Model-Based Leakage Detection for Large-Scale Water Pipeline Networks
PIPELINES 2023: CONDITION ASSESSMENT, UTILITY ENGINEERING, SURVEYING, AND MULTIDISCIPLINE(2023)
摘要
A significant amount of treated drinking water is lost through leakage in water distribution systems across the globe. Conventional leakage detection techniques are ad-hoc in nature and expensive for system-wide leakage detection and severity assessment. Advanced metering infrastructure (AMI) and cyber monitoring technologies enable the collection of valuable data that could reveal pipeline conditions. This paper presents a model-based technique that leverages AMI consumption data and pressure data from large-scale distribution networks to infer leakage presence and approximate severity. Pipe roughness, effective hydraulic diameters, and leak presence and severity are considered as the unknown dynamic parameters in this study based on a Monte Carlo engine in MATLAB. For inferring the leakage presence, pipe roughness and effective hydraulic diameters are considered as stochastic variables following a certain probabilistic distribution. A neural network model is developed to predict emitter coefficients as leakage surrogate measures for all the pipelines in the distribution system's considered pressure zone based on consumption data derived from the AMI meters and pressure data derived from the entire distribution system. The approach is demonstrated on a large-scale (>1,000 pipelines) water distribution network to evaluate the computational challenges along with the potential solutions. This study could benefit water utilities that have already installed or plan to install AMI meters.
更多查看译文
AI 理解论文
溯源树
样例
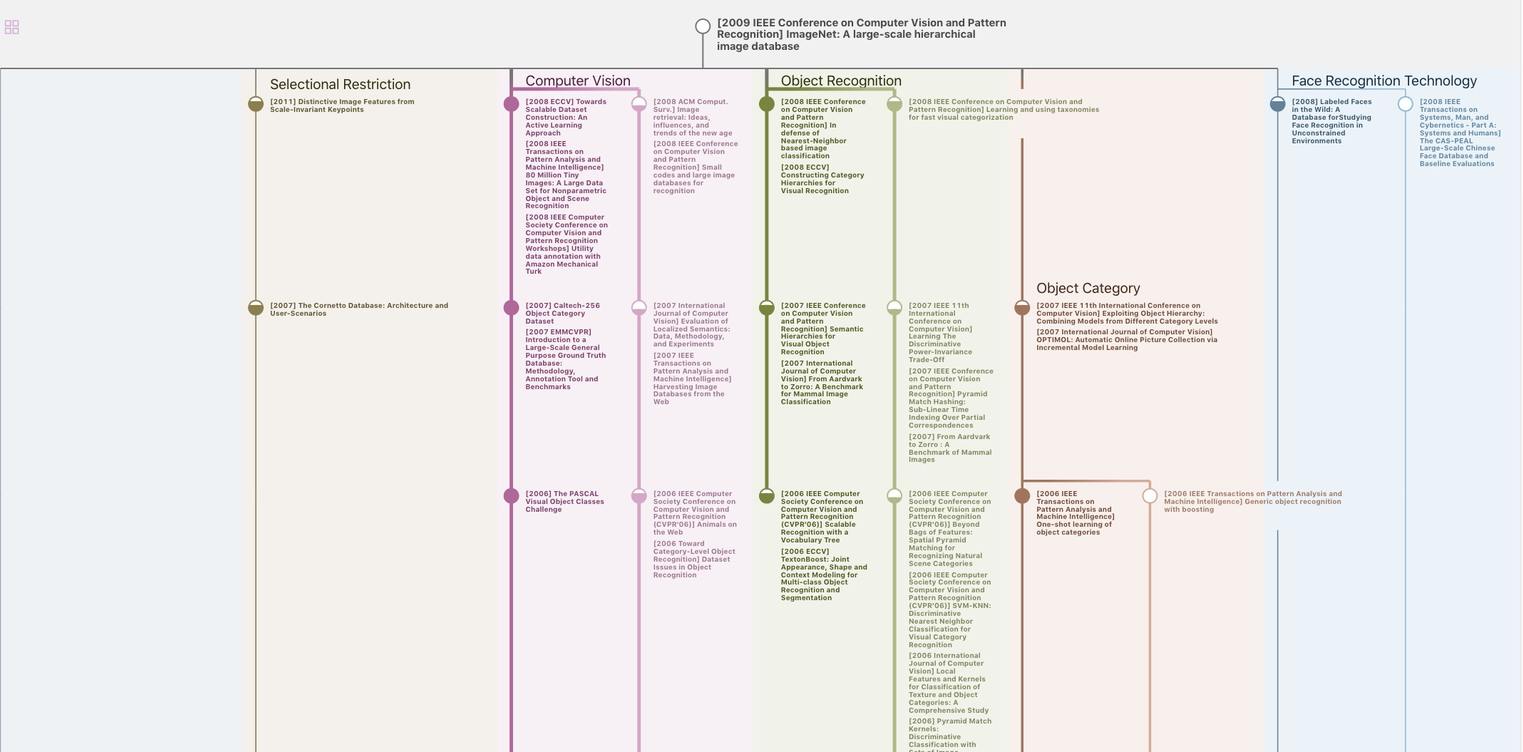
生成溯源树,研究论文发展脉络
Chat Paper
正在生成论文摘要