Improved saddle point prediction in stochastic two-player zero-sum games with a deep learning approach
ENGINEERING APPLICATIONS OF ARTIFICIAL INTELLIGENCE(2023)
摘要
In this paper, we propose a novel deep learning approach for predicting saddle points in stochastic two-player zero-sum games. Our method combines neurodynamic optimization and deep neural networks. First, we model the stochastic two-player zero-sum game as an ordinary differential equation (ODE) system using neurodynamic optimization. Second, we develop a neural network to approximate the solution to the ODE system, which includes the saddle point prediction for the game problem. Third, we introduce a specialized algorithm for training the neural network to enhance the accuracy of the saddle point prediction. Our experiments demonstrate that our model outperforms existing approaches, yielding faster convergence and more accurate saddle point predictions.
更多查看译文
关键词
Stochastic two-player zero-sum games,Saddle points,Neurodynamic optimization,Deep learning,Ordinary differential equations
AI 理解论文
溯源树
样例
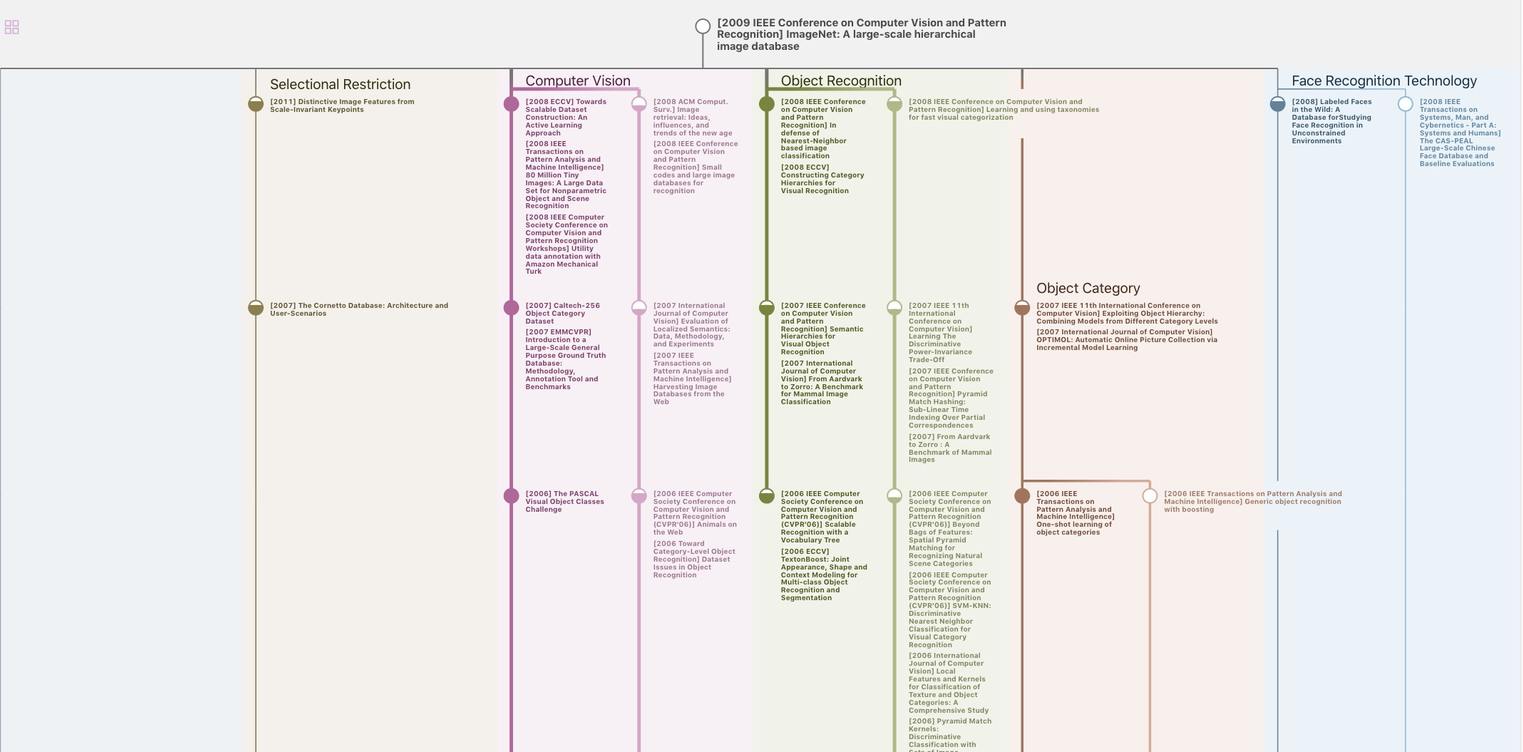
生成溯源树,研究论文发展脉络
Chat Paper
正在生成论文摘要