A hybrid enhanced attention transformer network for medical ultrasound image segmentation
BIOMEDICAL SIGNAL PROCESSING AND CONTROL(2023)
摘要
Ultrasound (US) technology is a widely utilized for clinical screening owing to its cost-effectiveness, painlessness, and convenience. However, the automatic segmentation of lesions or organs in US images remains to be challenging due to speckle artifacts, blurred boundaries, and low contrast. Recently, transformer-based methods have shown to be effective in long-range dependency, which is a good complement to Convolutional Neural Networks (CNN). In this article, we present a novel U-shape segmentation model based on a hybrid CNN-transformer structure that can effectively integrate CNN local features and transformer long-range contextual information of US images. To begin with, we design a coordinate residual block (CdRB) to encode the absolute position information of lesions. Further, we develop a channel enhanced self-attention-based transformer (ECAT) to help enhance the response of extracted global features. Finally, we adopt a comprehensive dual attention module (CDAM) to enhance skip connection features, which can learn feature correlations and capture more accurate edge features in US images. Results based on four public US datasets demonstrate that our method outperforms state-of-the-art segmentation methods, with 0.741 Dice on BUSI for breast lesion segmentation, 0.827 Dice on DDTI for thyroid lesion segmentation, 0.895 Dice on TN3k for thyroid lesion segmentation and 0.940 Dice on CAMUS for left ventricle segmentation. Furthermore, the robustness of our network is further demonstrated by an external validation dataset for breast lesion segmentation. In summary, our method showcases excellent adaptability and robustness in US image segmentation and can potentially be a general US segmentation tool.
更多查看译文
关键词
Ultrasound image segmentation,Convolutional neural network,Transformer,Attention modules
AI 理解论文
溯源树
样例
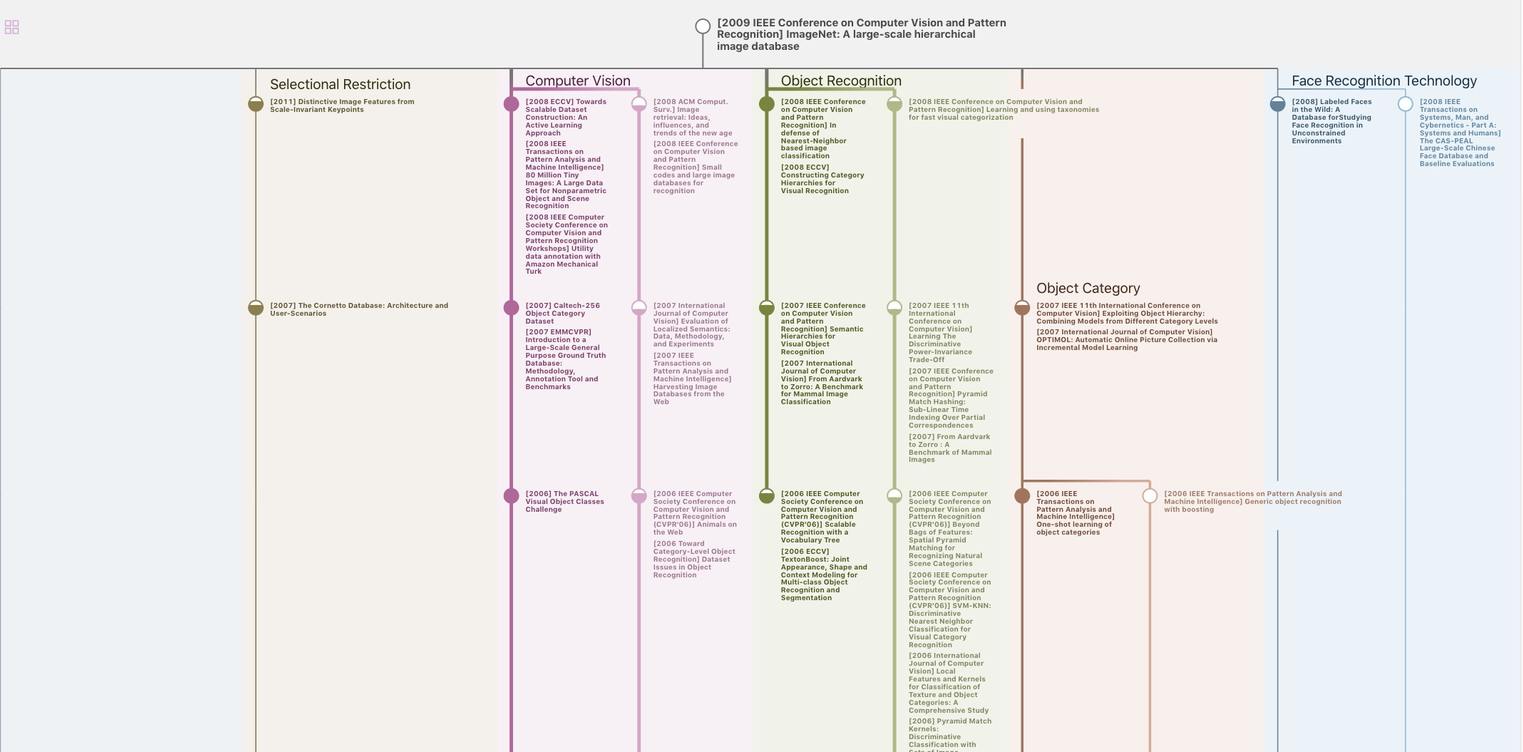
生成溯源树,研究论文发展脉络
Chat Paper
正在生成论文摘要