AUV planning and calibration method considering concealment in uncertain environments
FRONTIERS IN MARINE SCIENCE(2023)
摘要
Introduction Autonomous underwater vehicles (AUVs) are required to thoroughly scan designated areas during underwater missions. They typically follow a zig-zag trajectory to achieve full coverage. However, effective coverage can be challenging in complex environments due to the accumulation and drift of navigation errors. Possible solutions include surfacing for satellite positioning or underwater acoustic positioning using transponders on other vehicles. Nevertheless, surfacing or active acoustics can compromise stealth during reconnaissance missions in hostile areas by revealing the vehicle's location.Methods We propose calibration and planning strategies based on error models and acoustic positioning to address this challenge. Acoustic markers are deployed via surface ships to minimize navigation errors while maintaining stealth. And a new path planning method using a traceless Kalman filter and acoustic localization is proposed to achieve full-area coverage of AUVs. By analyzing the statistics of accumulated sensor errors, we optimize the positions of acoustic markers to communicate with AUVs and achieve better coverage. AUV trajectory concealment is achieved during detection by randomizing the USV navigation trajectory and irregularizing the locations of acoustic marker.Results The proposed method enables the cumulative determination of the absolute position of a target with low localization error in a side-scan sonar-based search task. Simulations based on large-scale maps demonstrate the effectiveness and robustness of the proposed algorithm.Discussion Solving the problem of accumulating underwater localization errors based on inertial navigation by error modeling and acoustic calibration is a typical way. In this paper, we have implemented a method to solve the localization error in a search scenario where stealth is considered.
更多查看译文
关键词
cooperative navigation, acoustic communication, CCPP, cumulative error, coverage ratio, concealment, UKF
AI 理解论文
溯源树
样例
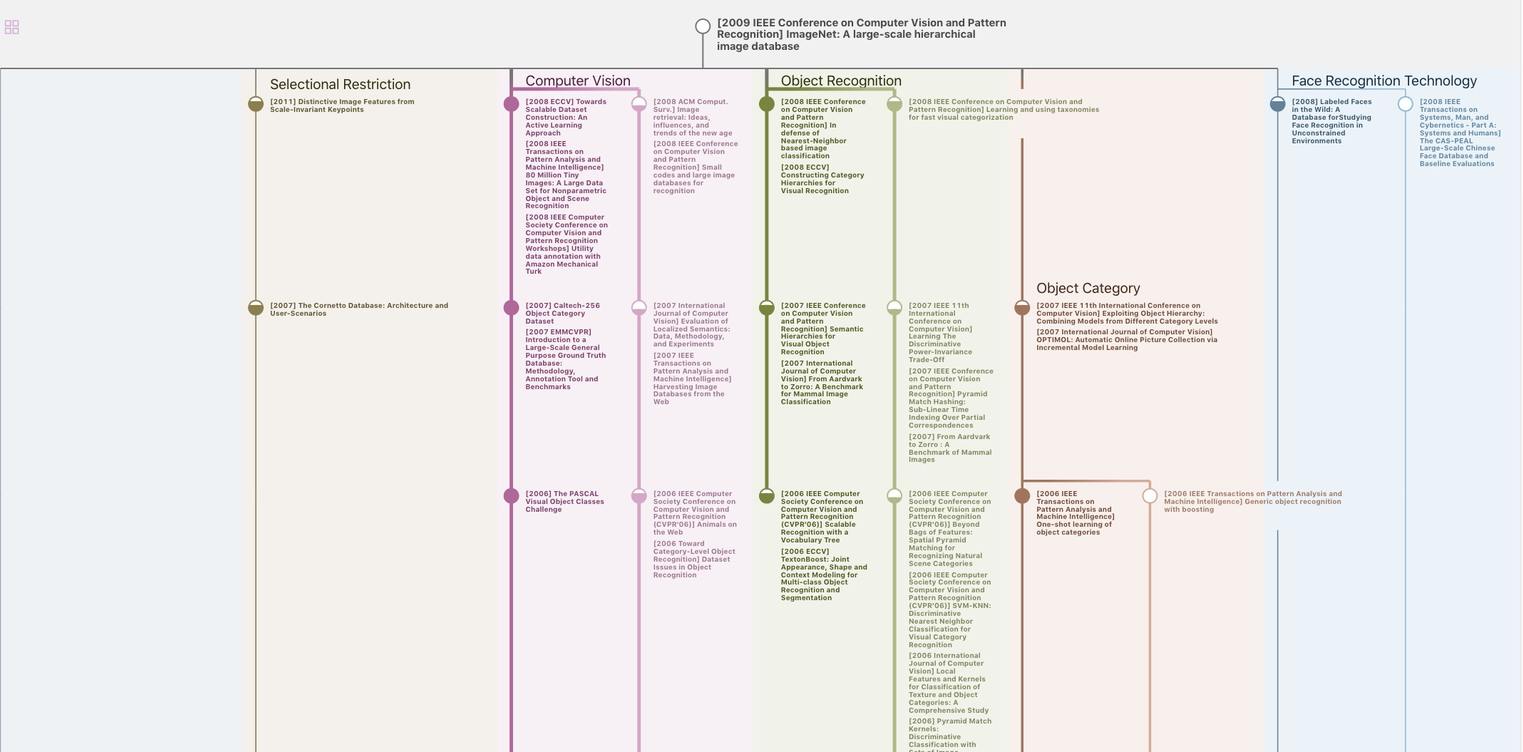
生成溯源树,研究论文发展脉络
Chat Paper
正在生成论文摘要