EGFNet: Edge-Aware Guidance Fusion Network for RGB-Thermal Urban Scene Parsing
IEEE TRANSACTIONS ON INTELLIGENT TRANSPORTATION SYSTEMS(2023)
摘要
Urban scene parsing is the core of the intelligent transportation system, and RGB-thermal urban scene parsing has recently attracted increasing research interest in the field of computer vision. However, most existing approaches fail to perform good boundary extraction for prediction maps and cannot fully use high-level features. In addition, these methods simply fuse the features from RGB and thermal modalities but are unable to obtain comprehensive fused features. To address these problems, an edge-aware guidance fusion network (EGFNet) was developed in this study for RGB-thermal urban scene parsing. First, a prior edge map generated using the RGB and thermal images were introduced to capture detailed information in the prediction map and then embed the prior edge cues into the feature maps. To fuse the RGB and thermal information effectively, a multimodal fusion module was designed that guarantees adequate cross-modal fusion. Considering the importance of high-level semantic information, global and semantic information modules were proposed to extract rich semantic information from the high-level features. For decoding, simple elementwise addition was utilized for cascaded feature fusion. Finally, to improve the parsing accuracy, multitask deep supervision was applied to the semantic and boundary maps. Extensive experiments were performed on benchmark datasets to demonstrate the effectiveness of the proposed EGFNet and its superior performance compared with the state-of-the-art methods.
更多查看译文
关键词
Deep supervision,edge map,high-level information,multimodal fusion,RGB-thermal urban scene parsing
AI 理解论文
溯源树
样例
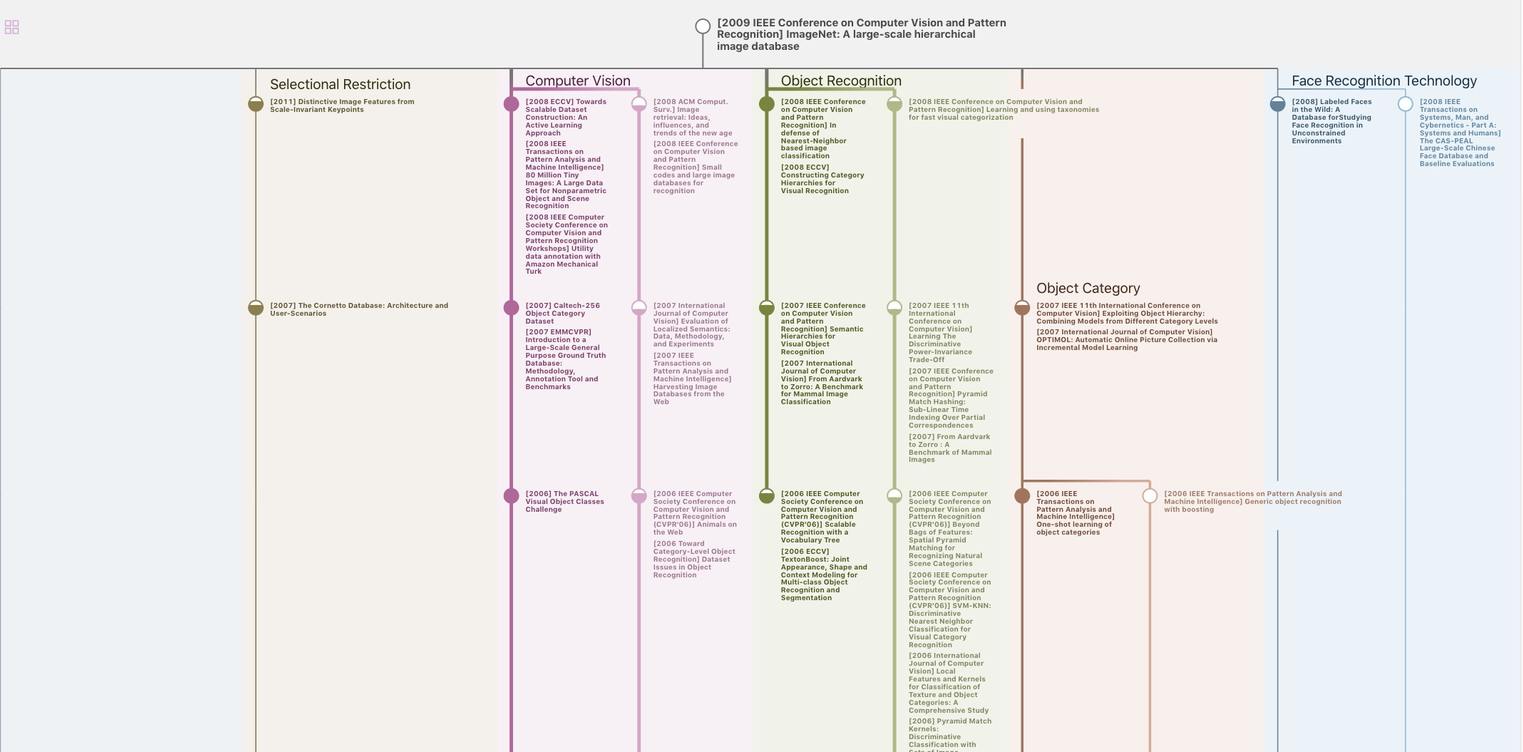
生成溯源树,研究论文发展脉络
Chat Paper
正在生成论文摘要