A Flexible Spatiotemporal Thick Cloud Removal Method with Low Requirements for Reference Images
REMOTE SENSING(2023)
摘要
Thick cloud and shadows have a significant impact on the availability of optical remote sensing data. Although various methods have been proposed to address this issue, they still have some limitations. First, most approaches rely on a single clear reference image as complementary information, which becomes challenging when the target image has large missing areas. Secondly, the existing methods that can utilize multiple reference images require the complementary data to have high temporal correlation, which is not suitable for situations where the difference between the reference image and the target image is large. To overcome these limitations, a flexible spatiotemporal deep learning framework based on generative adversarial networks is proposed for thick cloud removal, which allows for the use of three arbitrary temporal images as references. The framework incorporates a three-step encoder that can leverage the uncontaminated information from the target image to assimilate the reference images, enhancing the model's ability to handle reference images with diverse temporal differences. A series of simulated and real experiments on Landsat 8 and Sentinel 2 data is performed to demonstrate the effectiveness of the proposed method. The proposed method is especially applicable to small/large-scale regions with reference images that are significantly different from the target image.
更多查看译文
关键词
thick cloud removal, spatiotemporal deep learning framework, generative adversarial network, arbitrary temporal images
AI 理解论文
溯源树
样例
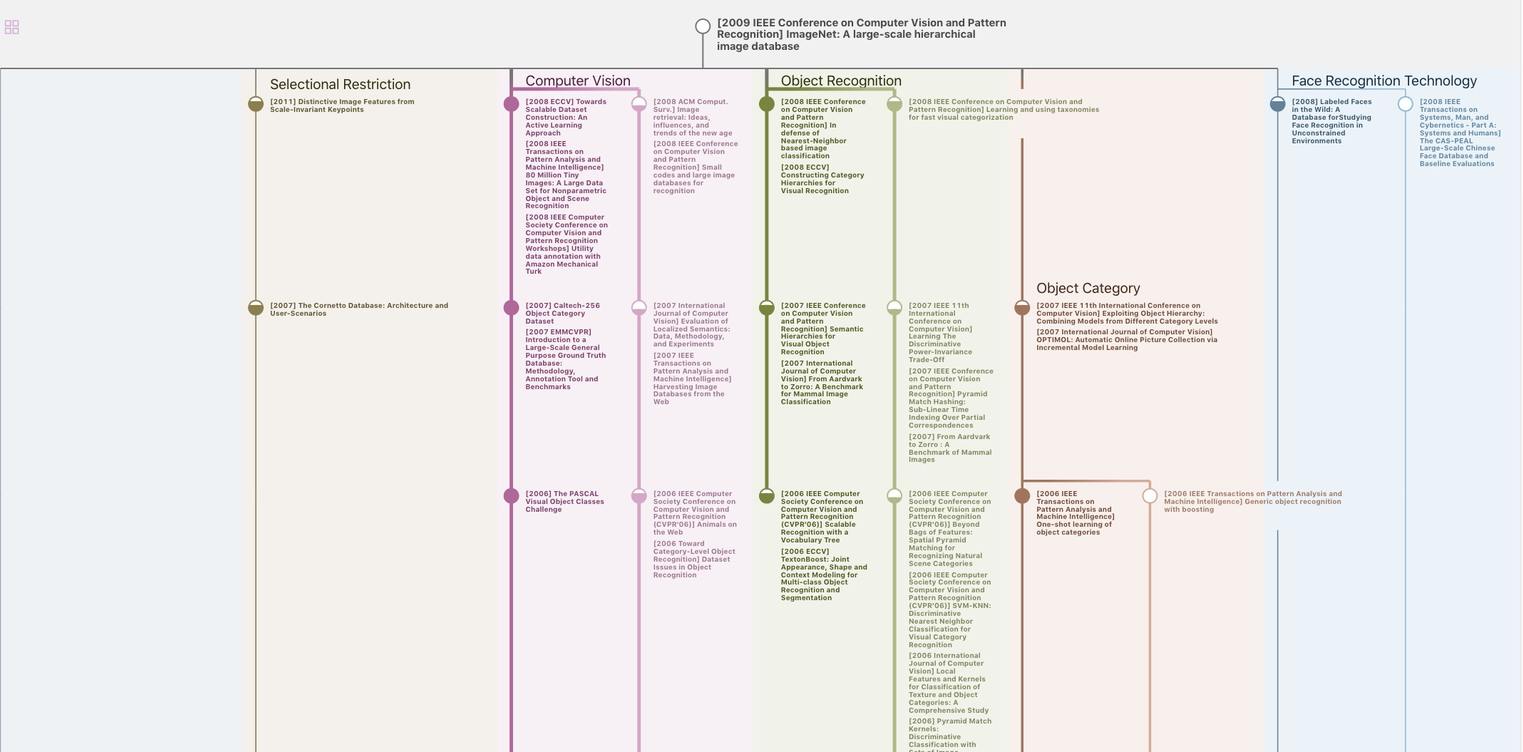
生成溯源树,研究论文发展脉络
Chat Paper
正在生成论文摘要