Data representation learning via dictionary learning and self-representation
APPLIED INTELLIGENCE(2023)
摘要
Dictionary learning is an effective feature learning method, leading to many remarkable results in data representation and classification tasks. However, dictionary learning is performed on the original data representation. In some cases, the capability of representation and discriminability of the learned dictionaries may need to be performed better, i.e., with only sparse but not low rank. In this paper, we propose a novel efficient data representation learning method by combining dictionary learning and self-representation, which utilizes both properties of sparsity in dictionary learning and low-rank in low-rank representation (LRR) simultaneously. Thus both the sparse and low-rank properties of the data representation can be naturally captured by our method. To obtain the solution of our proposed method effectively, we also innovatively introduce a more generalized data representation model in this paper. To our best knowledge, its closed-form solution is first derived analytically through our rigorous mathematical analysis. Experimental results show that our method not only can be used for data pre-processing but also can realize better dictionary learning. The samples in the same class can have similar representations by our method, and the discriminability of the learned dictionary can also be enhanced.
更多查看译文
关键词
Dictionary learning,Low-rank representation,Data representation,Self-representation
AI 理解论文
溯源树
样例
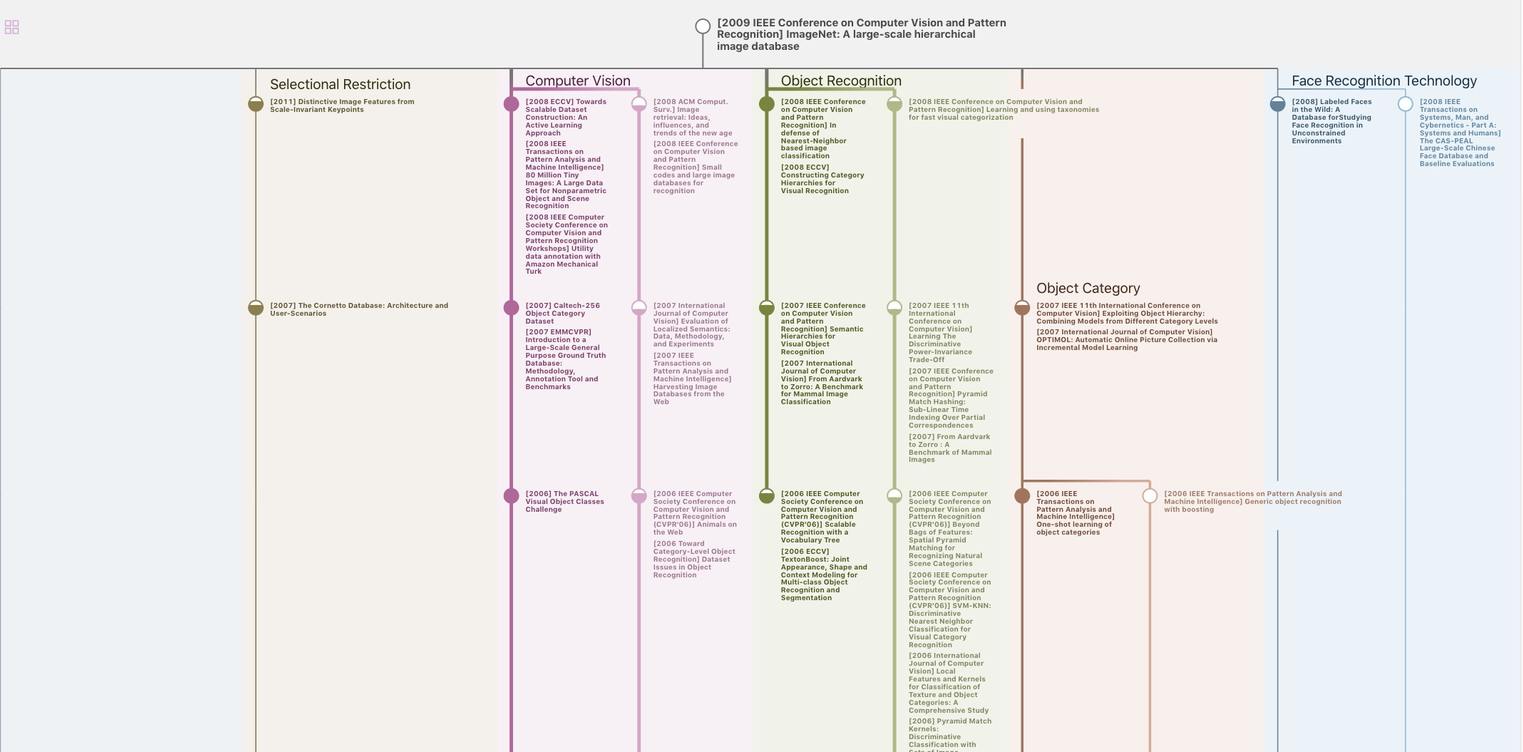
生成溯源树,研究论文发展脉络
Chat Paper
正在生成论文摘要