Employing ANN for daylight and energy prediction of hot climate office buildings: a case study of new Cairo, Egypt
ARCHITECTURAL ENGINEERING AND DESIGN MANAGEMENT(2023)
摘要
Indoor lighting conditions are significantly impacted by solar shading. It may be extremely difficult, time-consuming, and expensive to use solar energy efficiently, especially in hot climates. In this paper, the artificial neural network (ANN) is used to forecast and analyze daylight and energy intensity usage for electrical lighting and cooling loads in the early design stages of office buildings. This research will investigate the effect of using different fixed shading systems, including fins, inside louvers, and outside louvers, on an office building in New Cairo, Egypt. There are three steps in the framework of the analysis. Initially, a field experiment is conducted for validation. Then, 3700 simulations on Honeybee for Grasshopper are used to generate a dataset for training, validation, and testing of the ANN model at the third step using the MATLAB & REG; machine learning toolbox. The performance of the ANN model is evaluated using the R-Squared and mean-squared error (MSE) metrics. The resulting ANN models are 446 times quicker than the simulation software and have R-Squared values of 1, 1, 0.98, and 0.983 for Energy Use Intensity (EUI), Daylight Autonomy (DA), Continuous Daylight Autonomy (CDA), and Useful Daylight Illuminance (UDI), respectively. The results showed that adopting different shading systems improves the distribution of lighting inside the space, increases the UDI by 27%, and decreases cooling loads by 27%. The partial effect of each shading design parameter on UDI and EUI is investigated, and then suggested values of shading design parameters are offered.
更多查看译文
关键词
hot climate office buildings,daylight,energy prediction
AI 理解论文
溯源树
样例
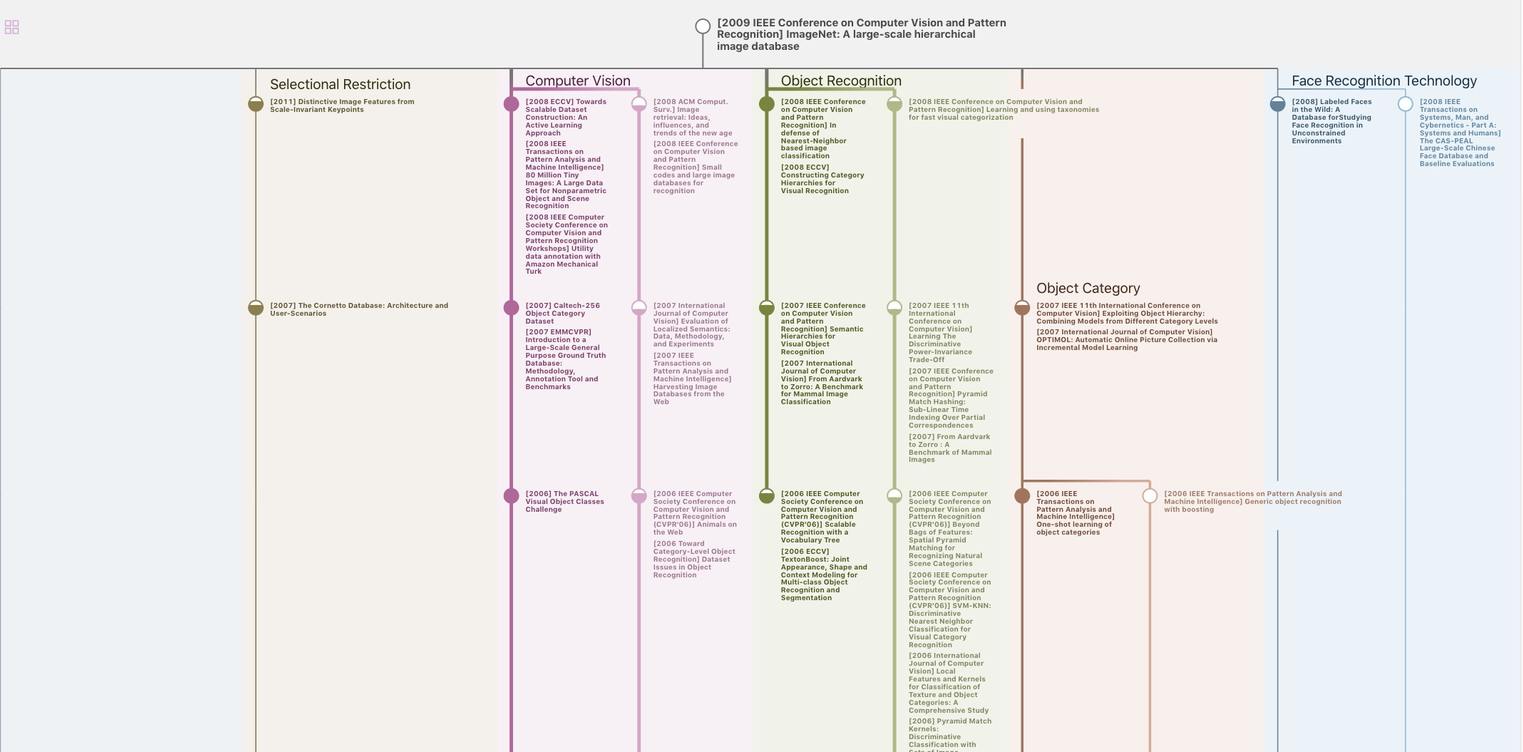
生成溯源树,研究论文发展脉络
Chat Paper
正在生成论文摘要