A robust multi-scale learning network with quasi-hyperbolic momentum-based Adam optimizer for bearing intelligent fault diagnosis under sample imbalance scenarios and strong noise environment
STRUCTURAL HEALTH MONITORING-AN INTERNATIONAL JOURNAL(2024)
摘要
Due to adverse working conditions of rotating machinery in actual engineering, bearing fault data are more difficult to acquire compared to normal data. That said, the real collected bearing vibration data are usually characterized by imbalance. Meanwhile, fault information of the raw collected bearing vibration data is effortlessly drowned out by strong noises, which indicates that it is awkward to efficiently recognize bearing fault states via using traditional fault diagnosis methods under this background. To overcome these problems, this research proposes an individual approach formally intituled as robust multi-scale learning network (RMSLN) with quasi-hyperbolic momentum-based Adam (QHAdam) optimizer for bearing fault diagnosis, which mainly includes convolution-pooling operation, multi-scale branch, and classification layer. Within the proposed method, the channel attention mechanism based on squeezed excitation network is embedded into the multi-scale branch in the form of residual connections, which not only reinforce important information and weaken noise interference, but also capture fault features more comprehensively and enhance the discrimination of fault states with fewer samples. Additionally, in the training process, QHAdam optimizer is introduced to tightly control the loss of RMSLN to enable a faster and smoother convergence. Two groups of experimental bearing data are studied to support the availability of presented approach, and several traditional fault diagnosis methods and representative imbalance fault diagnosis approaches are compared in four evaluation metrics (accuracy, macro-precision, macro-recall, and macro-F1 score) to highlight the advantages of the presented method.
更多查看译文
关键词
Robust multi-scale learning network,QHAdam optimizer,rolling bearing,fault diagnosis
AI 理解论文
溯源树
样例
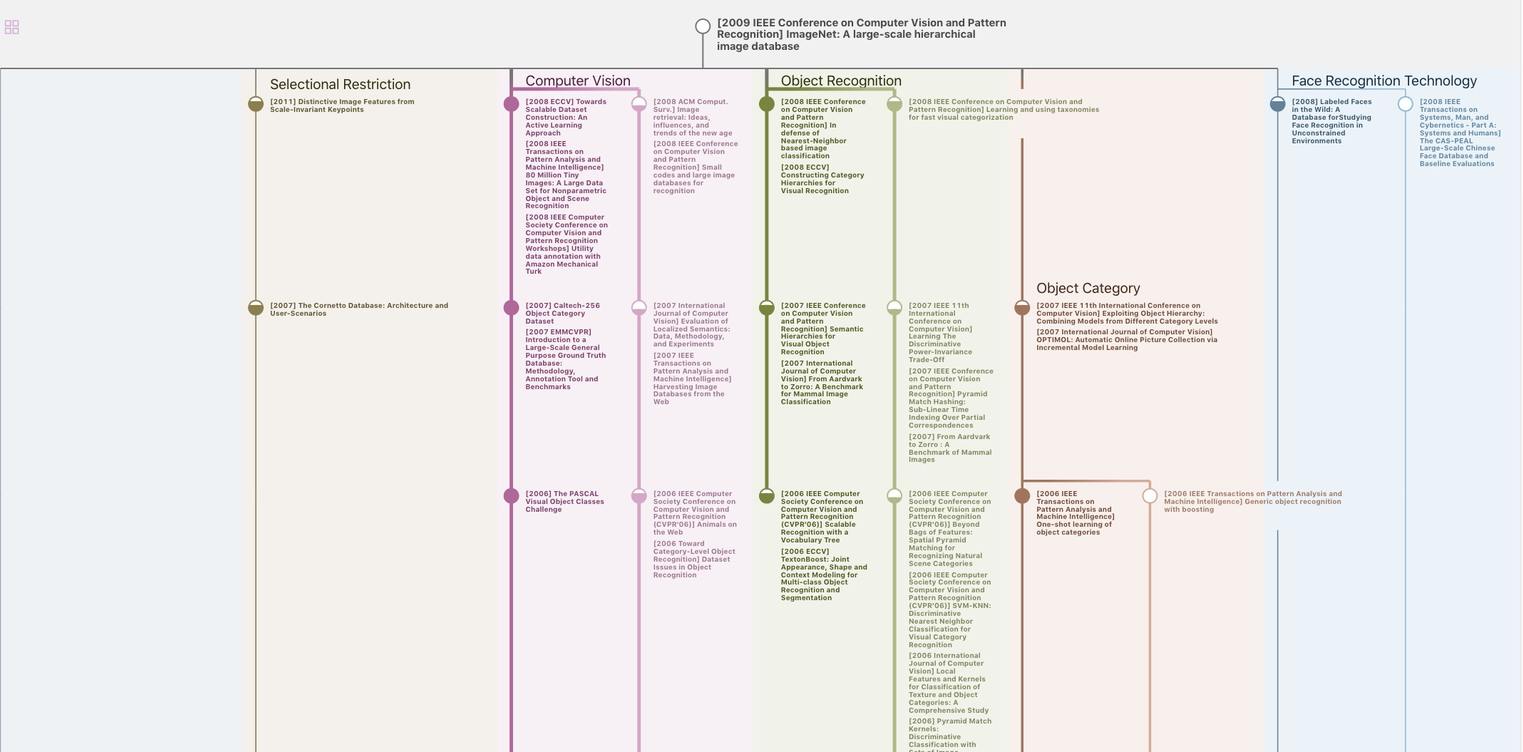
生成溯源树,研究论文发展脉络
Chat Paper
正在生成论文摘要