Finding Nash equilibrium based on reinforcement learning for bidding strategy and distributed algorithm for ISO in imperfect electricity market
APPLIED ENERGY(2023)
摘要
Finding Nash equilibrium in an imperfect market with active and strategic resources is key to the successful operation of the electricity market. A hierarchical Nash distributed reinforcement learning (HNDRL) framework is proposed to realize the interaction between the market participants and independent system operator (ISO). In the bidding stage, multi-agent Nash policy reinforcement learning is utilized to update the bidding strategies for market participants, i.e., generation company (GenCo) and distributed company (DisCo), which maximizes their own profit with imperfect information. The bidding action is updated by the probability iteration to deal with the continuous bidding strategy. And in the market clearing stage, the distributed dynamic-average consensus optimization algorithm is proposed at the ISO level to obtain the locational marginal price (LMP) and transaction quantities. With the participation of the demand-side in the electricity market, the proposed HNDRL framework can reach a Nash equilibrium with the optimal bidding strategy and handle global resource constraints in a distributed way. In addition, theoretical analysis is provided to ensure that the participants' strategy exponentially converges to the Nash equilibrium for both convex and non-convex problems. Finally, the IEEE 30-bus system is employed to illustrate the efficiency. Compared with the existing method, the simulation results show that the proposed HNDRL framework has a faster convergence rate with the range of 2.667-3.333 times and can obtain higher profit with the range of 1.4286%-7.1429% at the Nash equilibrium point.
更多查看译文
关键词
Bidding strategy,Imperfect electricity market,Nash equilibrium,Distributed optimization,Reinforcement learning,Multi-agent
AI 理解论文
溯源树
样例
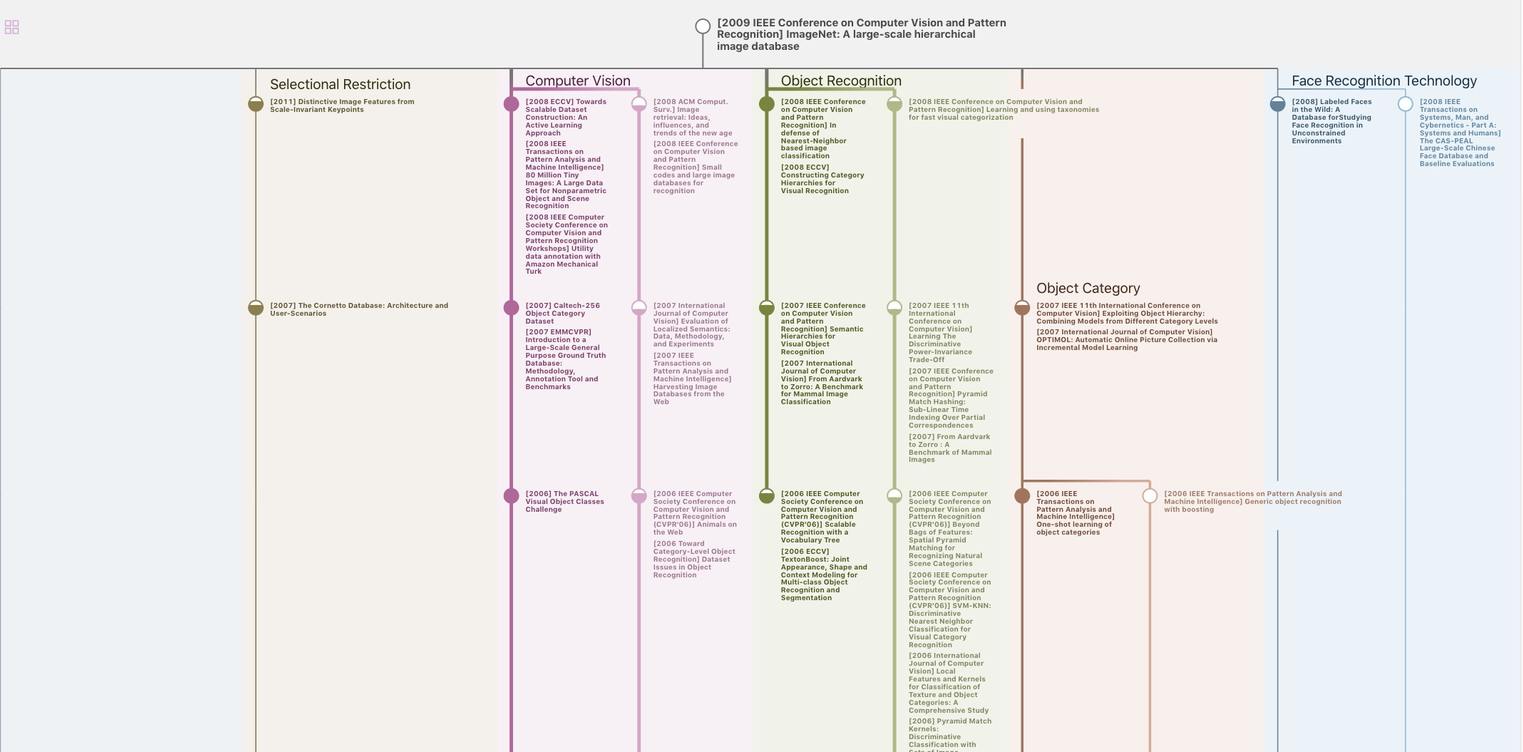
生成溯源树,研究论文发展脉络
Chat Paper
正在生成论文摘要