Dynamic weighted federated remaining useful life prediction approach for rotating machinery
MECHANICAL SYSTEMS AND SIGNAL PROCESSING(2023)
摘要
In actual industrial scenarios, the centralized learning paradigm for remaining useful life (RUL) prediction of rotating machineries usually suffers from several bottlenecks. Firstly, the sufficient life-cycle degradation data is difficult to collect for a single edge client, resulting in a poor prediction performance. Secondly, there is the problem of isolated data island among different users due to the data privacy preservation and commercial rivalry. Hence, it is an urgent challenge to train a prediction model via the decentralized data from multiple clients without touching the raw data. To bridge this gap, a dynamic weighted federated RUL prediction framework is proposed in this paper, which consists of a cloud server and multiple edge clients. Initially, each edge client trains its feature miner locally and uploads the model parameters and features to the server. The server then dynamically weights all the local models to aggregate them into a global one based on the maximum mean discrepancy (MMD) distances of the features between training clients and testing clients. After downloading the integrated feature miner, each client extracts the shallow features from the local dataset, which are then used for training the predictor. Finally, the central server achieves the weighted integration of all local predictors according to the prediction performance of training clients, and then the global model is formed. Experimental results on gear and bearing datasets verify the effectiveness and superiority of the proposed method.
更多查看译文
关键词
Data privacy,RUL prediction,Federated learning,Maximum mean discrepancy,Rotating machinery
AI 理解论文
溯源树
样例
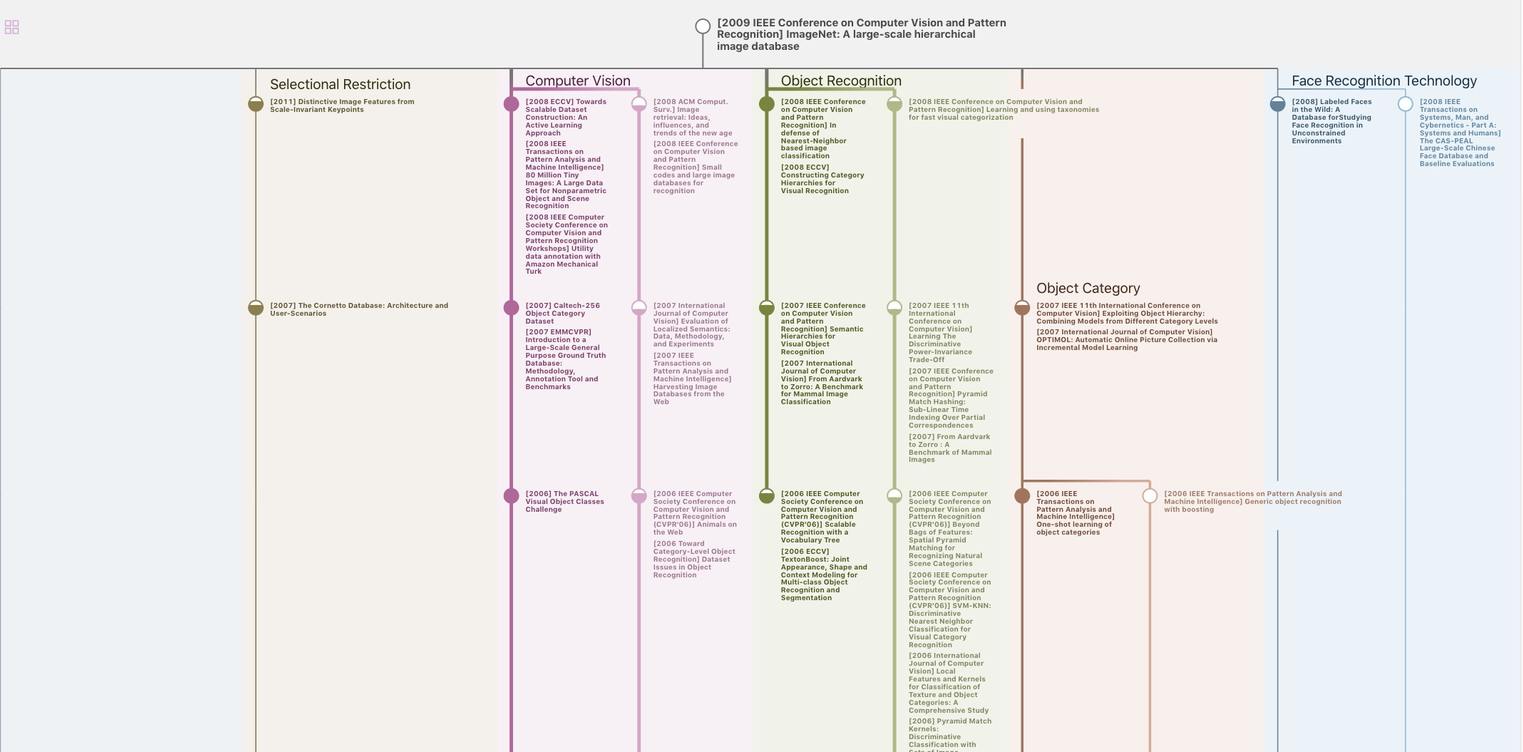
生成溯源树,研究论文发展脉络
Chat Paper
正在生成论文摘要