Realizing accurate battery capacity estimation using 4 min 1C discharging data
ENERGY(2023)
摘要
Accurate capacity estimation is important to ensure the safe operation of battery. Current data-driven capacity estimation methods mainly rely on large volume charging or discharging data, which usually lasts for tens of minutes to hours, to extract effective battery aging features. The large volume data requirement restricts the application in real-world scenarios to some extent. In this paper, a novel capacity estimation method, which only requires several-minute discharging data to realize accurate capacity estimation, is proposed. Firstly, the idea of regression shapelet is introduced and shapelet distance is defined to capture the battery degradation trend. Then maximum relevance minimum redundancy algorithm is used to select the representative shapelet feature set, which not only has strong correlation with battery capacity, but also contains least redundancy among each other. Finally, eXtreme gradient boosting is adopted as the final regressor mapping from shapelet distance to battery capacity. The proposed method is verified on the public Oxford battery dataset. Results show that 4min 1C discharging data is sufficient for the proposed method to realize accurate capacity estimation with mean absolute relative error of 0.86%, which is superior to existing methods in terms of data volume requirement or accuracy. Moreover, the proposed method is promising to be applied in vehicle-to-grid scenarios considering its light computational burden.
更多查看译文
关键词
Capacity estimation,Lithium-ion battery,Data-driven,Regression shapelet
AI 理解论文
溯源树
样例
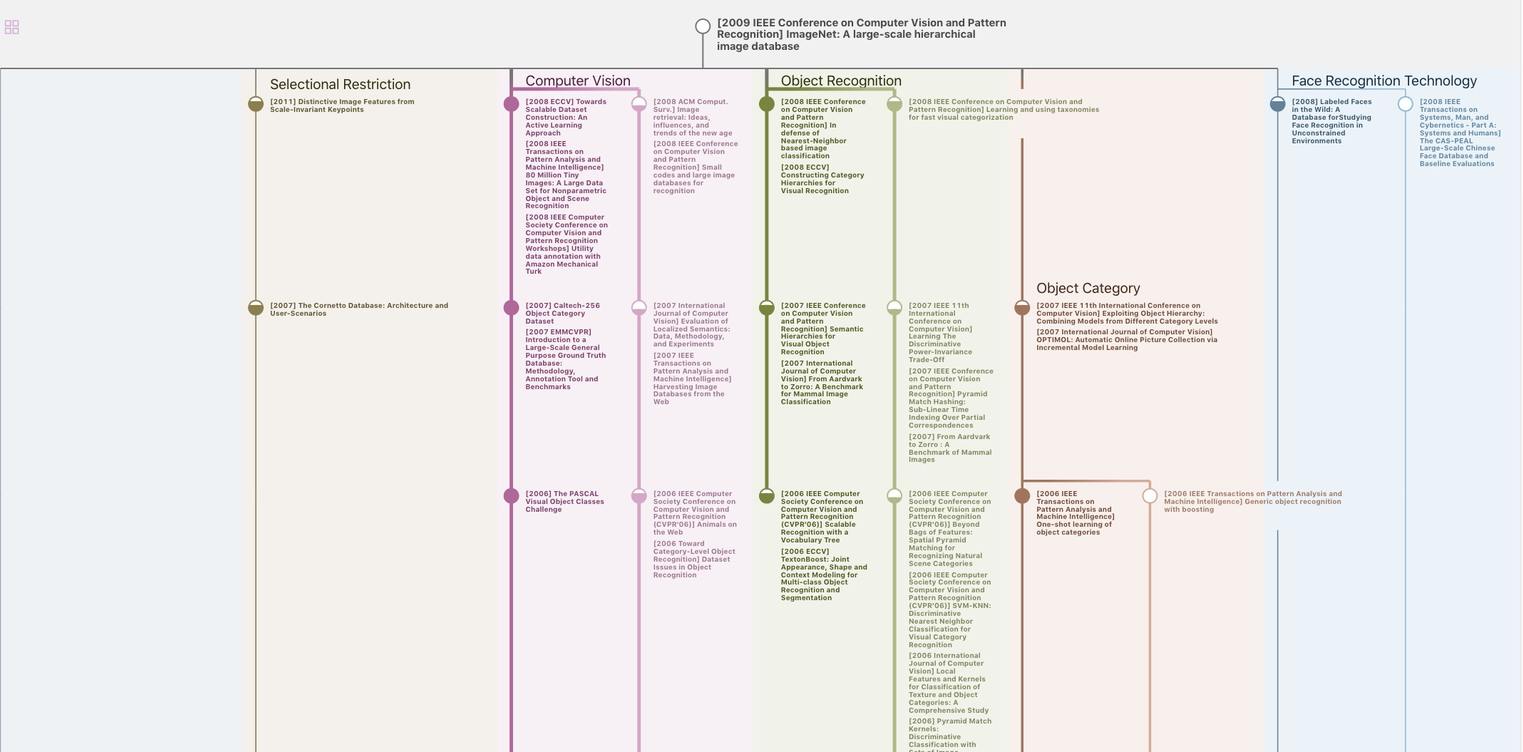
生成溯源树,研究论文发展脉络
Chat Paper
正在生成论文摘要