Uncertainty Decomposition to Understand the Influence of Water Systems Model Error in Climate Vulnerability Assessments
WATER RESOURCES RESEARCH(2023)
摘要
Climate vulnerability assessments rely on water infrastructure system models that imperfectly predict performance metrics under ensembles of future scenarios. There is a benefit to reduced complexity system representations to support these assessments, especially when large ensembles are used to better characterize future uncertainties. An important question is whether the total uncertainty in the output metrics is primarily attributable to the climate ensemble or to the systems model itself. Here we develop a method to address this question by combining time series error models of performance metrics with time-varying Sobol sensitivity analysis. The method is applied to a reduced complexity multi-reservoir systems model of the Sacramento-San Joaquin River Basin in California to demonstrate the decomposition of flood risk and water supply uncertainties under an ensemble of climate change scenarios. The results show that the contribution of systems model error to total uncertainty is small (& SIM;5%-15%) relative to climate based uncertainties. This indicates that the reduced complexity systems model is sufficiently accurate for use in the context of the vulnerability assessment. We also observe that climate uncertainty is dominated by the choice of general circulation model and its interactive effects with the representative concentration pathway (RCP), rather than the RCP alone. This observation has implications for how climate vulnerabilities should be interpreted.
更多查看译文
关键词
climate vulnerability assessment, uncertainty, variance decomposition, systems model, fit-for-purpose
AI 理解论文
溯源树
样例
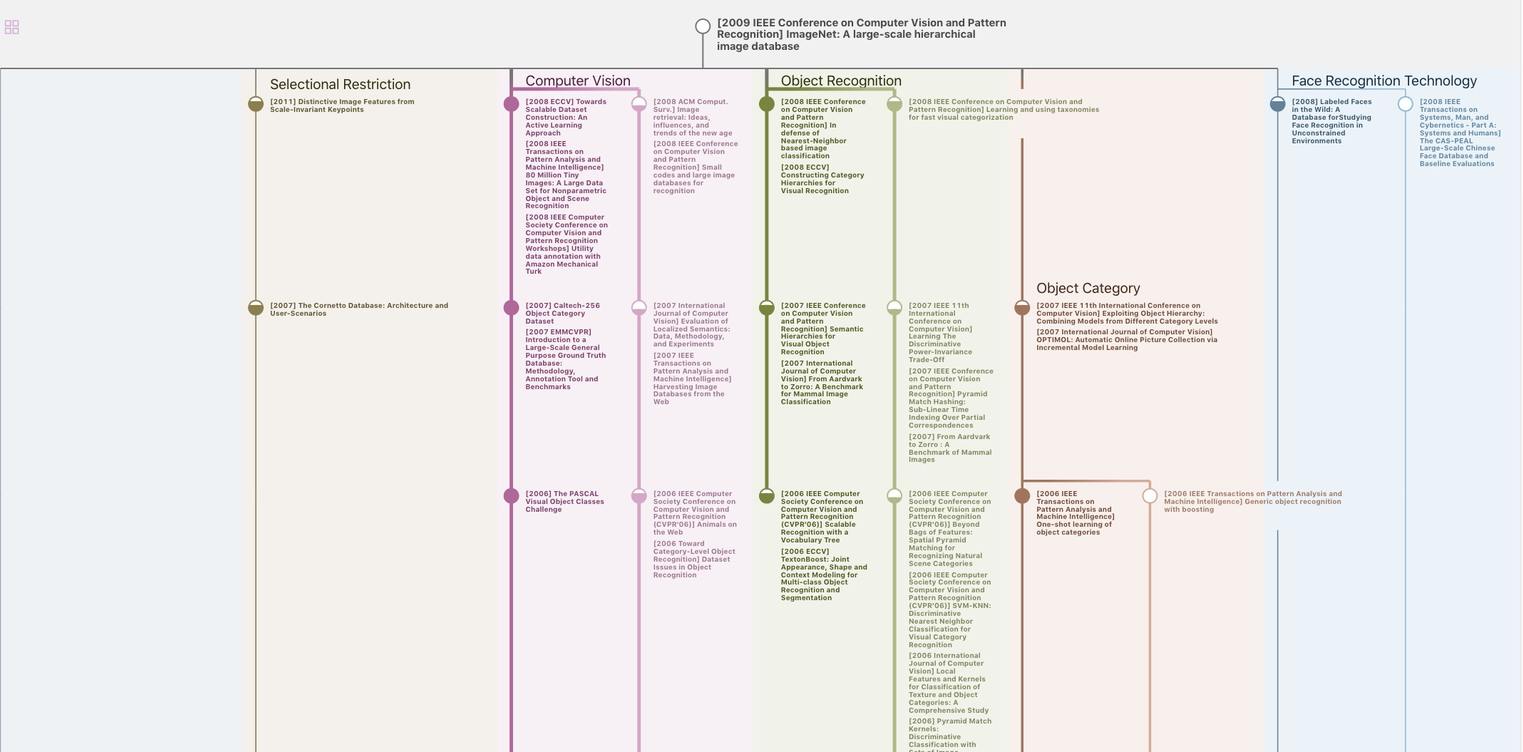
生成溯源树,研究论文发展脉络
Chat Paper
正在生成论文摘要