Application, interpretability and prediction of machine learning method combined with LSTM and LightGBM-a case study for runoff simulation in an arid area
JOURNAL OF HYDROLOGY(2023)
摘要
The runoff prediction can provide scientific basis for flood control, disaster reduction and water resources planning. Due to a large number of uncertainties in runoff prediction, it is difficult to make precise predictions. To improve the accuracy of runoff prediction, this study combines techniques of Long Short-Term Memory (LSTM) and Light Gradient Boosting Machine (LightGBM) in machine learning with reciprocal error method to develop an integrated data-driven model (i.e., LSTM-LightGBM) for runoff prediction. To demonstrate its applicability, the model is applied to the annual runoff prediction of the Caiqi hydrological monitoring station in the Shiyang River in an arid area. Indicators include Error of Peak (EP), Nash-Sutcliffe Efficiency (NSE), Root Mean Squared Error (RMSE), and Mean Absolute Error (MAE) are adopted to evaluate the prediction performance of the LSTM, LightGBM, and LSTM-LightGBM methods under the same hyperparameter combinations. Then, the interpretability of LSTM and LightGBM models is also explored based on the permutation importance method and Shapley Additive exPlanations (SHAP) values, respectively. Finally, future annual runoff at the Caiqi for the next 50 years (2025-2075) is predicted based on LSTM-LightGBM model under 12 climate scenarios. Therefore, results show that: 1. the integrated model (LSTM-LightGBM) has good performance than two single models in NSE (0.92), RMSE (0.075 million m3) and MAE (0.046 million m3) and EP value (i.e., for bridging the peak-valley runoff). 2. In this case, it is found that four feature variables have the greatest influence on the target variables through the interpretable analysis. 3. The 12 combined climate scenarios used in this investigation produced generally steady predictions. The scenarios with the highest and lowest mean values are GFDL RCP 6.0 (3.12 x 108m3) and IPSL RCP 2.6 (3.04 x 108m3), respectively, with a decrease of 24.09 % and 26.03 % compared to the mean annual runoff of 4.11 x 108m3 in the baseline period (1955-2017). These findings can provide scientific bases for future water resources planning in the downstream of the Shiyang River Basin.
更多查看译文
关键词
runoff simulation,lstm,machine learning method,machine learning
AI 理解论文
溯源树
样例
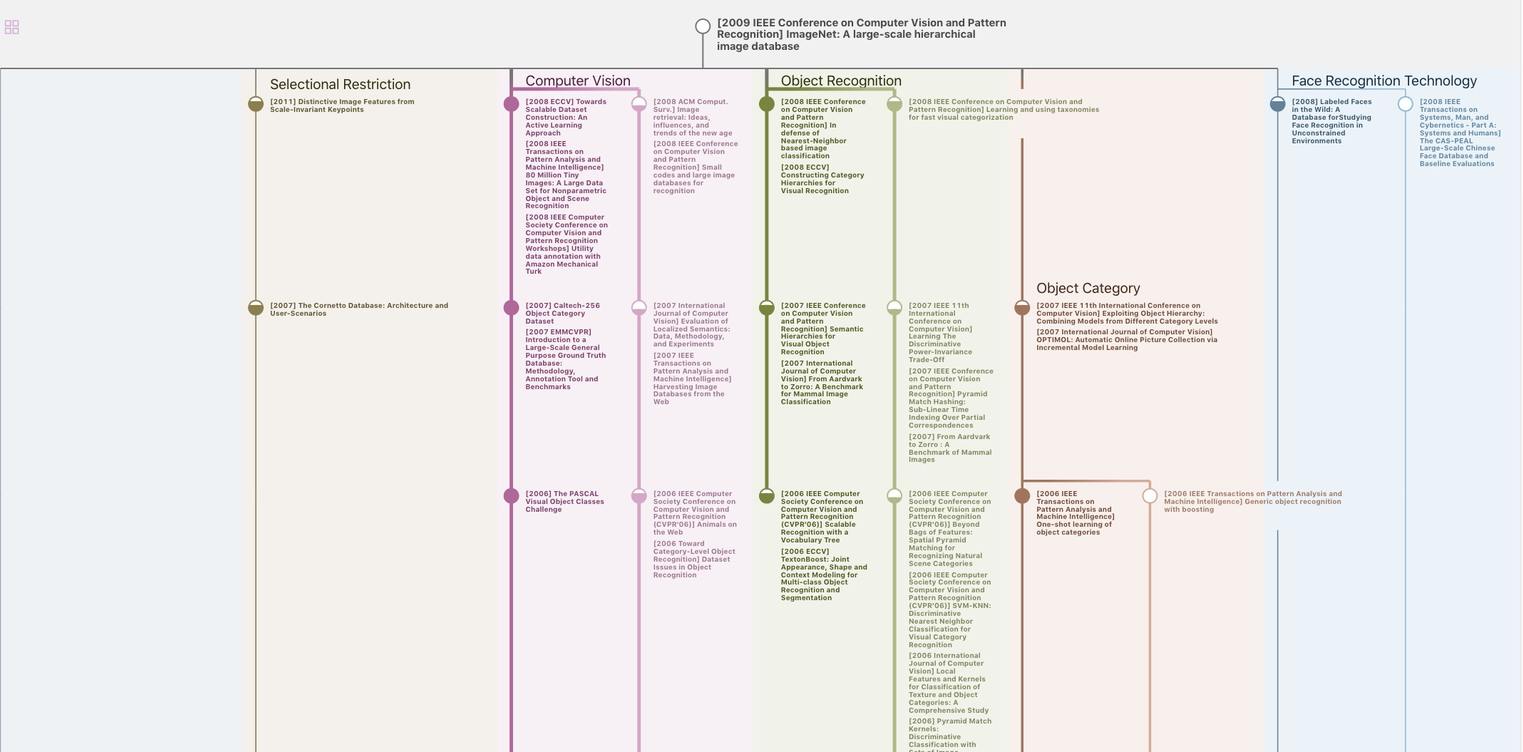
生成溯源树,研究论文发展脉络
Chat Paper
正在生成论文摘要