Investigation of KNN and Decision Tree Methods' Efficiency in Developing Prediction Models for Sewer Pipes
PIPELINES 2023: CONDITION ASSESSMENT, UTILITY ENGINEERING, SURVEYING, AND MULTIDISCIPLINE(2023)
摘要
Wastewater collection systems become less effective over time, necessitating ongoing changes and the creation of asset management frameworks on the side of utility owners to keep their assets operating as intended. Since inspecting every sewer pipe is time-consuming and costly, developing prediction models that can anticipate sewer pipes' current and future state is vital. In this research, two machine learning methods including k-nearest neighbors (KNN) and decision tree have been investigated in order to create a prediction model for sewer pipes of two cities in USA. The data which is used is a combined data from Tampa, Florida, and Dallas, Texas, containing nine physical and environmental variables. F1-score and area under the curve (AUC) were calculated to compare the effectiveness of developed models. It was concluded that the decision tree model performed better than the other. The overall F1-score for the decision tree model was 0.73 and for KNN was 0.70.
更多查看译文
AI 理解论文
溯源树
样例
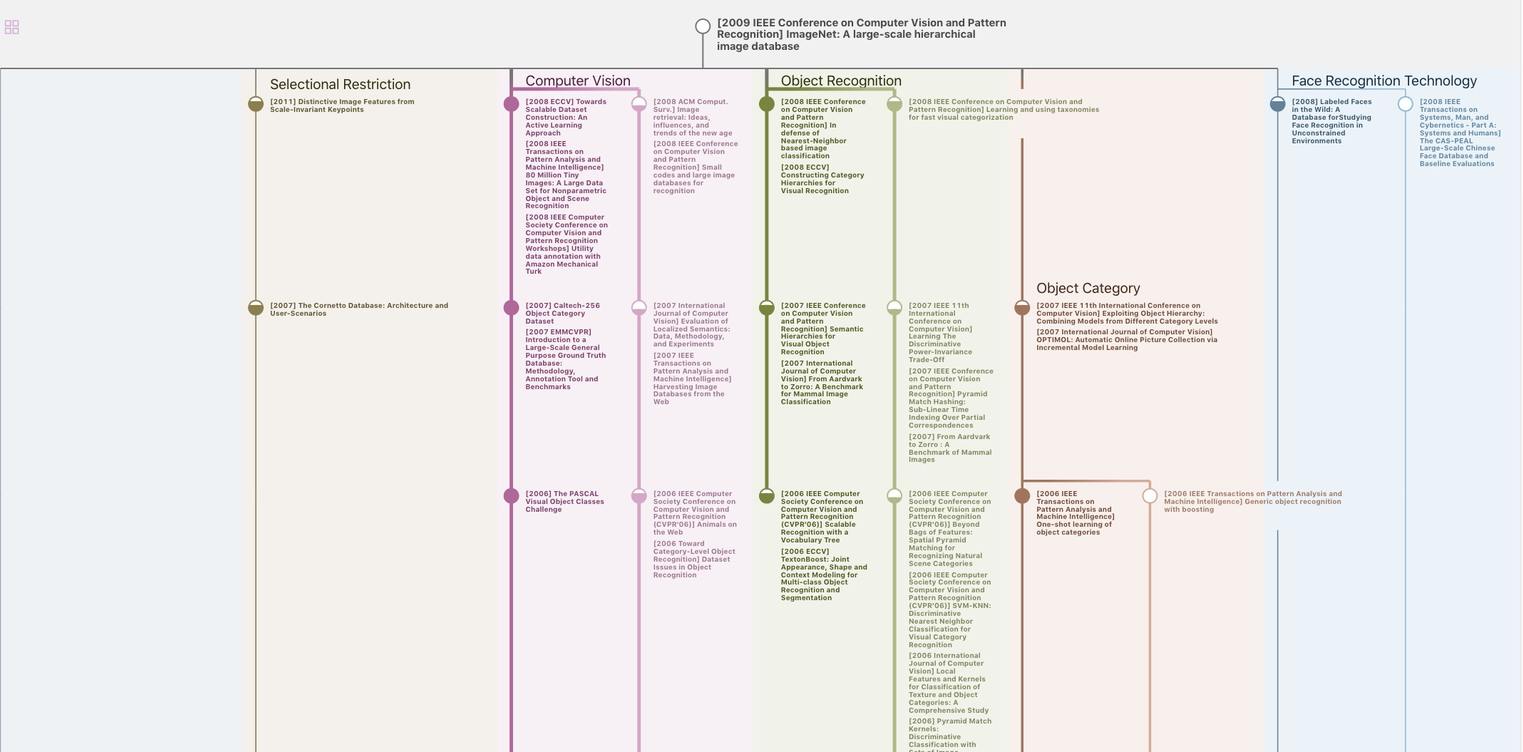
生成溯源树,研究论文发展脉络
Chat Paper
正在生成论文摘要