Surface and Underwater Acoustic Source Recognition Using Multi-Channel Joint Detection Method Based on Machine Learning
JOURNAL OF MARINE SCIENCE AND ENGINEERING(2023)
摘要
Sound source recognition is a very important application of passive sonar. How to distinguish between surface and underwater acoustic sources has always been a challenge. Due to the mixing of underwater target radiated noise and marine environmental noise, especially in shallow water environments where multipath effects exist, it is difficult to distinguish them. To solve the surface and underwater acoustic source recognition problem, this paper proposes a multi-channel joint detection method based on machine learning. First, the simulation data are generated using the normal model KRAKEN setting in the same environment as the SACLANT 1993 experiment, which uses a vertical linear array of 48 hydrophones. Secondly, the GBDT classifier and LightGBM classifier are trained separately, and then the model is evaluated using precision, recall, F1, and accuracy. Finally, four ML models (kNN, random subspace kNN, GBDT, and LightGBM) are used to analyze all 48 channels of hydrophone data. For each model, two kinds of feature extraction methods (module features, real and imaginary features) are applied. Generally, the results show that both GBDT and LightGBM models have better performance than both kNN and random subspace kNN ones. For both GBDT and LightGBM models, the results using module features have better performance than using real and imaginary features.
更多查看译文
关键词
surface and underwater acoustic source recognition, machine learning, GBDT, LightGBM, multi-channel joint detection
AI 理解论文
溯源树
样例
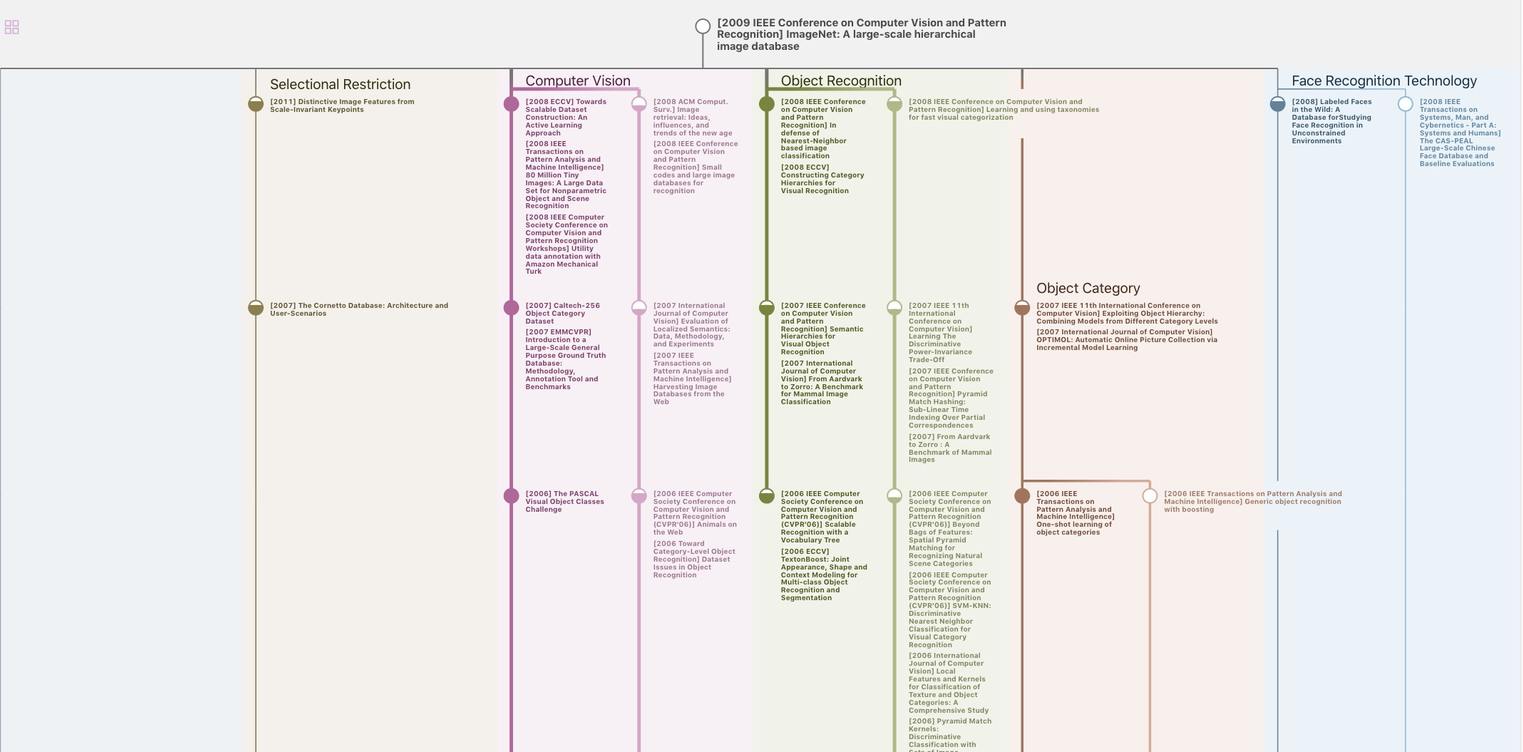
生成溯源树,研究论文发展脉络
Chat Paper
正在生成论文摘要