Prediction of pore-scale clogging using artificial intelligence algorithms
STOCHASTIC ENVIRONMENTAL RESEARCH AND RISK ASSESSMENT(2023)
摘要
We use five established, but conceptually different artificial intelligence algorithms for analysing clogging and quantifying colloid transport at pore scale: artificial neural networks, decision tree, random forest, linear regression, and support vector regression. We test how these algorithm can predict clogging by interpolating physics based simulation data. Our training and test data set is based on results from Lattice Boltzmann simulations reproducing the physics of colloid transport through a typical pore throat present in glass beads or medium sized sand. We perform hyperparameter optimization through cross validation for all algorithms. The tree based methods have the highest Nash–Sutcliffe efficiencies among all tested algorithms with values mostly above 0.9 for the independent test data. The event of clogging can be predicted even with 100% accuracy. Our results indicate a non-linear, rather categorial nature of the (simulation) data. This is in contrast to the typical use of neural network algorithms for simulation data while tree based methods are often applied to observational data. We partly link this to the small size of our dataset. Our application of artificial intelligence in porous media research shows that time-consuming Lattice Boltzmann simulations can be easily supplemented and extended at small computational costs while predictability of clogging and quantitative effects of process specific parameters on colloidal transport are given with high reliability.
更多查看译文
关键词
Clogging, Porous media, Artificial intelligence algorithms, Pore scale, Colloid transport
AI 理解论文
溯源树
样例
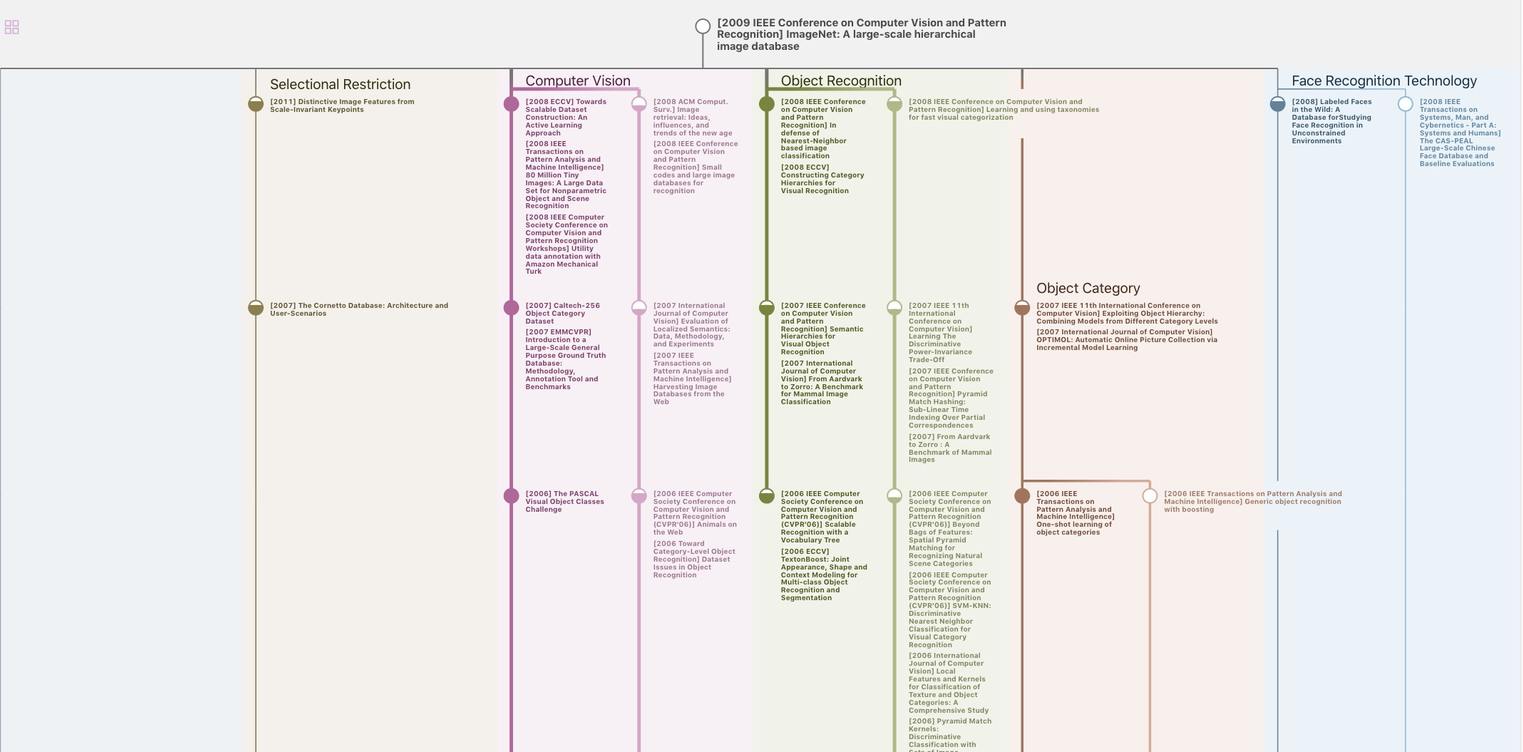
生成溯源树,研究论文发展脉络
Chat Paper
正在生成论文摘要