Condition Prediction of Sanitary Sewer Pipe Data Set with Imbalanced Classification
PIPELINES 2023: CONDITION ASSESSMENT, UTILITY ENGINEERING, SURVEYING, AND MULTIDISCIPLINE(2023)
摘要
Inspection and condition assessment of pipelines play a vital role in the successful operation and maintenance of systems. In the United States, closed-circuit television (CCTV) is the commonly used device for inspecting the inner environment of sanitary sewer pipes. Inspection of every individual sanitary sewer pipe segment is not feasible for any municipality owing to its large inventory of pipes and incurred cost. Machine learning (ML) algorithms, such as logistic regression (LR), k-nearest neighbors (k-NN), and random forests (RF), were employed to develop condition prediction models that could predict the sanitary sewer pipes in need of repair or a maintenance activity. Although the LR model was unable to capture any sewer pipe in poor condition, the same model has resulted in a reasonably higher area under the curve (AUC) value of 0.76. This phenomenon was found to be due to higher imbalance in the data set. Therefore, the study aimed to overcome the limitation of imbalanced classification by employing techniques such as random under sampling and random over sampling. ML algorithms were employed for all three sampled data sets. With an F1-score of 0.94, the RF model outperformed both LR and k-NN models. The developed models can be utilized by utility owners and municipal asset managers to make more informed decisions on future inspections of sewer pipelines.
更多查看译文
AI 理解论文
溯源树
样例
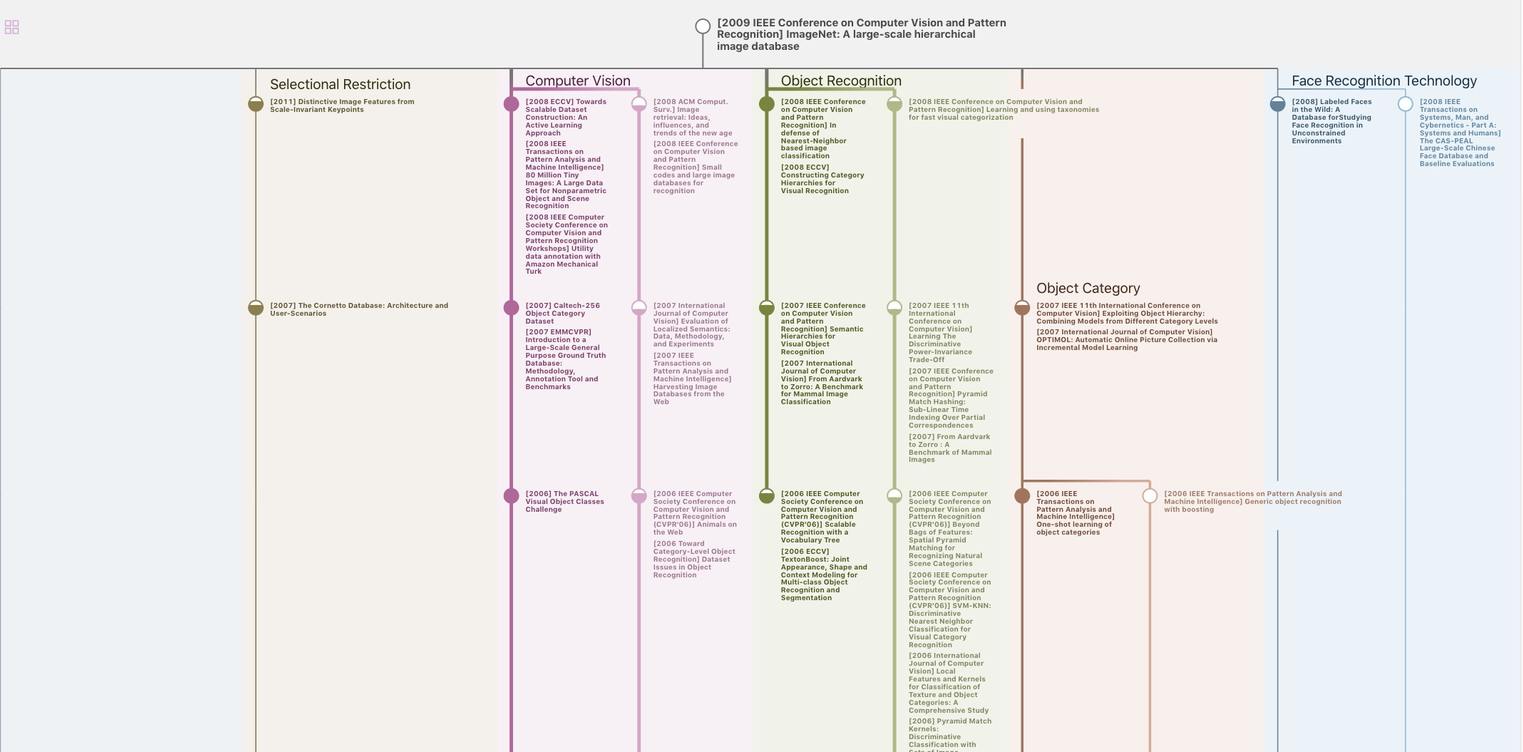
生成溯源树,研究论文发展脉络
Chat Paper
正在生成论文摘要