Bi-level Multi-Agent Actor-Critic Methods with Transformers
2023 IEEE INTERNATIONAL CONFERENCE ON JOINT CLOUD COMPUTING, JCC(2023)
摘要
Recently, deep multi-agent reinforcement learning methods have witnessed great progress, including multi-agent actor-critic methods. However, it's worth noticing there is a performance gap between multi-agent actor-critic methods and state-of-the-art value-based methods. In this paper, we investigate the causes and attribute inferior performance to issues of contribution-mismatch and indiscriminate guidance. To overcome these problems, we introduce a novel bi-level multi-agent actorcritic reinforcement learning approach with transformers, called BMT. Specifically, we propose a simple but efficient bi-level optimization mechanism to learn both global critic and agent-specific critic, thus jointly guiding the policy update. In addition, we adopt the transformer-based model as the policy network to decouple complicated relationships and generate flexible policy. BMT is also general enough to be plugged into any actor-critic multi-agent reinforcement learning approach, such as MAPPO, and equips it with strong expression. On multiple benchmarks including multi-agent particle environments and a challenging set of StarCraft II micromanagement tasks, large-scale empirical experiments demonstrate that BMT-based multi-agent reinforcement learning methods achieve superior performance over both state-of-the-art actor-critic and value-based approaches.
更多查看译文
关键词
multi-agent reinforcement learning,actor-critic method,bi-level critic
AI 理解论文
溯源树
样例
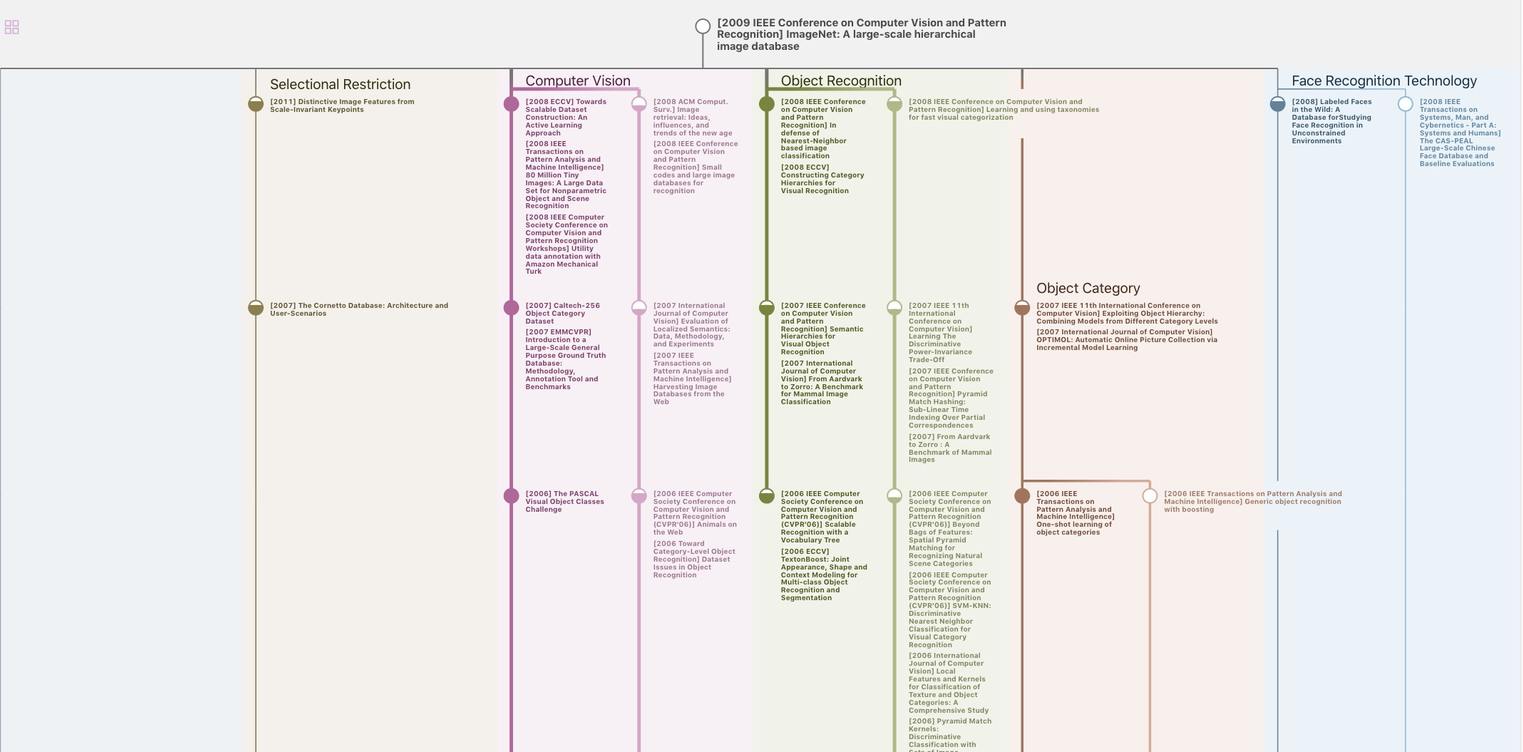
生成溯源树,研究论文发展脉络
Chat Paper
正在生成论文摘要