Reinforcement learning-based design of shape-changing metamaterials
JOURNAL OF MATERIALS CHEMISTRY A(2023)
摘要
During the last decade, artificially architected materials have been designed to obtain properties unreachable by naturally occurring materials, whose properties are determined by their atomic structure and chemical composition. In this work, we implement a new reinforcement learning (RL) method able to rationally design unique metamaterial structures at the nano-, micro-, and macroscale, which change shape during operational conditions. As an example, we apply this method to design nanostructured silicon anodes for Li-ion batteries (LIBs). The RL model is designed to apply different actions and predict change during operational conditions. The multi-component reward function comprises an increase in the total storage capacity of the resulting battery electrode and structural parameters, such as the minimum distance between the individual components of the nanostructure. Upon experimental validation using a polymer-based 3D printing technique, we expect that the newly discovered structures improve the current Si-based LIB anodes state-of-the-art by almost three times and almost ten times the current commercial LIB based on a graphitic anode. This RL-based optimization method opens up vast design space for other responsive metamaterials with tailored properties and pre-programmed structural transformation. We have implemented a new reinforcement learning method able to rationally design unique metamaterial structures, which change shape during operational conditions. We have applied this to design nanostructured silicon anodes for Li-ion batteries.
更多查看译文
关键词
metamaterials,reinforcement,learning-based,shape-changing
AI 理解论文
溯源树
样例
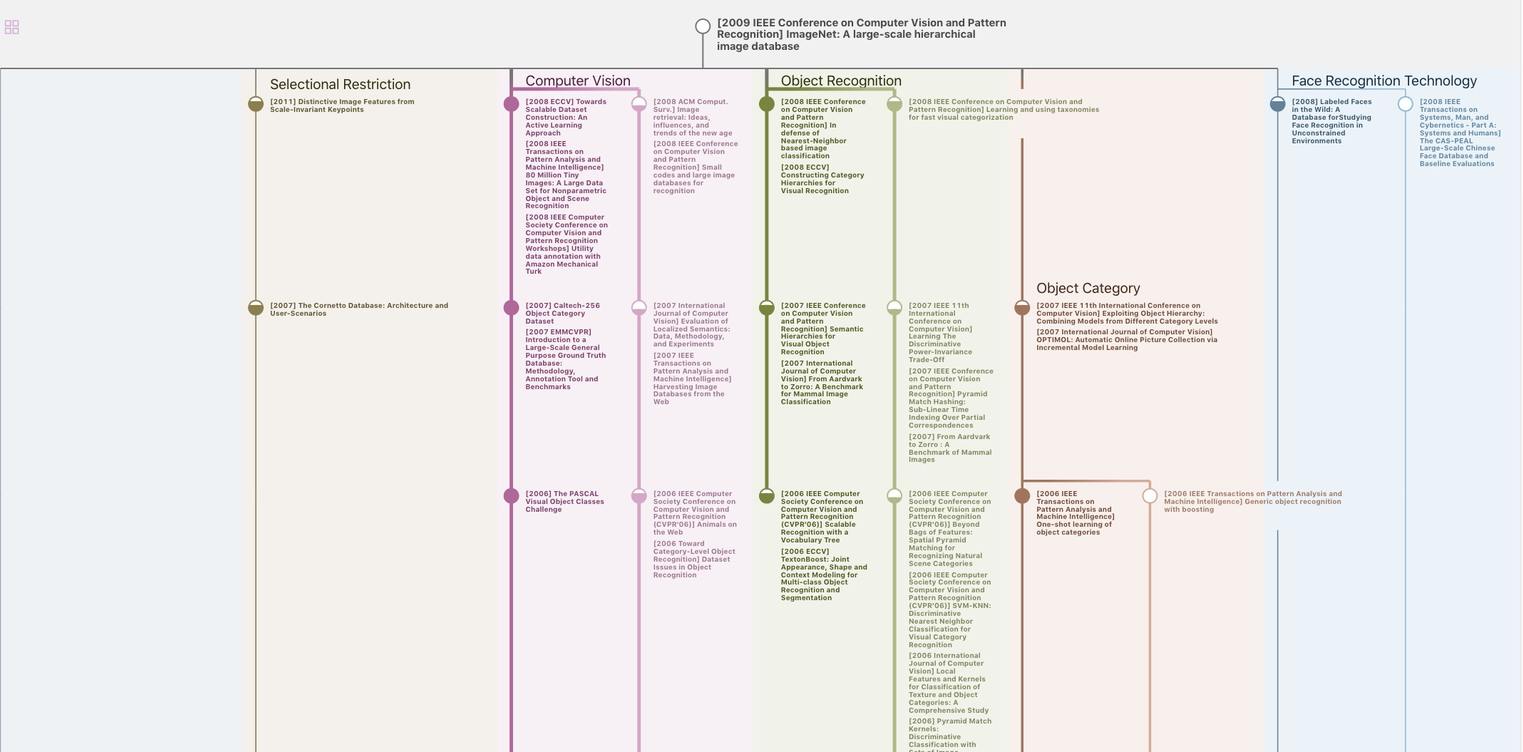
生成溯源树,研究论文发展脉络
Chat Paper
正在生成论文摘要