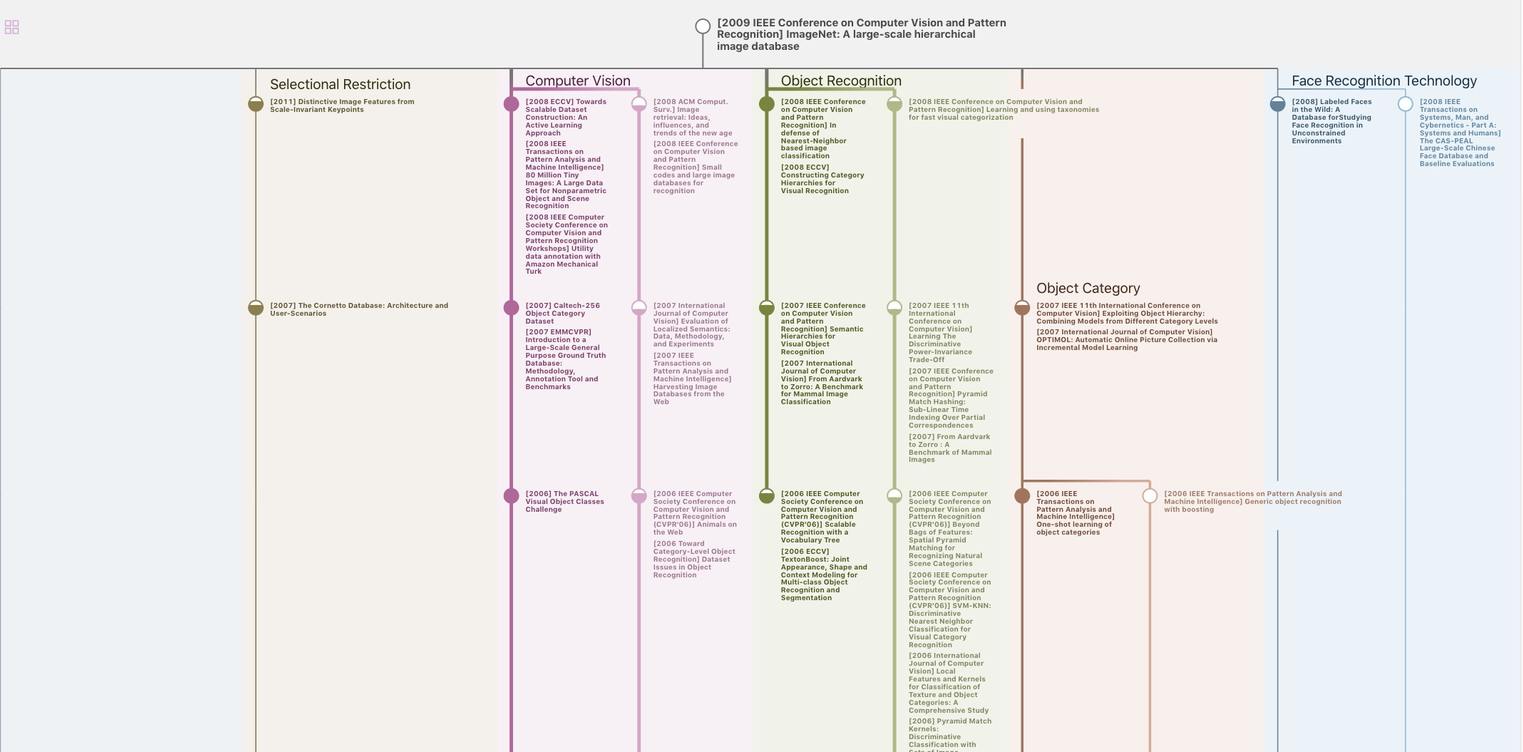
生成溯源树,研究论文发展脉络
Chat Paper
正在生成论文摘要