A Generalized Community-Structure-Aware Optimization Framework for Efficient Subgraph Matching in Social Network Analysis
IEEE TRANSACTIONS ON COMPUTATIONAL SOCIAL SYSTEMS(2023)
摘要
Subgraph matching is a common and important query for analyzing social networks. However, existing subgraph matching algorithms are still unsatisfactory in efficiency. As different subgraph matching algorithms are suitable for different conditions, this article aims to find optimization methods effective for different subgraph matching algorithms. Specifically, the contributions of this article mainly include three aspects. First, this article defines the concept of a community distribution scheme (c-scheme), and proposes an efficient generalized community-structure-aware optimization framework named GCF to accelerate the process of subgraph matching. Specifically, in the GCF framework, subgraph matching is performed by dealing with all c-schemes, and GCF can accelerate different subgraph matching algorithms by exploiting the community structure of the graph data. Second, three strategies are proposed and applied in GCF to further accelerate the process of subgraph matching. In detail, GCF analyzes the structure of the pattern graph and avoids redundant calculation with the strategy of two-level symmetry-breaking (2LS). Besides, GCF builds indexes on the community paths of the data graph to detect useless c-schemes in advance. Moreover, GCF performs community-structure-based boundary pruning and reduces the search space. Third, in the experiments, four state-of-the-art subgraph matching algorithms, i.e., TurboISO, VF3, VF3-Light, and DAF, are optimized with GCF. The results of the experiments conducted on both real-world and synthetic datasets show that the GCF is efficient and can significantly reduce the time consumption of different existing subgraph matching algorithms. Specifically, the optimized subgraph matching algorithms can be more than 2800 $\times$ faster than the original algorithms in the best condition. Moreover, the experimental results on datasets with different scales demonstrate that GCF has considerable scalability.
更多查看译文
关键词
Community structure,optimization,subgraph matching
AI 理解论文
溯源树
样例
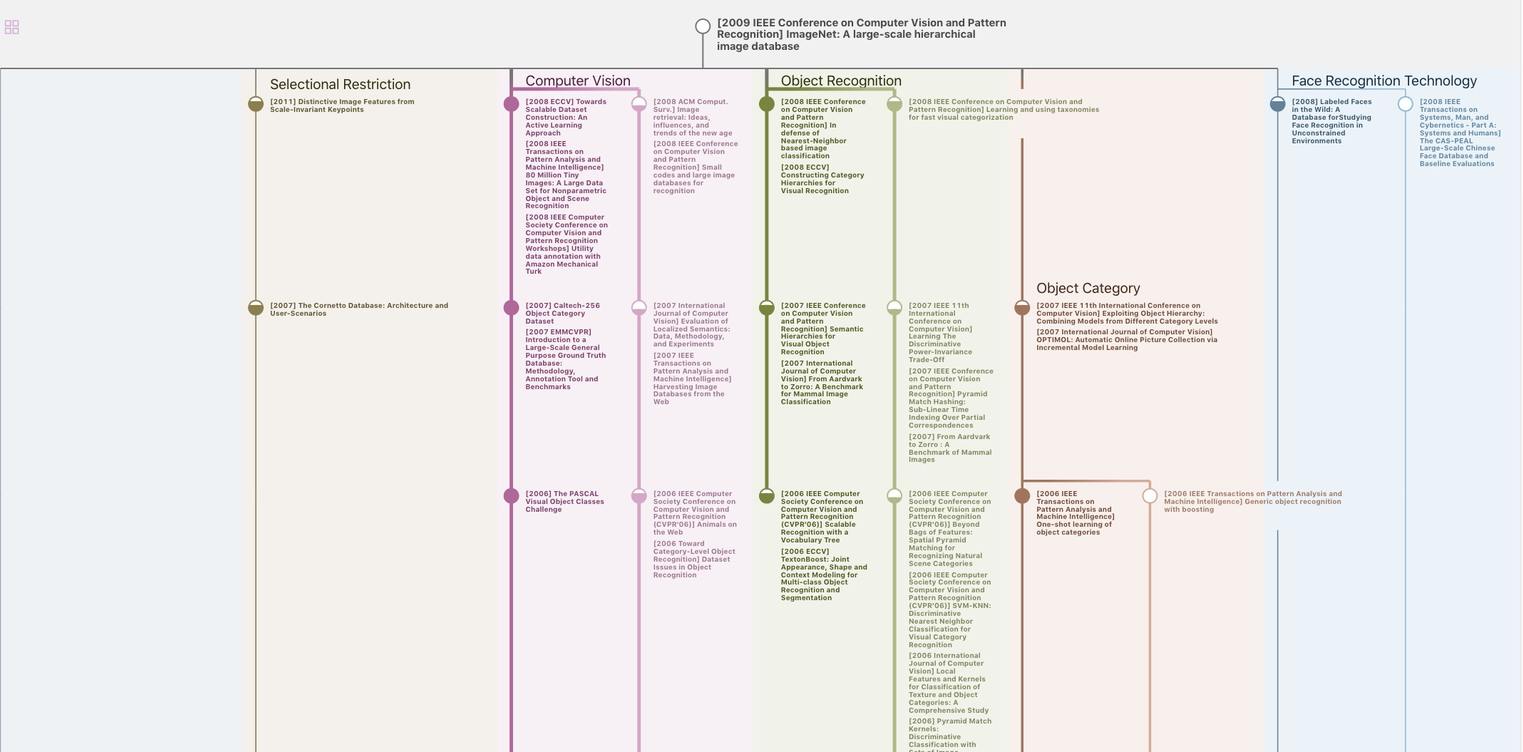
生成溯源树,研究论文发展脉络
Chat Paper
正在生成论文摘要