Optimized Embedded Model for Time Sequence Alignment within Distributed Video Streams Environment
2023 3RD INTERNATIONAL CONFERENCE ON INFORMATION COMMUNICATION AND SOFTWARE ENGINEERING, ICICSE(2023)
摘要
When the same action is captured from different perspectives, different video sequences will be recorded. For video alignment in a distributed video stream environment, learning the visual invariance features of different frames from different viewpoints is affected by the change of foreground and background, which makes learning much more difficult. Note that, the 2D human pose sequences extracted from multiple consecutive images of the video contain the main behavioral information of the person in the video. To reduce the difficulty of model learning, we explore learning visual invariance features from 2D human pose sequences in different video streams. We map the 2D human pose sequences into the embedding space, and the Euclidean distance in the embedding space quantifies the similarity between different frame sequences. Experimental results show that: (i) In the alignment task of distributed video streams, compared to methods that directly learn the similarity between video image sequences, our approach achieves better alignment results with a smaller model. (ii) In the multi-view human pose retrieval task, compared with 3D pose estimation models, our embedding model achieves higher accuracy when retrieving 2D poses projected from the same 3D pose across different camera views. (iii) We exploit the similarity between consecutive multiple frames and treat it as a classification task, which allows our model to learn visual invariance features with higher resolution.
更多查看译文
关键词
distribute video streams,video alignment,2D human pose sequence,visual invariance features
AI 理解论文
溯源树
样例
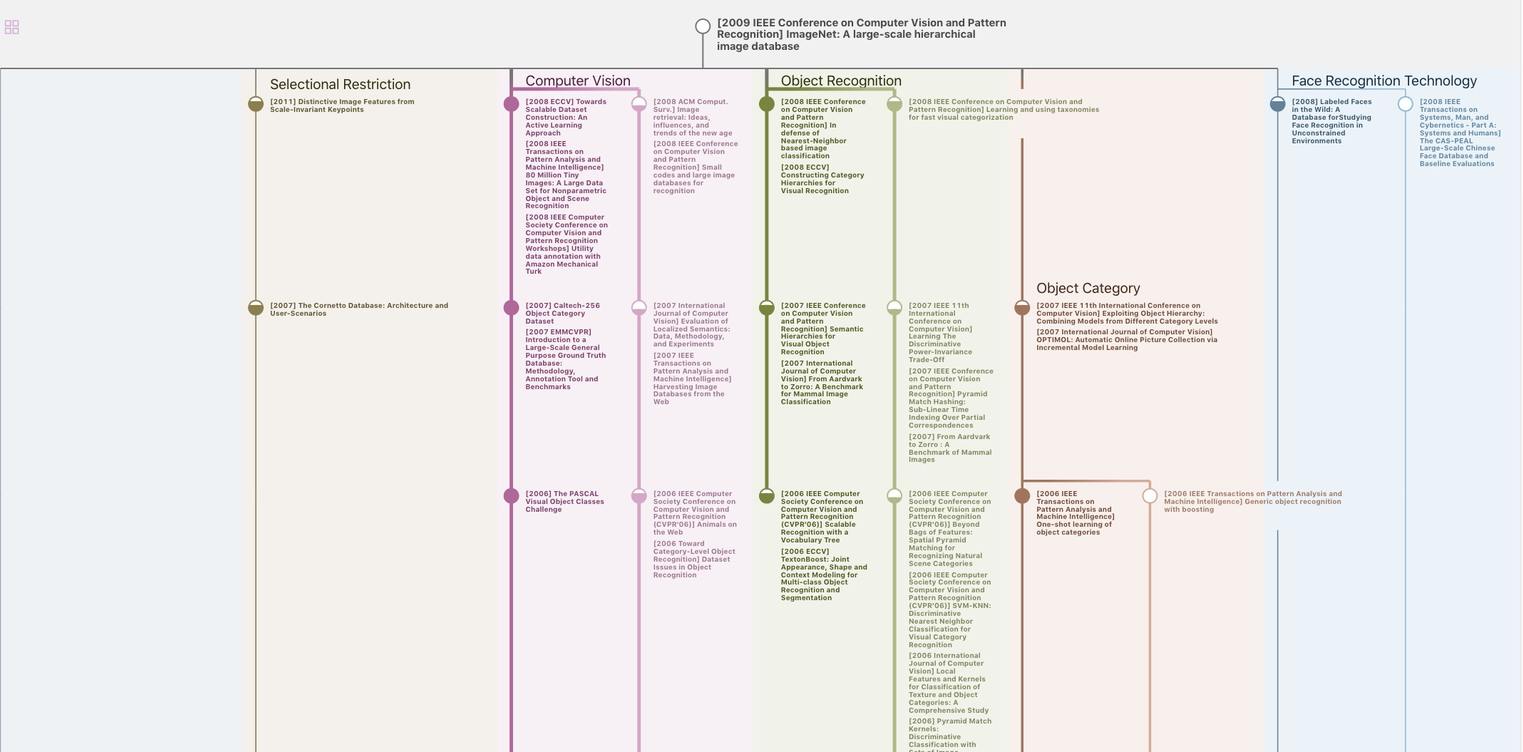
生成溯源树,研究论文发展脉络
Chat Paper
正在生成论文摘要