Deep learning-driven super-resolution reconstruction of two-dimensional explosion pressure fields
JOURNAL OF BUILDING ENGINEERING(2023)
摘要
Precise and rapid calculation of explosive loads on structures in specific explosion scenarios is crucial for blast-resistant design, emergency response and safety assessments of structures after disasters. However, available methods such as empirical models and numerical simulations are inadequate in meeting the fast computation demands for diverse explosion scenarios due to limitations in accuracy or computational cost. In order to obtain high-precision explosion pressure fields and overpressure time histories with low computational cost, this study presents a deep learning-based framework for reconstructing two-dimensional explosion pressure fields, which transforms low-resolution numerical simulation results into super-resolution outputs equivalent to high-resolution numerical simulation results. A super-resolution strategy, which concatenates high-resolution channels that record flow field information with the low-resolution channel, is proposed to improve the network performance. Based on the constructed dataset, a comparative study of three different networks is presented to assess their super-resolution applicability. Finally, the performance and generalization ability of the trained model is evaluated with super-resolution evaluation metrics, statistical evaluation metrics, and relative error distribution. The findings indicate that the super-resolution strategy can effectively improve the prediction accuracy of the network. The super-resolution model can not only provide high-credibility super-resolution pressure fields and pressure time histories, but also exhibits excellent generalization capabilities for explosive scenarios beyond the training dataset, therefore providing a new approach for realizing high-precision calculation of explosive flow fields and loads under diverse blast scenarios.
更多查看译文
关键词
Deep learning,Super-resolution strategy,Flow field reconstruction,Blast loading
AI 理解论文
溯源树
样例
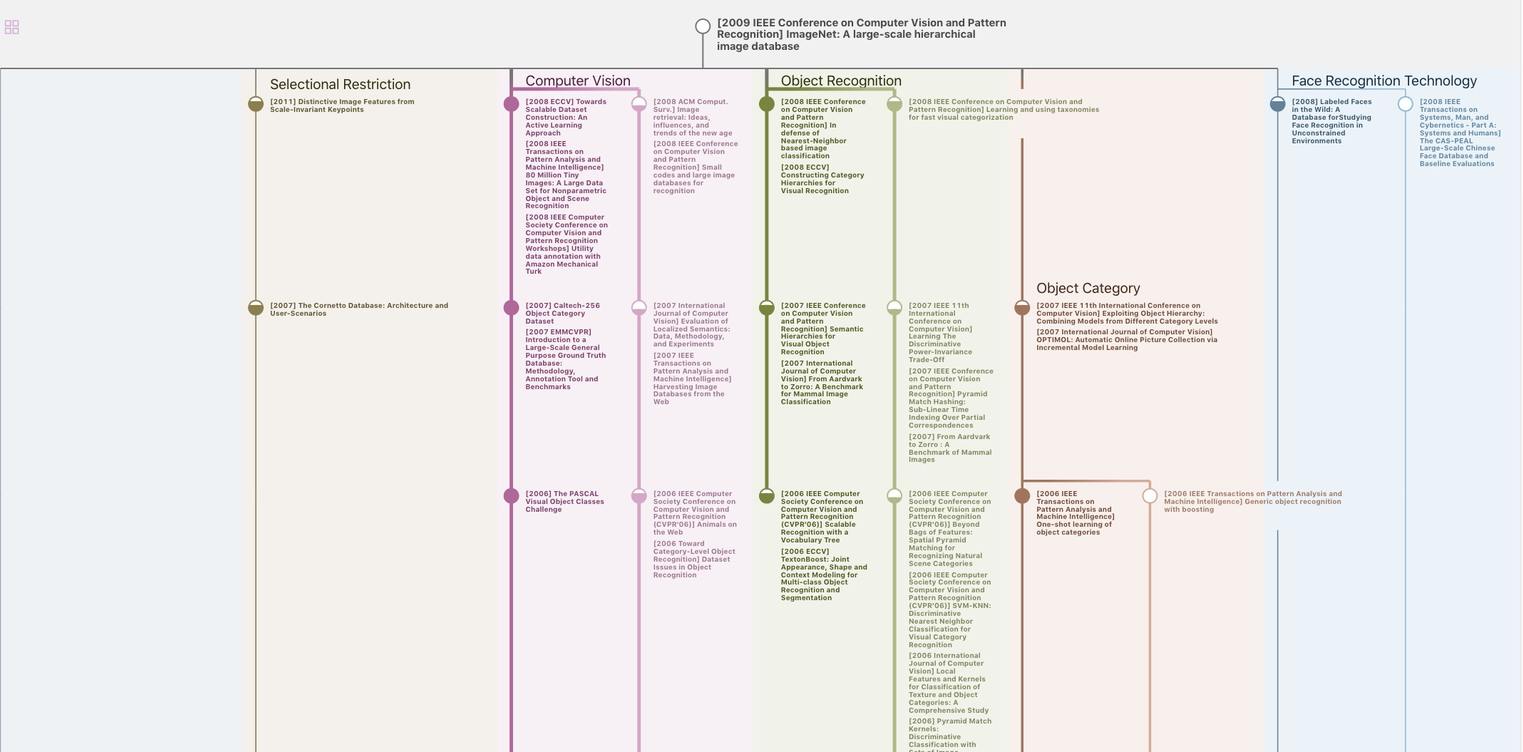
生成溯源树,研究论文发展脉络
Chat Paper
正在生成论文摘要