A robust twin support vector machine based on fuzzy systems
INTERNATIONAL JOURNAL OF INTELLIGENT COMPUTING AND CYBERNETICS(2024)
摘要
PurposeTwin support vector machine (TSVM) is an effective machine learning technique. However, the TSVM model does not consider the influence of different data samples on the optimal hyperplane, which results in its sensitivity to noise. To solve this problem, this study proposes a twin support vector machine model based on fuzzy systems (FSTSVM).Design/methodology/approachThis study designs an effective fuzzy membership assignment strategy based on fuzzy systems. It describes the relationship between the three inputs and the fuzzy membership of the sample by defining fuzzy inference rules and then exports the fuzzy membership of the sample. Combining this strategy with TSVM, the FSTSVM is proposed. Moreover, to speed up the model training, this study employs a coordinate descent strategy with shrinking by active set. To evaluate the performance of FSTSVM, this study conducts experiments designed on artificial data sets and UCI data sets.FindingsThe experimental results affirm the effectiveness of FSTSVM in addressing binary classification problems with noise, demonstrating its superior robustness and generalization performance compared to existing learning models. This can be attributed to the proposed fuzzy membership assignment strategy based on fuzzy systems, which effectively mitigates the adverse effects of noise.Originality/valueThis study designs a fuzzy membership assignment strategy based on fuzzy systems that effectively reduces the negative impact caused by noise and then proposes the noise-robust FSTSVM model. Moreover, the model employs a coordinate descent strategy with shrinking by active set to accelerate the training speed of the model.
更多查看译文
关键词
Fuzzy system,Membership function,Twin support vector machine,Pattern classification
AI 理解论文
溯源树
样例
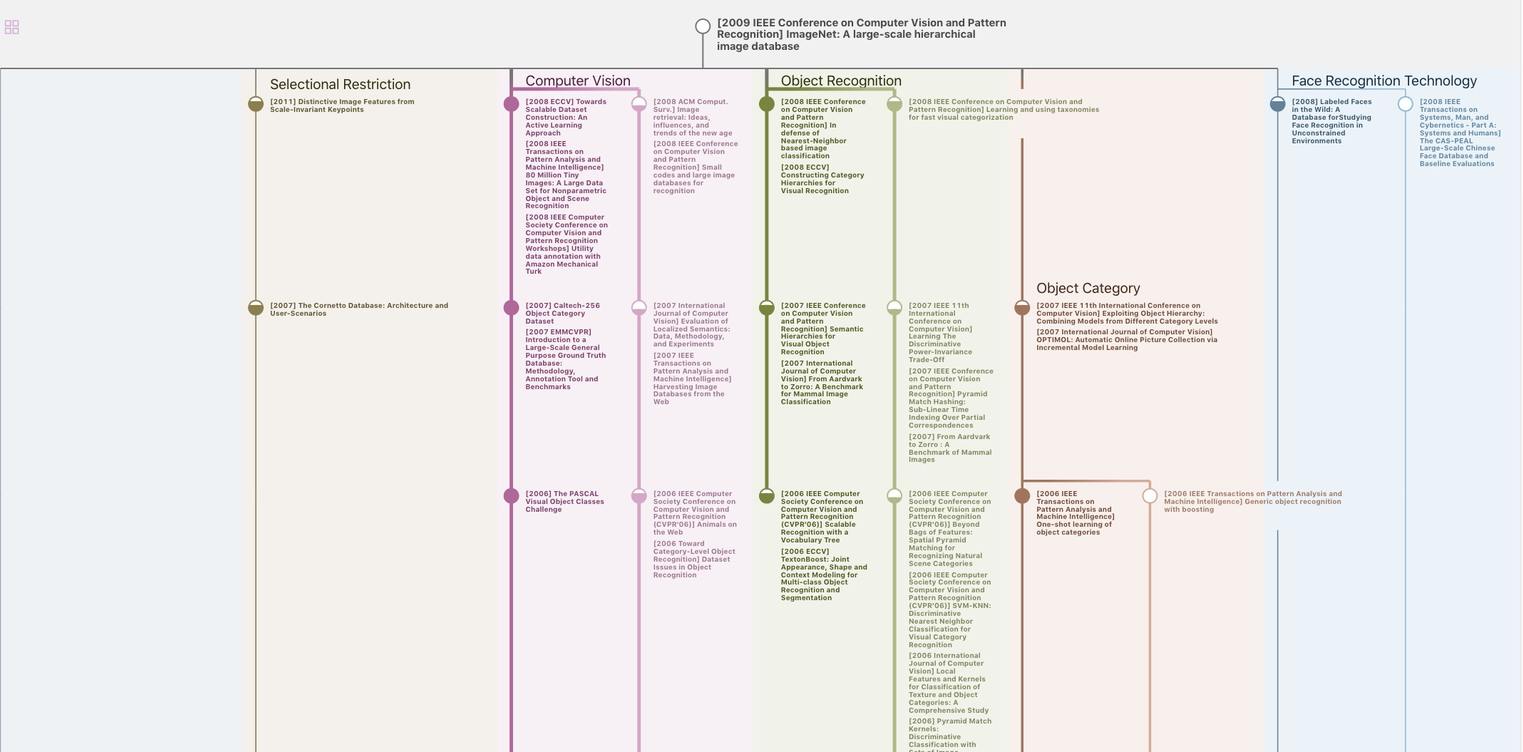
生成溯源树,研究论文发展脉络
Chat Paper
正在生成论文摘要