A Novel Fake News Detection Model for Context of Mixed Languages Through Multiscale Transformer
IEEE TRANSACTIONS ON COMPUTATIONAL SOCIAL SYSTEMS(2023)
摘要
Fake news detection has been a more urgent technical demand for operators of online social platforms, and the prevalence of deep learning well boosts its development. From the model structure, existing research works can be categorized into three types: convolution filtering-based neural network approaches, sequential analysis-based neural network approaches, and attention mechanism-based neural network approaches. However, almost all of them were developed oriented to scenes of a single language, without considering the context of mixed languages. To bridge such gap, this article extends to the basic pretraining language processing model transformer into the multiscale format and proposes a novel fake news detection model for the context of mixed languages through a multiscale transformer to fully capture the semantic information of the text. By extracting more fruitful feature levels of initial textual contents, it is expected to obtain more resilient feature spaces for the semantics characteristics of mixed languages. Finally, experiments are conducted on a postprocessed real-world dataset to illustrate the efficiency of the proposal by comparing performance with four baseline methods. The results obtained show that the proposed method has an accuracy of about 2%-10% higher than commonly used baseline models, indicating that the scheme has appropriate detection efficiency in mixed language scenarios.
更多查看译文
关键词
Deep learning,fake news detection,mixed semantic analysis,multiscale transformer
AI 理解论文
溯源树
样例
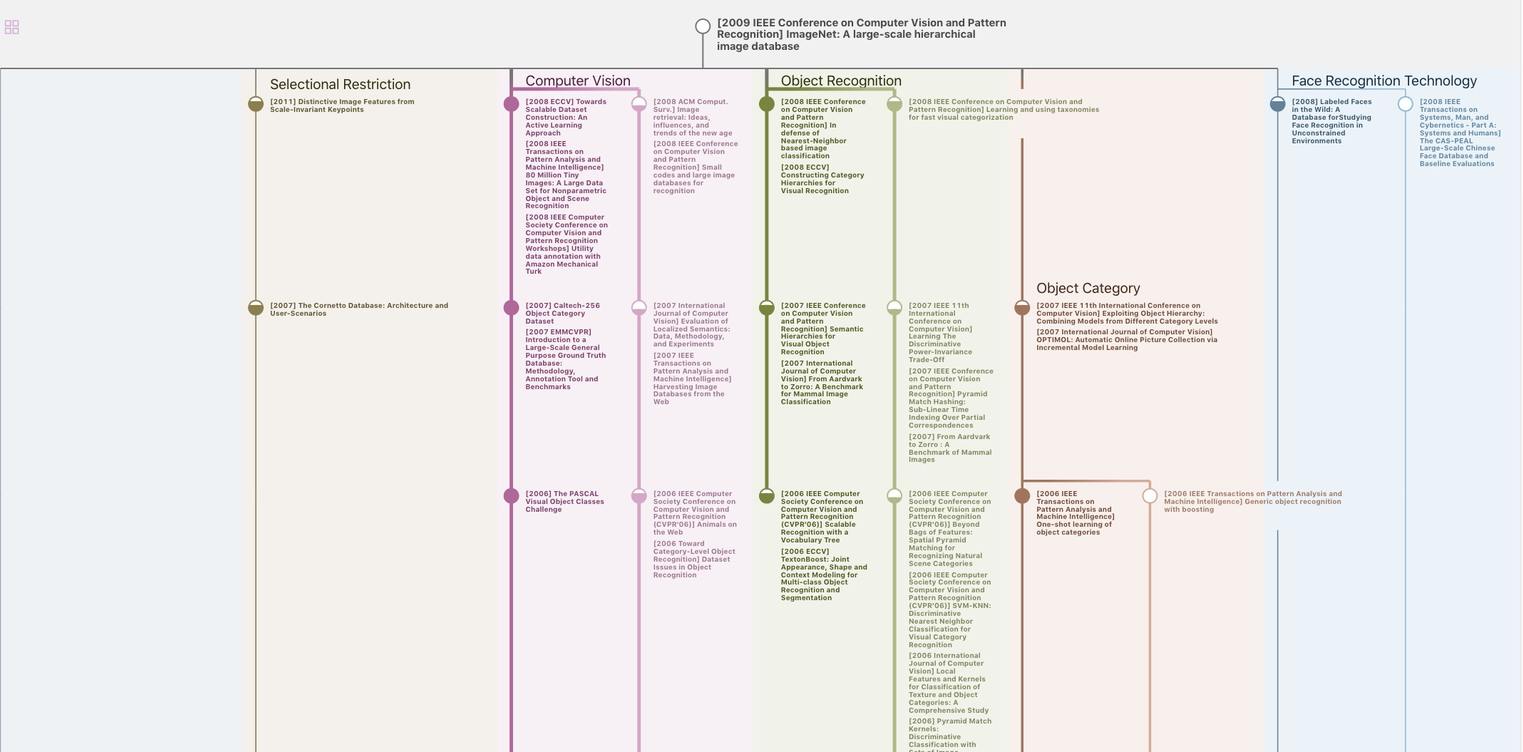
生成溯源树,研究论文发展脉络
Chat Paper
正在生成论文摘要