An intelligent Hybrid-Q Learning clustering approach and resource management within heterogeneous cluster networks based on reinforcement learning
TRANSACTIONS ON EMERGING TELECOMMUNICATIONS TECHNOLOGIES(2023)
摘要
Recently, heterogeneous cluster networks (HCNs) have been the subject of sig-nificant research. The nature of the next-generation HCN environment is decen-tralized and highly dynamic; optimization techniques cannot quite express the dynamic characteristics of node resource utilization and communication of HCN networks. In this article, we present an intelligent Hybrid-Q Learning approach (Hybrid QL)-based clustering approach for IoT and WSN. Using the self-learning abilities of (HCNs), we propose a model for dynamic accessing systems on nodes and agents that identify the best possible paths and com-munication over heterogeneous cluster networks using reinforcement learning. In addition to reducing energy consumption, it creates efficient and effective resource utilization and node communication performance. Through increased throughput and link management, the HCN aims to reduce energy consump-tion. The proposed model is compared to existing approaches based on various scenarios. Finally, the results of the evaluation tasks demonstrate high accu-racy, low-level complexity, fast dynamic response times, and scalability for heterogeneous cluster networks. Our model showed exceptional node allocation efficiency for dynamic IOT environments and WSNs.
更多查看译文
关键词
heterogeneous clustering networks,reinforcement learning,resource management
AI 理解论文
溯源树
样例
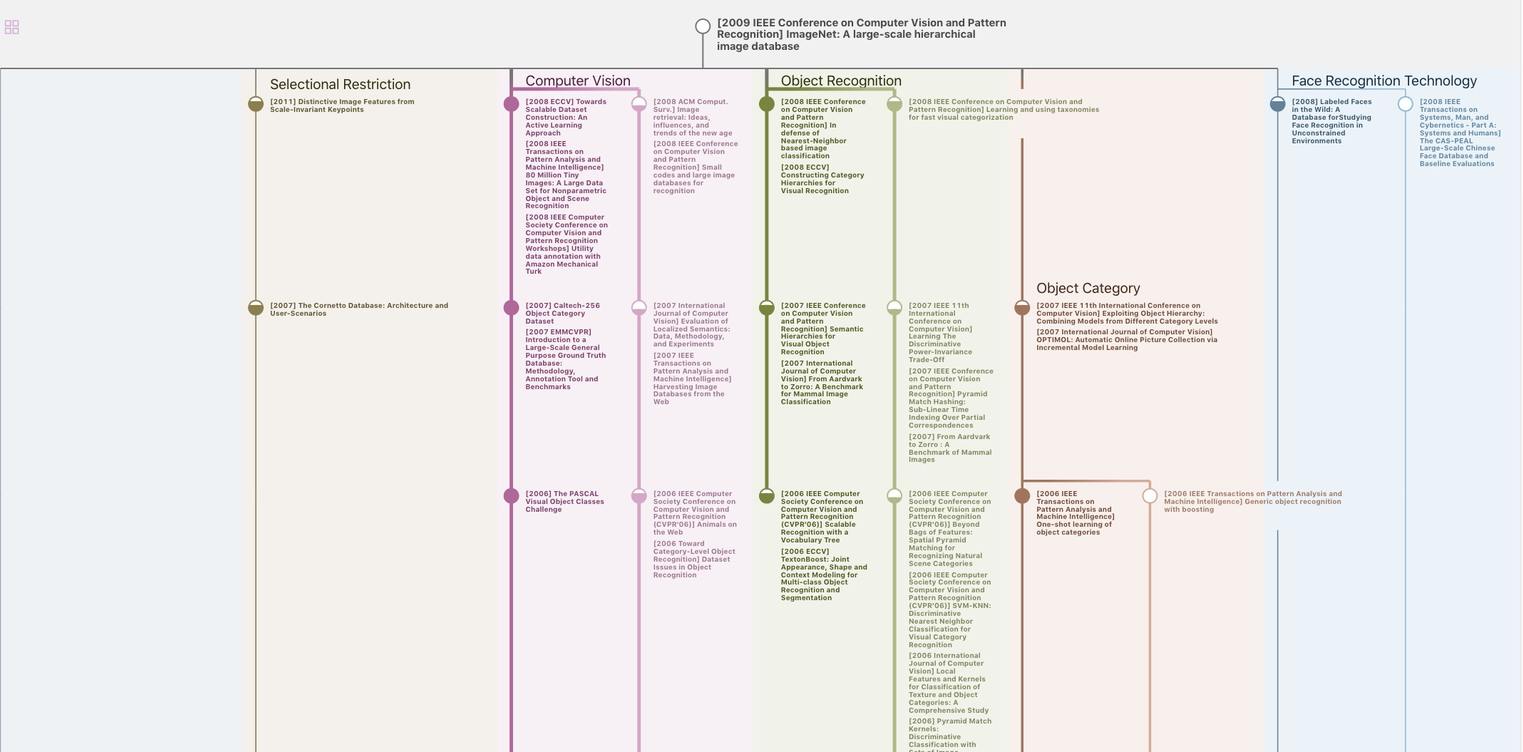
生成溯源树,研究论文发展脉络
Chat Paper
正在生成论文摘要