Optimizing Odometry Accuracy through Temporal Information in Self-Supervised Deep Networks
2023 20TH INTERNATIONAL CONFERENCE ON UBIQUITOUS ROBOTS, UR(2023)
摘要
In this paper, we propose a self-supervised deep recurrent convolutional network with a self-attention pipeline for LiDAR-Inertial odometry estimation that leverages temporal information. In contrast to existing learning-based approaches, our network does not require expensive ground truth and it utilizes sliding window optimization for self-supervisory signal for training. The results indicate that the proposed network is able to achieve accuracy levels comparable to conventional model-based methods and outperform other learning-based approaches that do not incorporate temporal information. Our findings provide strong evidence for the potential of incorporating temporal information in deep networks for LiDAR-Inertial odometry and highlight the effectiveness of our proposed selfsupervised approach.
更多查看译文
关键词
accuracy levels,conventional model-based methods,existing learning-based approaches,expensive ground truth,LiDAR-Inertial odometry estimation,odometry accuracy,self-supervised approach,self-supervised deep networks,self-supervised deep recurrent convolutional network,temporal information,window optimization
AI 理解论文
溯源树
样例
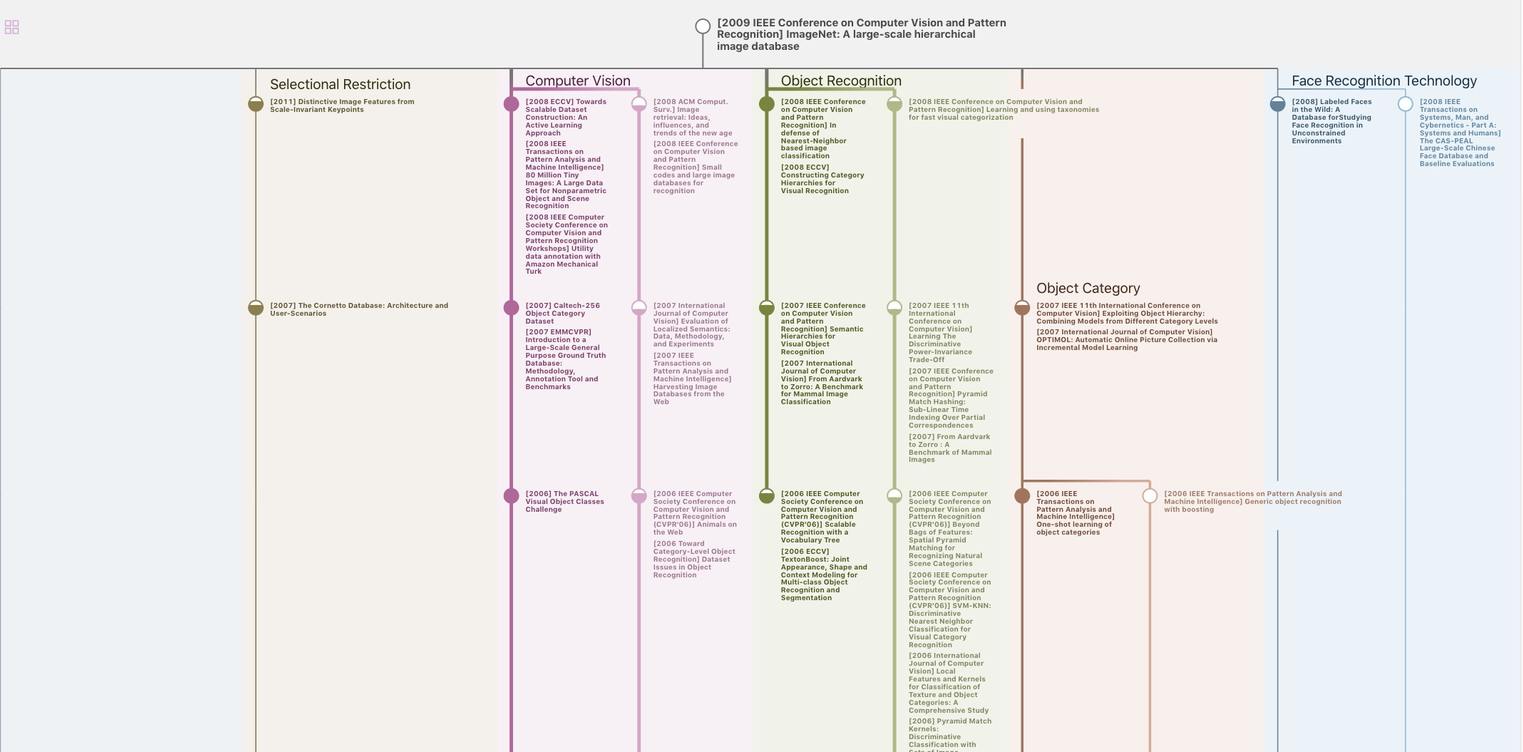
生成溯源树,研究论文发展脉络
Chat Paper
正在生成论文摘要